Improving Rare Word Recognition with LM-aware MWER Training
Conference of the International Speech Communication Association (INTERSPEECH)(2022)
摘要
Language models (LMs) significantly improve the recognition accuracy of end-to-end (E2E) models on words rarely seen during training, when used in either the shallow fusion or the rescoring setups. In this work, we introduce LMs in the learning of hybrid autoregressive transducer (HAT) models in the discriminative training framework, to mitigate the training versus inference gap regarding the use of LMs. For the shallow fusion setup, we use LMs during both hypotheses generation and loss computation, and the LM-aware MWER-trained model achieves 10\% relative improvement over the model trained with standard MWER on voice search test sets containing rare words. For the rescoring setup, we learn a small neural module to generate per-token fusion weights in a data-dependent manner. This model achieves the same rescoring WER as regular MWER-trained model, but without the need for sweeping fusion weights.
更多查看译文
关键词
rare word recognition,word recognition,lm-aware
AI 理解论文
溯源树
样例
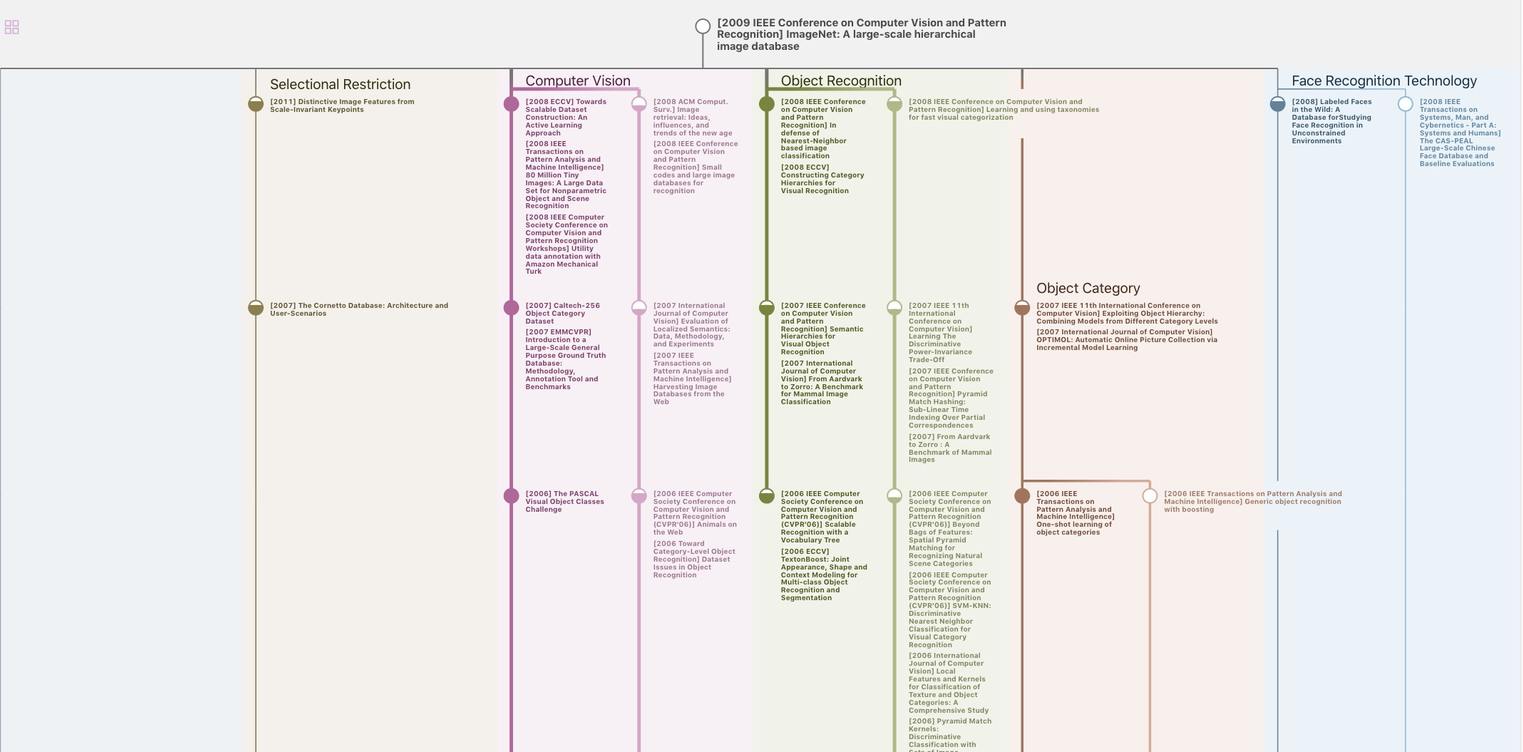
生成溯源树,研究论文发展脉络
Chat Paper
正在生成论文摘要