Federated Learning Cost Disparity for IoT Devices
2022 IEEE INTERNATIONAL CONFERENCE ON COMMUNICATIONS WORKSHOPS (ICC WORKSHOPS)(2022)
摘要
Federated learning (FL) promotes predictive model training at the Internet of things (IoT) devices by evading data collection cost in terms of energy, time, and privacy. We model the learning gain achieved by an IoT device against its participation cost as its utility. Due to the device-heterogeneity, the local model learning cost and its quality, which can be time-varying, differs from device to device. We show that this variation results in utility unfairness because the same global model is shared among the devices. By default, the master is unaware of the local model computation and transmission costs of the devices, thus it is unable to address the utility unfairness problem. Also, a device may exploit this lack of knowledge at the master to intentionally reduce its expenditure and thereby enhance its utility. We propose to control the quality of the global model shared with the devices, in each round, based on their contribution and expenditure. This is achieved by employing differential privacy to curtail global model divulgence based on the learning contribution. In addition, we devise adaptive computation and transmission policies for each device to control its expenditure in order to mitigate utility unfairness. Our results show that the proposed scheme reduces the standard deviation of the energy cost of devices by 99% in comparison to the benchmark scheme, while the standard deviation of the training loss of devices varies around 0.103.
更多查看译文
关键词
federated learning cost disparity,IoT devices,predictive model training,Internet of Things devices,data collection cost,local model learning cost,local model computation,transmission costs,utility unfairness problem,learning contribution,adaptive computation,transmission policies,differential privacy
AI 理解论文
溯源树
样例
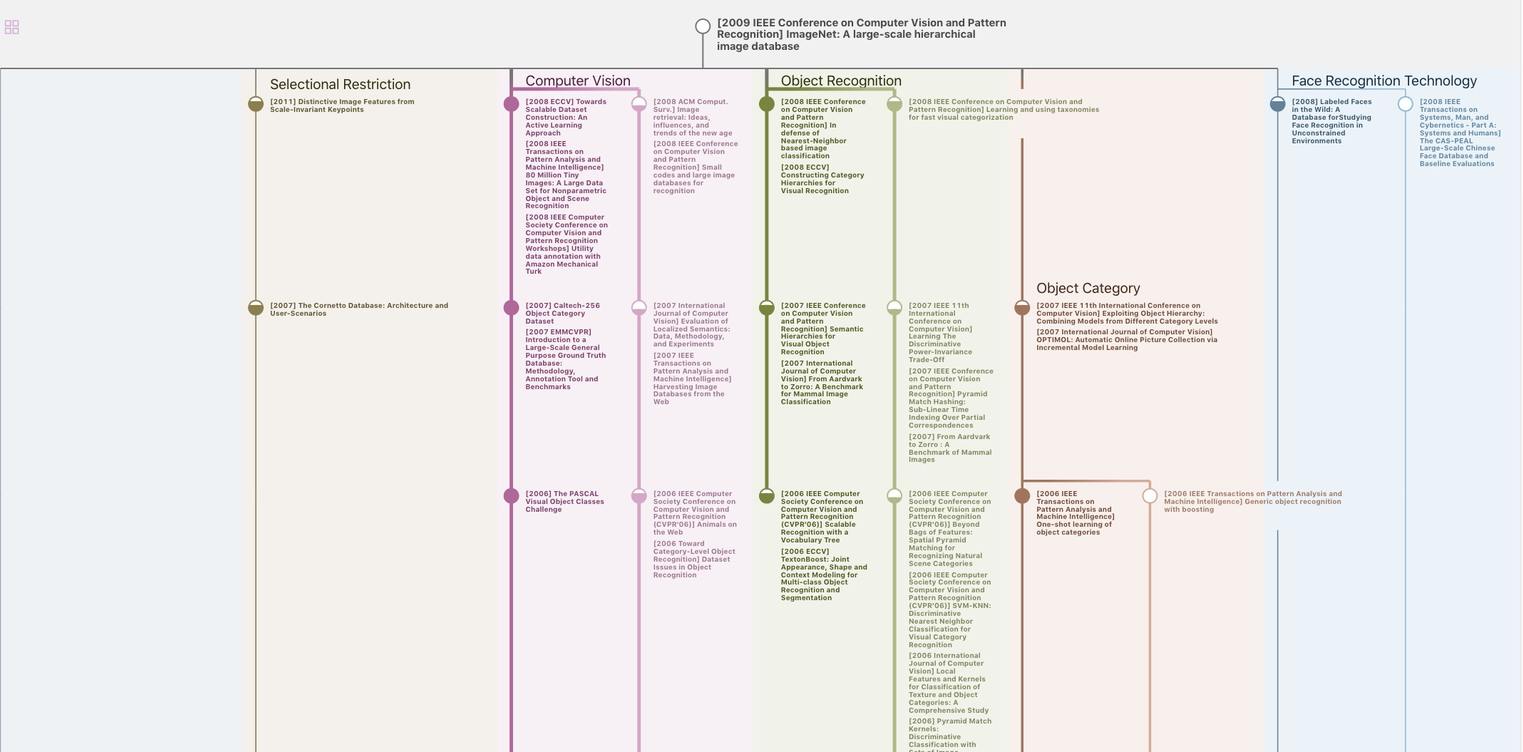
生成溯源树,研究论文发展脉络
Chat Paper
正在生成论文摘要