Towards Comprehensive Testing on the Robustness of Cooperative Multi-agent Reinforcement Learning
2022 IEEE/CVF Conference on Computer Vision and Pattern Recognition Workshops (CVPRW)(2022)
摘要
While deep neural networks (DNNs) have strengthened the performance of cooperative multi-agent reinforcement learning (c-MARL), the agent policy can be easily perturbed by adversarial examples. Considering the safety critical applications of c-MARL, such as traffic management, power management and unmanned aerial vehicle control, it is crucial to test the robustness of c-MARL algorithm before it was deployed in reality. Existing adversarial attacks for MARL could be used for testing, but is limited to one robustness aspects (e.g., reward, state, action), while c-MARL model could be attacked from any aspect. To overcome the challenge, we propose MARLSafe, the first robustness testing framework for c-MARL algorithms. First, motivated by Markov Decision Process (MDP), MARLSafe consider the robustness of c-MARL algorithms comprehensively from three aspects, namely state robustness, action robustness and reward robustness. Any c-MARL algorithm must simultaneously satisfy these robustness aspects to be considered secure. Second, due to the scarceness of c- MARL attack, we propose c-MARL attacks as robustness testing algorithms from multiple aspects. Experiments on SMAC environment reveals that many state-of-the-art c- MARL algorithms are of low robustness in all aspect, pointing out the urgent need to test and enhance robustness of c-MARL algorithms.
更多查看译文
关键词
robustness testing algorithms,comprehensive testing,cooperative multiagent reinforcement learning,state robustness,action robustness,reward robustness,c-MARL attacks,MARLSafe,Markov decision process,safety critical applications,adversarial attacks,agent policy
AI 理解论文
溯源树
样例
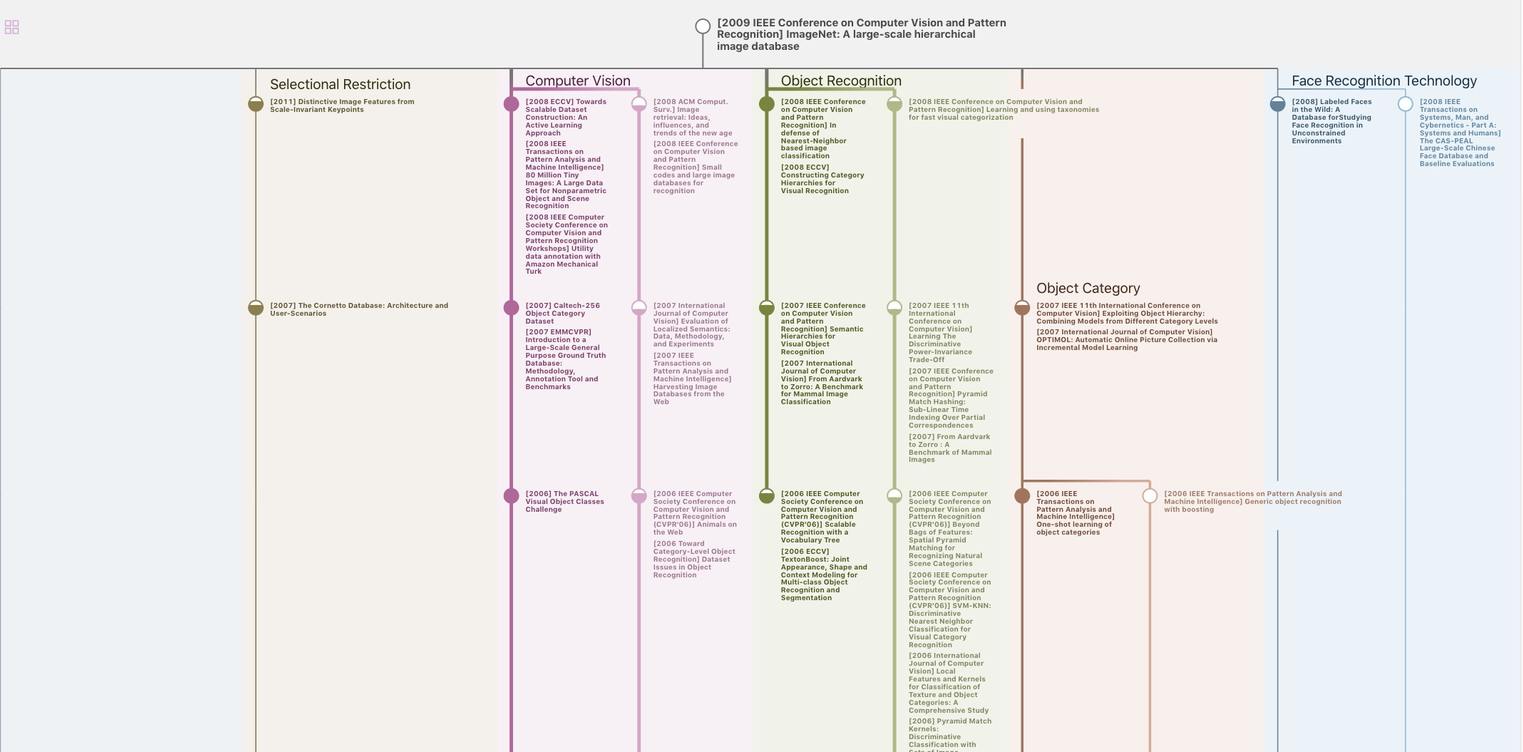
生成溯源树,研究论文发展脉络
Chat Paper
正在生成论文摘要