Unsupervised Contrastive Domain Adaptation for Semantic Segmentation
arxiv(2022)
摘要
Semantic segmentation models struggle to generalize in the presence of domain shift. In this paper, we introduce contrastive learning for feature alignment in cross-domain adaptation. We assemble both in-domain contrastive pairs and cross-domain contrastive pairs to learn discriminative features that align across domains. Based on the resulting well-aligned feature representations we introduce a label expansion approach that is able to discover samples from hard classes during the adaptation process to further boost performance. The proposed approach consistently outperforms state-of-the-art methods for domain adaptation. It achieves 60.2% mIoU on the Cityscapes dataset when training on the synthetic GTA5 dataset together with unlabeled Cityscapes images.
更多查看译文
关键词
contrastive domain adaptation,domain adaptation
AI 理解论文
溯源树
样例
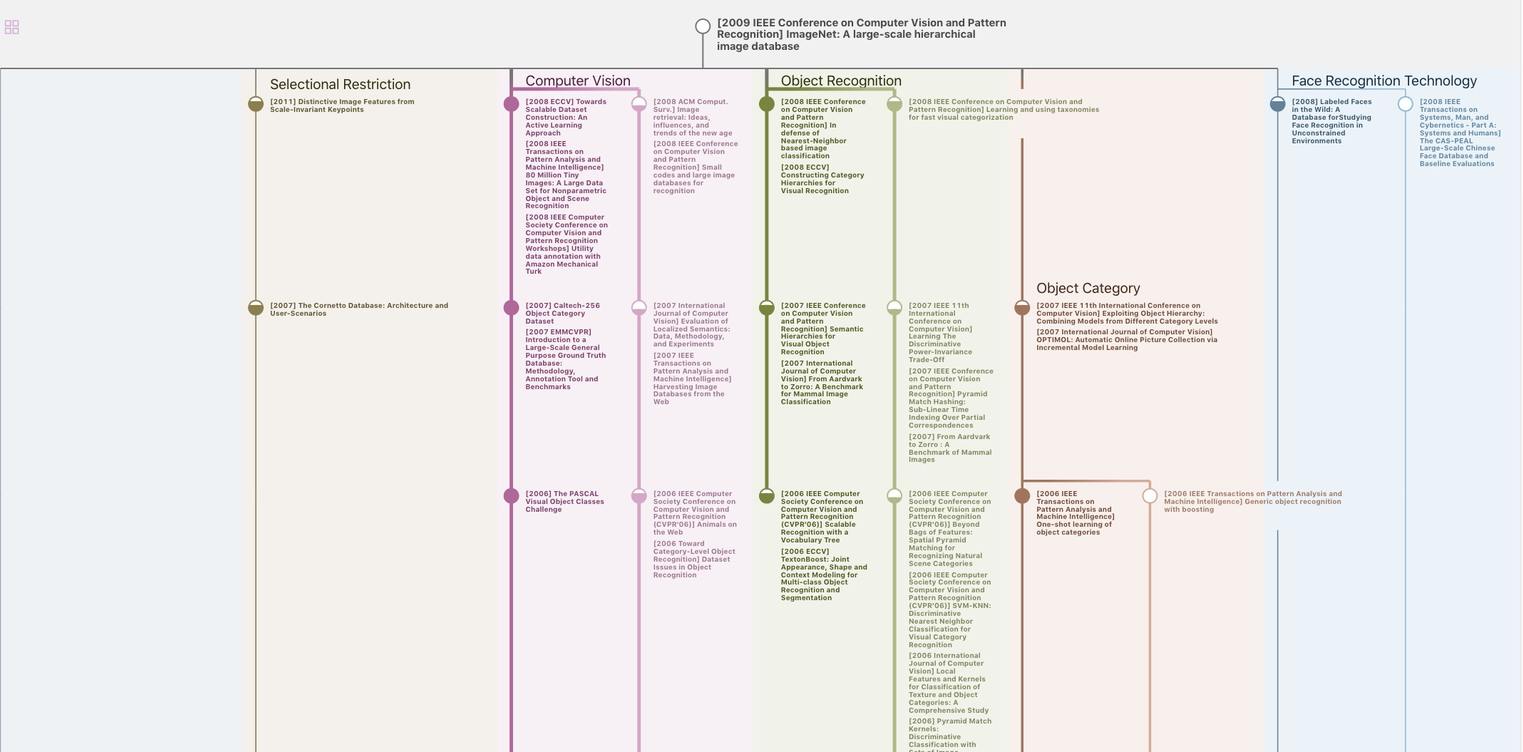
生成溯源树,研究论文发展脉络
Chat Paper
正在生成论文摘要