DcsNet: a real-time deep network for crack segmentation
Signal, Image and Video Processing(2022)
摘要
Detecting cracks are a great significance for the maintenance of the man-made buildings, and deep learning methods such as semantic segmentation have greatly boosted this process in recent years. However, the existing crack segmentation methods often sacrifice feature resolution to achieve real-time inference speed which leads to poor performance, or use complex network module to improve the accuracy which leads to lower inference speed. In this paper, we propose a novel Deep Crack Segmentation Network (DcsNet) that incorporates two feature extraction branches to achieve the balance of speed and accuracy. We first design a morphology branch (MB) to preserve the morphology information of scale invariance that consists of a lightweight convolution network, a pyramid pooling module (PPM), and an attention module (CSA). Meanwhile, a shallow detail branch (DB) with a small stride is constructed to supplement detailed information. Extensive experiments are conducted on five challenging datasets (Crack500, Deepcrack, Gaps384, Structure, and Damcrack), and the results demonstrated that the proposed network achieves a good trade-off between accuracy and inference speed and outperforms state-of-the-art methods.
更多查看译文
关键词
Crack segmentation,Real-time,Morphology information,Detailed information
AI 理解论文
溯源树
样例
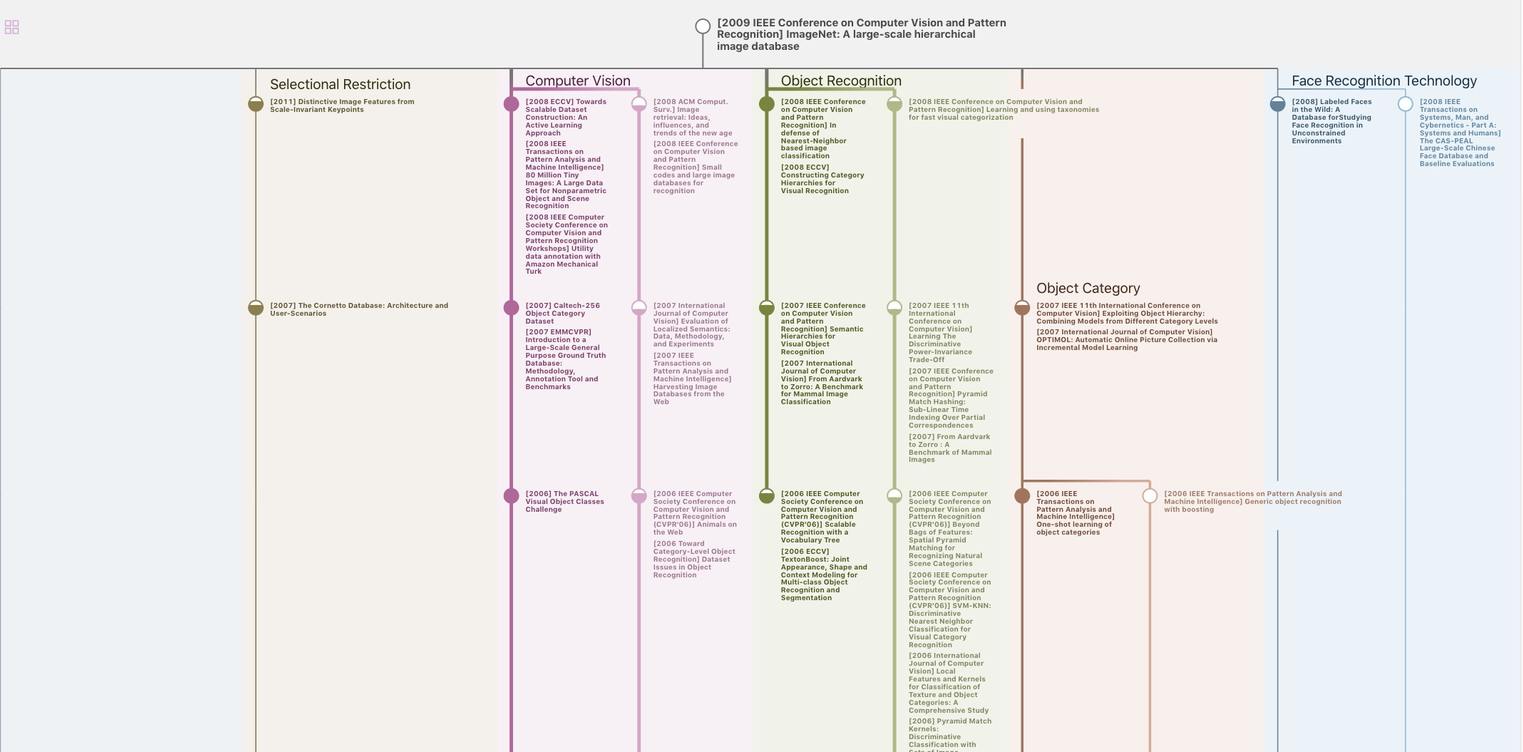
生成溯源树,研究论文发展脉络
Chat Paper
正在生成论文摘要