DRFLM: Distributionally Robust Federated Learning with Inter-client Noise via Local Mixup
arxiv(2022)
摘要
Recently, federated learning has emerged as a promising approach for training a global model using data from multiple organizations without leaking their raw data. Nevertheless, directly applying federated learning to real-world tasks faces two challenges: (1) heterogeneity in the data among different organizations; and (2) data noises inside individual organizations. In this paper, we propose a general framework to solve the above two challenges simultaneously. Specifically, we propose using distributionally robust optimization to mitigate the negative effects caused by data heterogeneity paradigm to sample clients based on a learnable distribution at each iteration. Additionally, we observe that this optimization paradigm is easily affected by data noises inside local clients, which has a significant performance degradation in terms of global model prediction accuracy. To solve this problem, we propose to incorporate mixup techniques into the local training process of federated learning. We further provide comprehensive theoretical analysis including robustness analysis, convergence analysis, and generalization ability. Furthermore, we conduct empirical studies across different drug discovery tasks, such as ADMET property prediction and drug-target affinity prediction.
更多查看译文
关键词
distributionally robust federated learning,local mixup,drflm,noise,inter-client
AI 理解论文
溯源树
样例
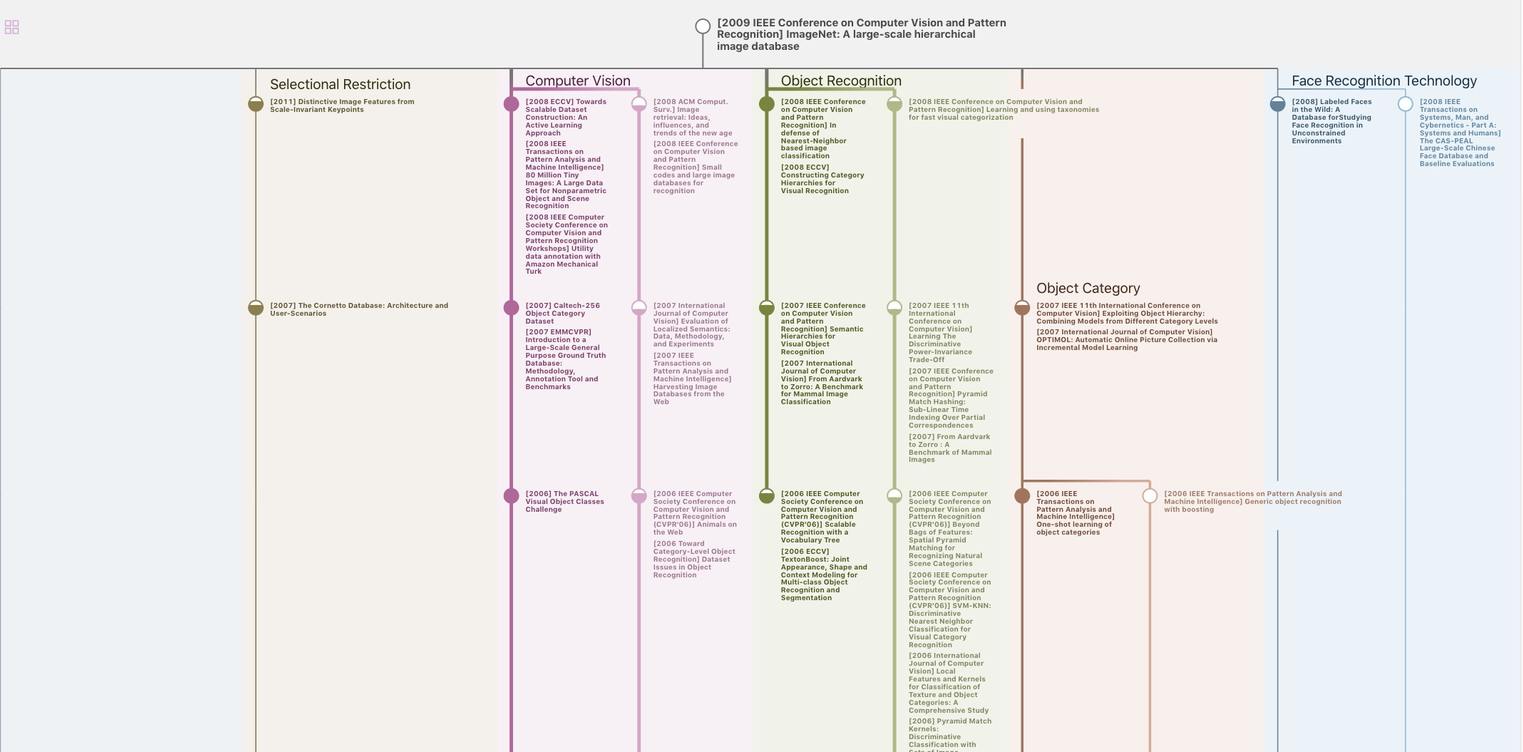
生成溯源树,研究论文发展脉络
Chat Paper
正在生成论文摘要