Imbalanced Classification via a Tabular Translation GAN
arxiv(2022)
摘要
When presented with a binary classification problem where the data exhibits severe class imbalance, most standard predictive methods may fail to accurately model the minority class. We present a model based on Generative Adversarial Networks which uses additional regularization losses to map majority samples to corresponding synthetic minority samples. This translation mechanism encourages the synthesized samples to be close to the class boundary. Furthermore, we explore a selection criterion to retain the most useful of the synthesized samples. Experimental results using several downstream classifiers on a variety of tabular class-imbalanced datasets show that the proposed method improves average precision when compared to alternative re-weighting and oversampling techniques.
更多查看译文
关键词
gan,classification,translation
AI 理解论文
溯源树
样例
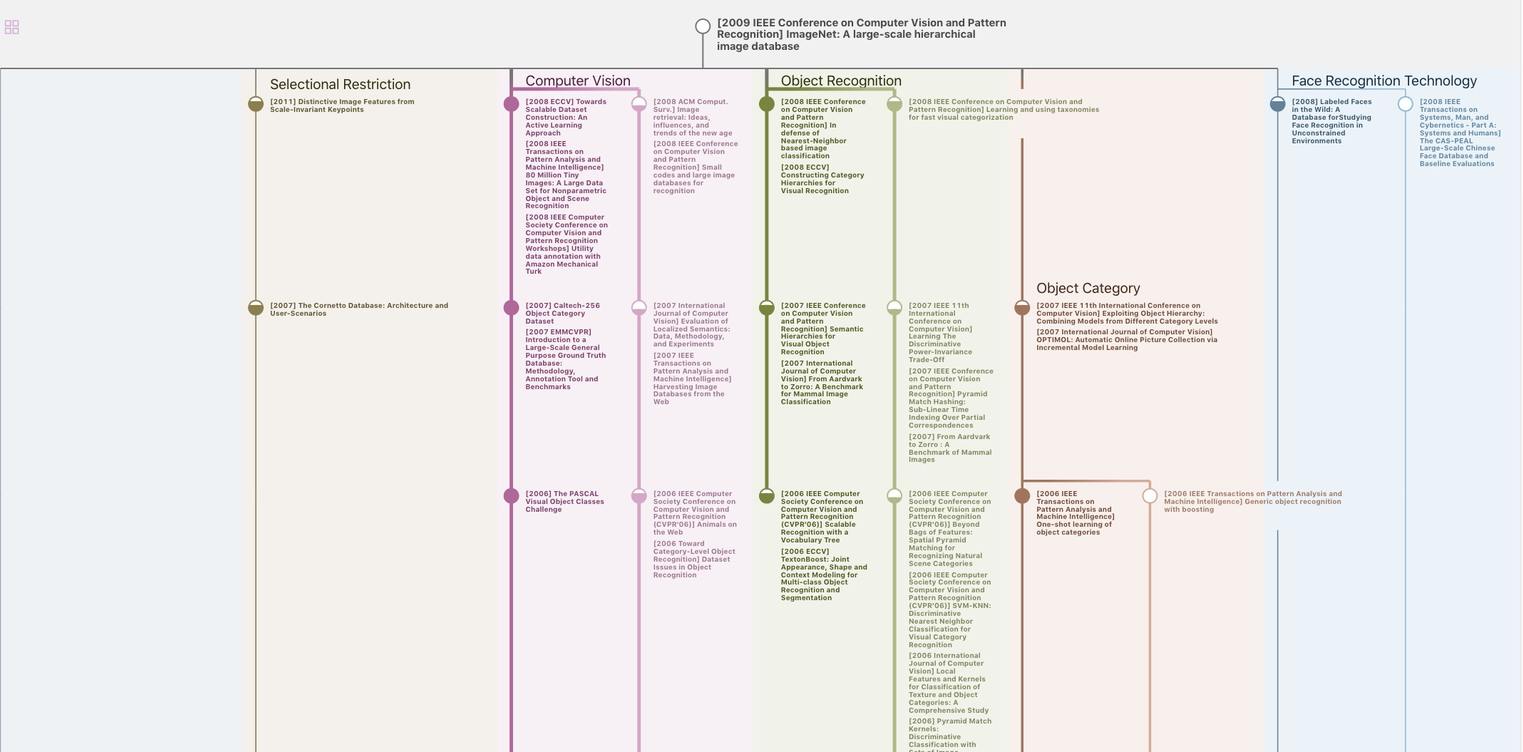
生成溯源树,研究论文发展脉络
Chat Paper
正在生成论文摘要