Higher Moments for Optimal Balance Weighting in Causal Estimation.
Epidemiology(2022)
摘要
We expand upon a simulation study that compared three promising methods for estimating weights for assessing the average treatment effect on the treated for binary treatments: generalized boosted models, covariate-balancing propensity scores, and entropy balance. The original study showed that generalized boosted models can outperform covariate-balancing propensity scores, and entropy balance when there are likely to be nonlinear associations in both the treatment assignment and outcome models and when the other two models are fine-tuned to obtain balance only on first-order moments. We explore the potential benefit of using higher-order moments in the balancing conditions for covariate-balancing propensity scores and entropy balance. Our findings showcase that these two models should, by default, include higher-order moments and focusing only on first moments can result in substantial bias in estimated treatment effect estimates from both models that could be avoided using higher moments.
更多查看译文
关键词
Causal inference,Observational study,Propensity score,Entropy balance,Higher-order moments,Balance
AI 理解论文
溯源树
样例
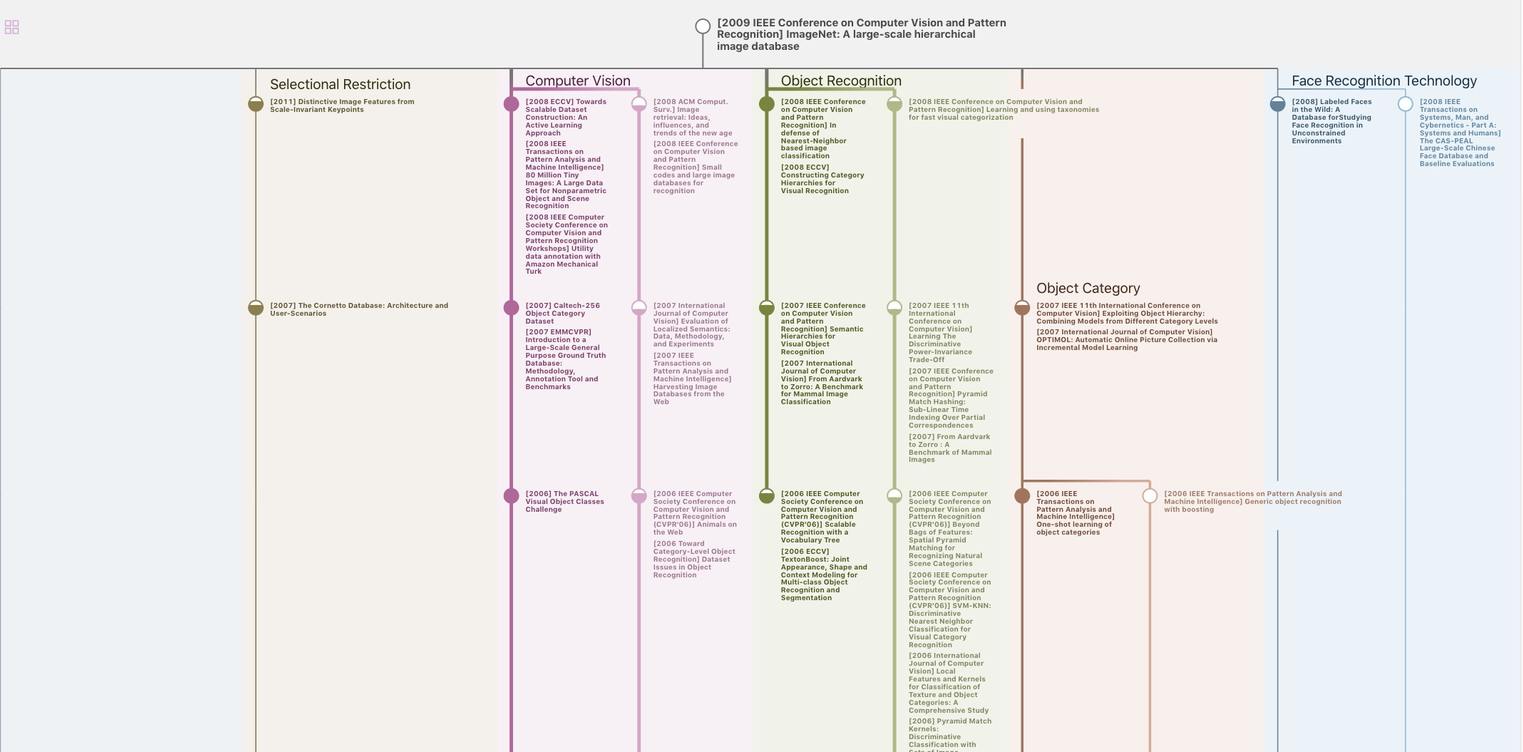
生成溯源树,研究论文发展脉络
Chat Paper
正在生成论文摘要