VDTR: Video Deblurring With Transformer
IEEE Transactions on Circuits and Systems for Video Technology(2023)
摘要
Video deblurring is still an unsolved problem due to the challenging spatio-temporal modeling process. While existing convolutional neural network (CNN)-based methods show a limited capacity of effective spatial and temporal modeling for video deblurring. This paper presents VDTR, an effective Transformer-based model that makes the first attempt to adapt pure Transformer for video deblurring. VDTR exploits the superior long-range and relation modeling capabilities of Transformer for both spatial and temporal modeling. However, it is challenging to design an appropriate Transformer-based model for video deblurring due to the complicated non-uniform blurs, misalignment across multiple frames and the high computational costs for high-resolution spatial modeling. To address these problems, VDTR advocates performing attention within non-overlapping windows and exploiting the hierarchical structure for long-range dependencies modeling. For frame-level spatial modeling, we propose an encoder-decoder Transformer that utilizes multi-scale features for deblurring. For multi-frame temporal modeling, we adapt Transformer to fuse multiple spatial features efficiently. Compared with CNN-based methods, the proposed method achieves highly competitive results on both synthetic and real-world video deblurring benchmarks, including DVD, GOPRO, REDS and BSD. We hope such a Transformer-based architecture can serve as a powerful alternative baseline for video deblurring and other video restoration tasks. The source code will be available at
https://github.com/ljzycmd/VDTR
.
更多查看译文
关键词
Video deblurring,vision transformer,spatio-temporal modeling
AI 理解论文
溯源树
样例
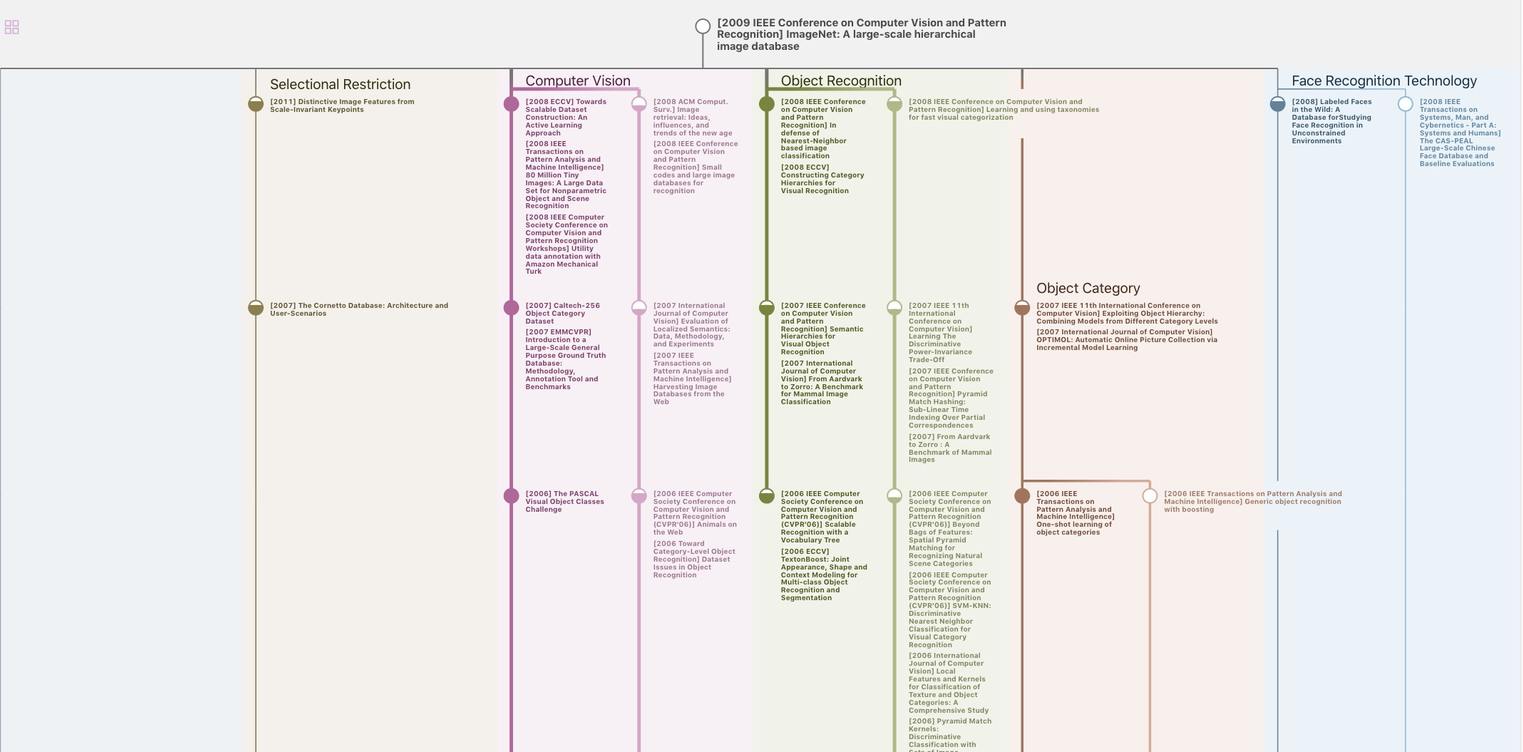
生成溯源树,研究论文发展脉络
Chat Paper
正在生成论文摘要