Rapid 3D Multiparametric Mapping of Brain Metastases with Deep Learning-Based Phase-Sensitive MR Fingerprinting
arxiv(2022)
摘要
In MR fingerprinting (MRF) reconstruction, measured data is pattern-matched to simulated signals to extract quantitative tissue parameters. A critical drawback to this approach is the exponentially increasing compute time for mapping of multiple parameters. Previously, a deep learning (DL) reconstruction method called DRONE was shown to overcome this constraint by mapping the magnitude time-series signal to the underlying tissue parameters. However, relaxometry from magnitude images is susceptible to errors arising from ambiguities in the zero crossing of the signal or the non-zero noise mean. The aim of this study is to develop rapid acquisition and quantification methods to enable accurate multiparametric tissue mapping from complex data. An optimized EPI based MRF sequence is developed along with a novel phasesensitive DL quantification allowing the use of real-valued neural networks to reconstruct complex measured data and providing an additional quantitative map of the phase. Phantom experiments demonstrate the accuracy of the proposed approach. A comparison to previous DRONE methods in a healthy subject shows improved fidelity to known T1 and T2 values for the phase-sensitive approach. By processing the estimated phase map with conventional quantitative susceptibility mapping algorithms, we demonstrate the feasibility of simultaneous quantification of proton density, T1, T2, transmitter B1+ field and the quantitative susceptibility maps. In vivo experiments in a healthy volunteer and a subject with metastatic brain cancer are used to illustrate potential applications of this technology for treatment response assessment and tumor characterization.
更多查看译文
AI 理解论文
溯源树
样例
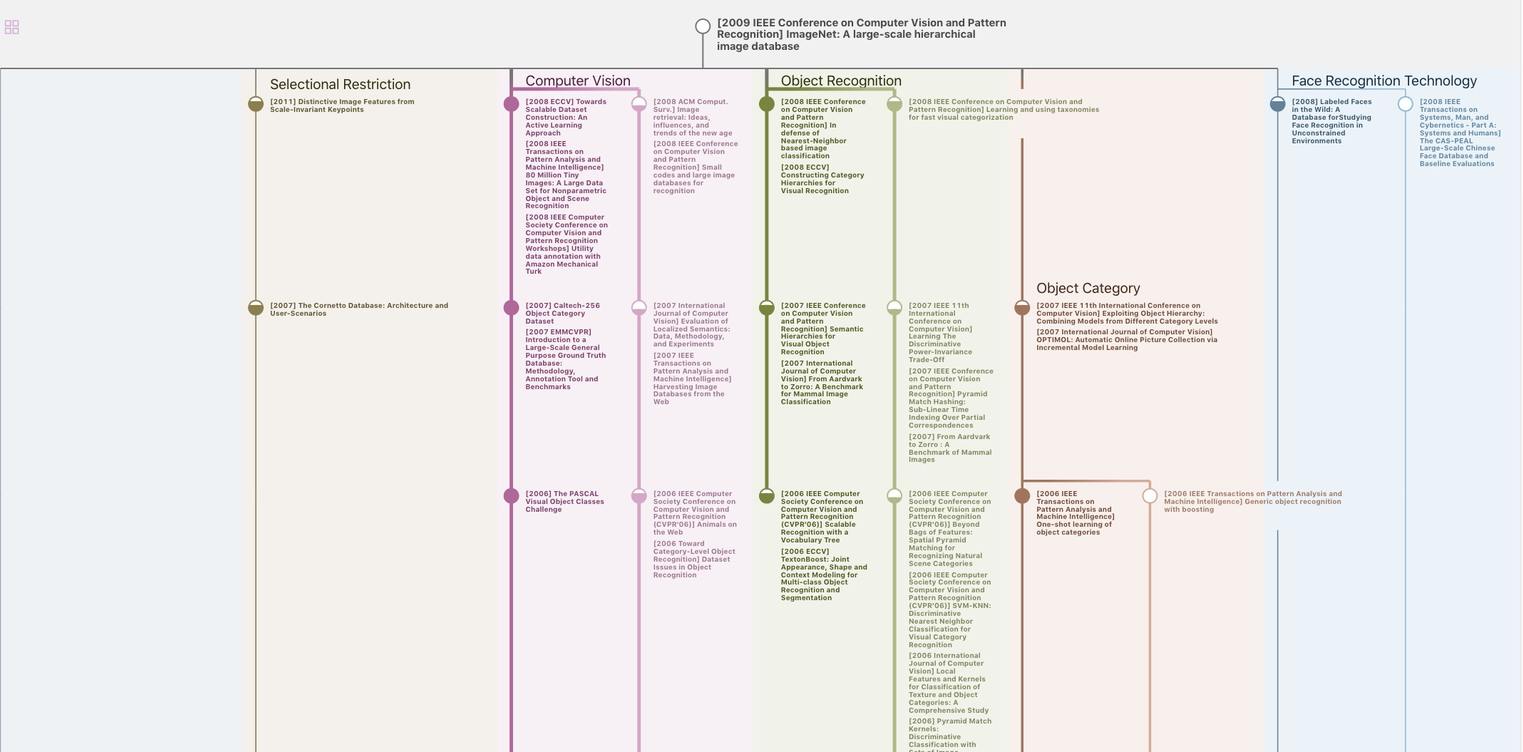
生成溯源树,研究论文发展脉络
Chat Paper
正在生成论文摘要