Application of unsupervised deep learning algorithms for identification of specific clusters of chronic cough patients from EMR data
BMC Bioinformatics(2022)
摘要
Background Chronic cough affects approximately 10% of adults. The lack of ICD codes for chronic cough makes it challenging to apply supervised learning methods to predict the characteristics of chronic cough patients, thereby requiring the identification of chronic cough patients by other mechanisms. We developed a deep clustering algorithm with auto-encoder embedding (DCAE) to identify clusters of chronic cough patients based on data from a large cohort of 264,146 patients from the Electronic Medical Records (EMR) system. We constructed features using the diagnosis within the EMR, then built a clustering-oriented loss function directly on embedded features of the deep autoencoder to jointly perform feature refinement and cluster assignment. Lastly, we performed statistical analysis on the identified clusters to characterize the chronic cough patients compared to the non-chronic cough patients. Results The experimental results show that the DCAE model generated three chronic cough clusters and one non-chronic cough patient cluster. We found various diagnoses, medications, and lab tests highly associated with chronic cough patients by comparing the chronic cough cluster with the non-chronic cough cluster. Comparison of chronic cough clusters demonstrated that certain combinations of medications and diagnoses characterize some chronic cough clusters. Conclusions To the best of our knowledge, this study is the first to test the potential of unsupervised deep learning methods for chronic cough investigation, which also shows a great advantage over existing algorithms for patient data clustering.
更多查看译文
关键词
Chronic cough,Unsupervised learning,Deep clustering,EMR data
AI 理解论文
溯源树
样例
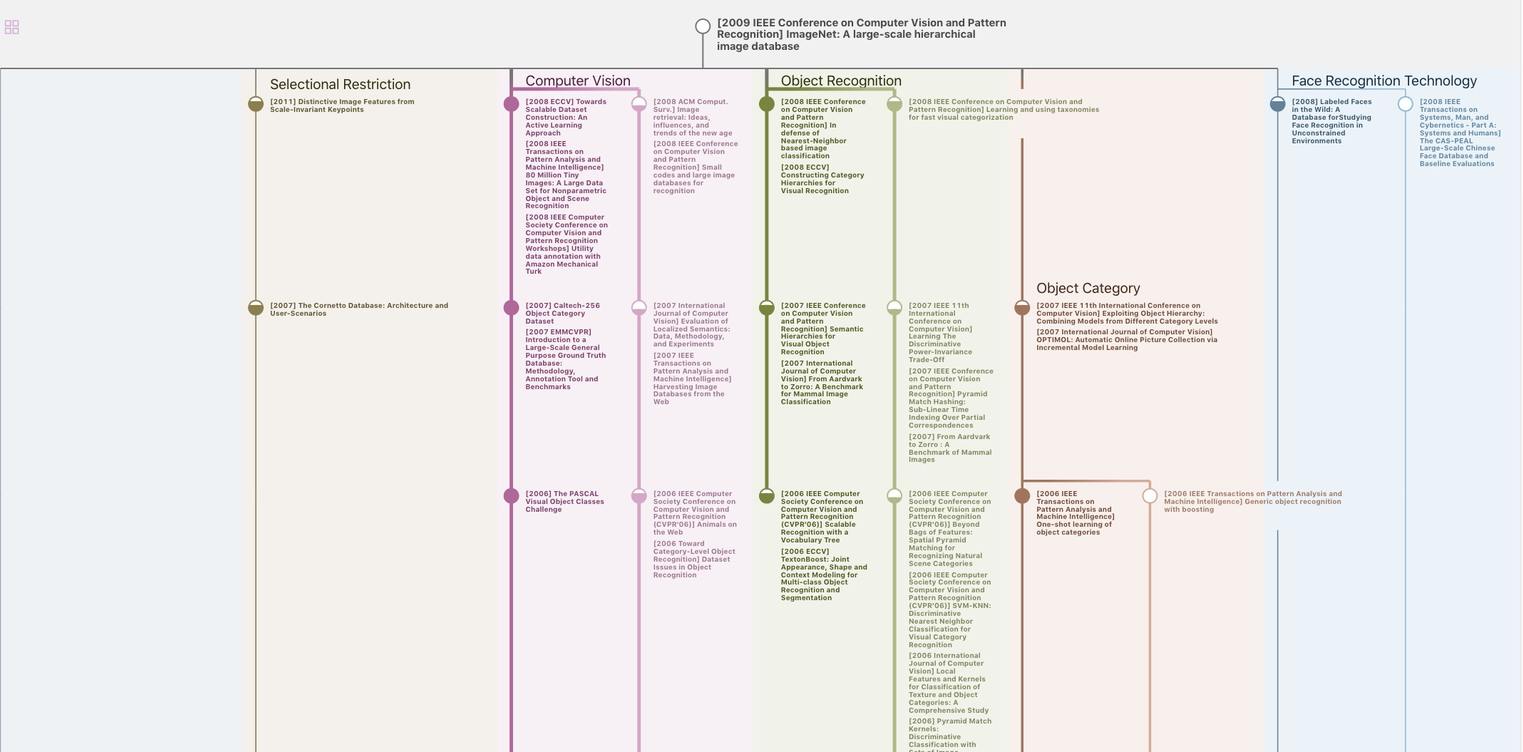
生成溯源树,研究论文发展脉络
Chat Paper
正在生成论文摘要