THORN: Temporal Human-Object Relation Network for Action Recognition
ICPR(2022)
摘要
Most action recognition models treat human activities as unitary events. However, human activities often follow a certain hierarchy. In fact, many human activities are compositional. Also, these actions are mostly human-object interactions. In this paper we propose to recognize human action by leveraging the set of interactions that define an action. In this work, we present an end-to-end network: THORN, that can leverage important human-object and object-object interactions to predict actions. This model is built on top of a 3D backbone network. The key components of our model are: 1) An object representation filter for modeling object. 2) An object relation reasoning module to capture object relations. 3) A classification layer to predict the action labels. To show the robustness of THORN, we evaluate it on EPIC-Kitchen55 and EGTEA Gaze+, two of the largest and most challenging first-person and human-object interaction datasets. THORN achieves state-of-the-art performance on both datasets.
更多查看译文
关键词
3D backbone network,action recognition models,end-to-end network,human action,human activities,human-object interaction datasets,human-object interactions,leverage important human-object,modeling object,most challenging first-person,object relation reasoning module,object relations,object representation filter,object-object interactions,temporal human-object relation network,THORN
AI 理解论文
溯源树
样例
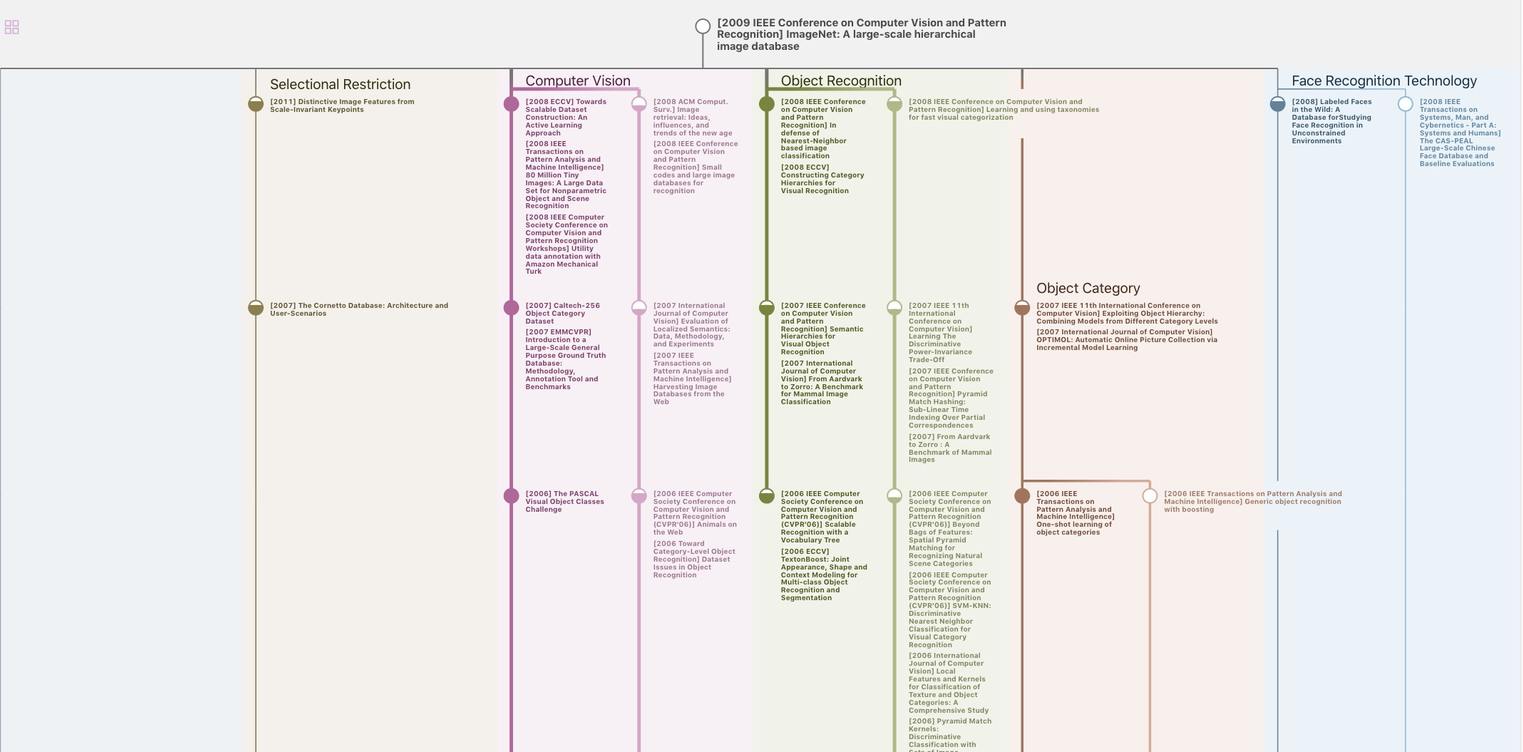
生成溯源树,研究论文发展脉络
Chat Paper
正在生成论文摘要