Multi-modal interactive attention and dual progressive decoding network for RGB-D/T salient object detection
Neurocomputing(2022)
摘要
RGB-based salient object detection (SOD) algorithms have shown good ability to segment salient objects from images, but the performance is still unsatisfactory when dealing with challenging scenes such as ambiguous object contours, low color contrasts between foreground and background. To overcome this problem, RGB-D or RGB-T SOD has been studied. However, they are currently usually treated as separate visual tasks. And most of them directly extract and fuse raw features from backbones. In this paper, we explore the potential commonalities between the two tasks and propose a novel end-to-end unified framework that can be used for both RGB-D and RGB-T SOD. The framework consists of three key components: multi-modal interactive attention (MIA) unit, joint attention guided cross-modal decoding (JAGCD) module, and multi-level feature progressive decoding (MFPD) module. Specifically, MIA units effectively capture rich multi-layered context features from each modality feature, which serve as a bridge between feature encoding and cross-modal decoding. Moreover, the proposed JAGCD and MFPD modules progressively integrate complementary features from multi-source features and different level of fusion features, respectively. To demonstrate the effectiveness of the proposed approach, we conduct comprehensive experiments not only on RGB-D but also on RGB-T saliency detection benchmark. Experimental results show that our approach outperforms other state-of-the-art methods and has good generalization. Moreover, the proposed framework can provide a potential solution for cross-modal complementary tasks. The code will be available at https://github.com/Liangyh18/MIA_DPD.
更多查看译文
关键词
Salient object detection,RGB-D/T image,Cross-modal fusion,Multi-modal interactive attention,Dual progressive decoding
AI 理解论文
溯源树
样例
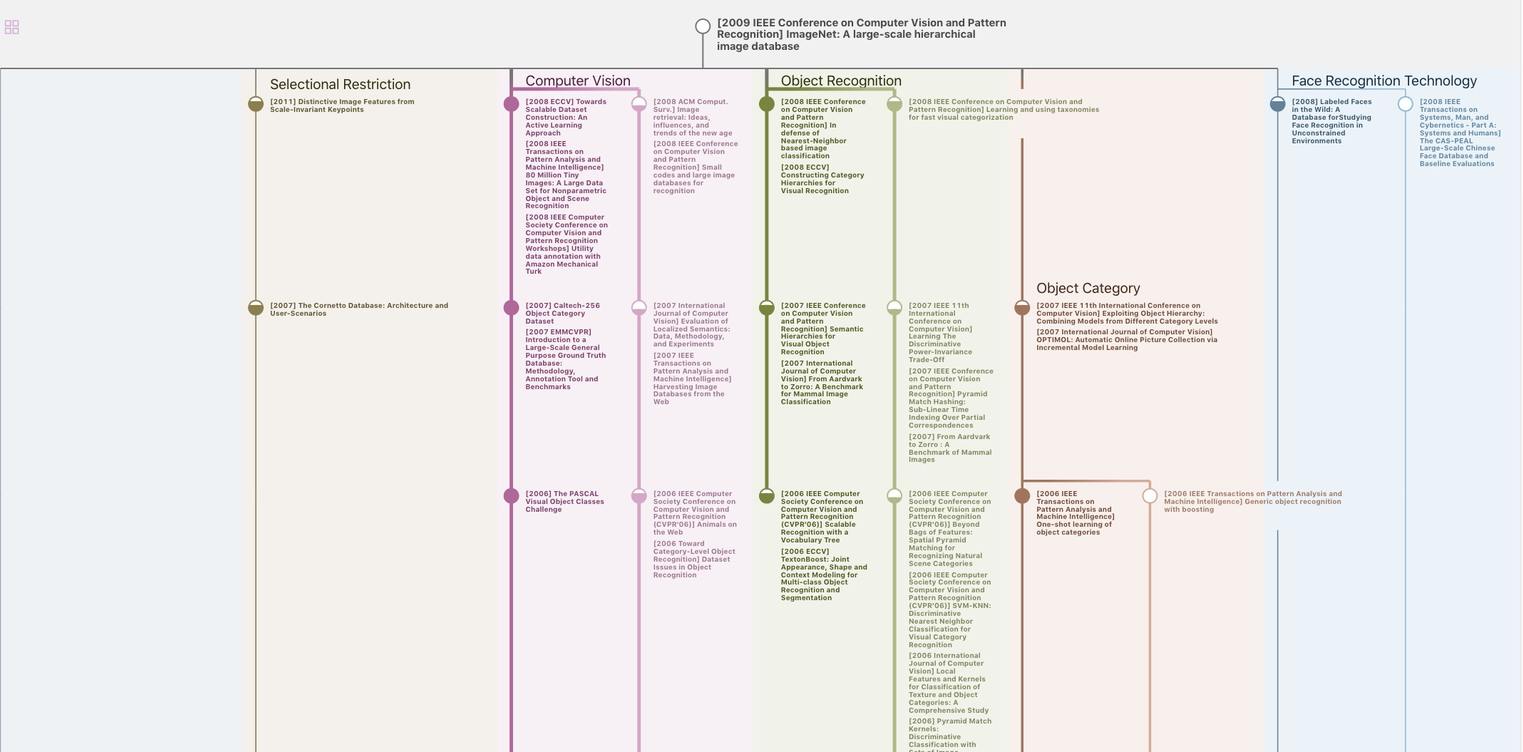
生成溯源树,研究论文发展脉络
Chat Paper
正在生成论文摘要