A Learned Index for Exact Similarity Search in Metric Spaces
arxiv(2022)
摘要
Indexing is an effective way to support efficient query processing in large databases. Recently the concept of learned index, which replaces or complements traditional index structures with machine learning models, has been actively explored to reduce storage and search costs. However, accurate and efficient similarity query processing in high-dimensional metric spaces remains to be an open challenge. In this paper, we propose a novel indexing approach called LIMS that uses data clustering, pivot-based data transformation techniques and learned indexes to support efficient similarity query processing in metric spaces. In LIMS, the underlying data is partitioned into clusters such that each cluster follows a relatively uniform data distribution. Data redistribution is achieved by utilizing a small number of pivots for each cluster. Similar data are mapped into compact regions and the mapped values are totally ordinal. Machine learning models are developed to approximate the position of each data record on disk. Efficient algorithms are designed for processing range queries and nearest neighbor queries based on LIMS, and for index maintenance with dynamic updates. Extensive experiments on real-world and synthetic datasets demonstrate the superiority of LIMS compared with traditional indexes and state-of-the-art learned indexes.
更多查看译文
关键词
Learned index,multi-dimension,metric space
AI 理解论文
溯源树
样例
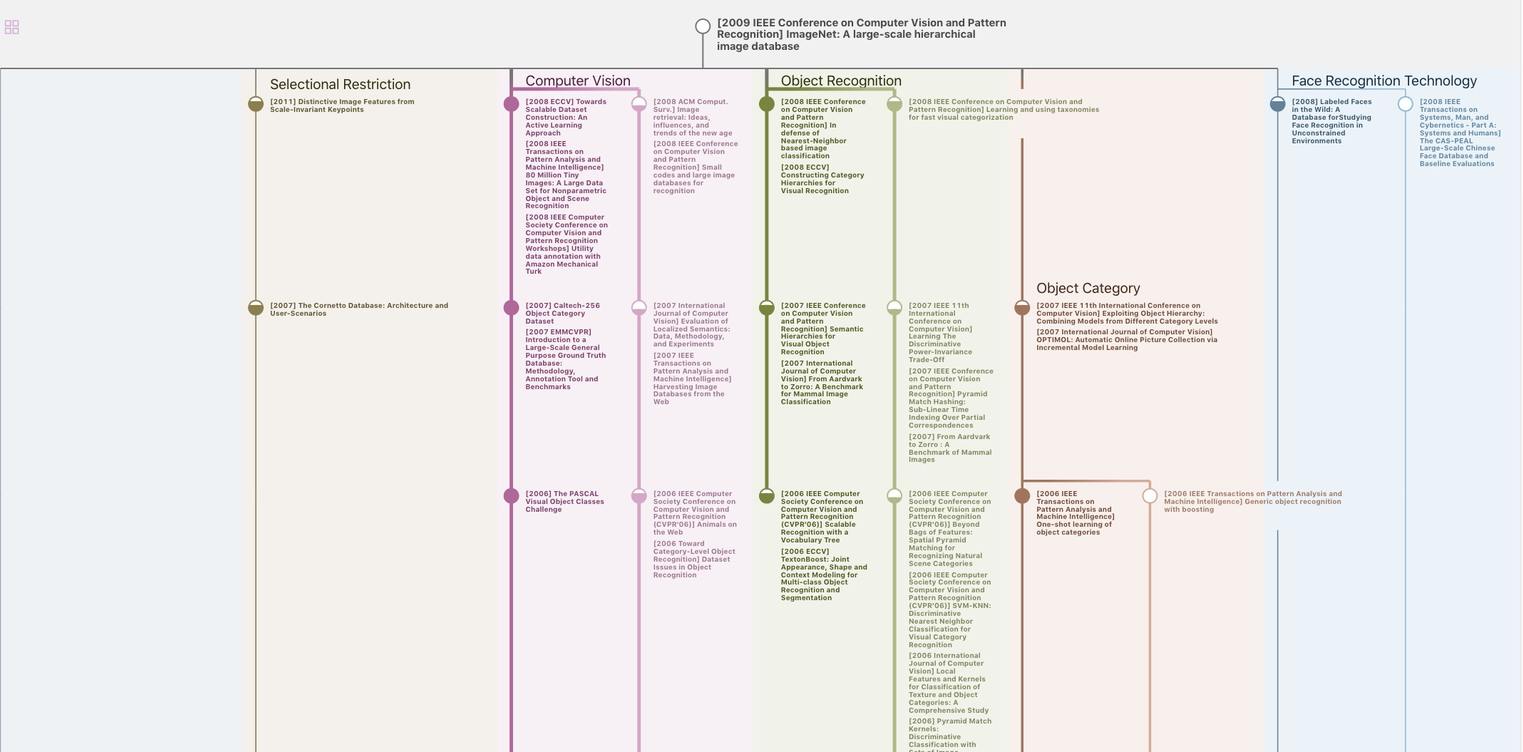
生成溯源树,研究论文发展脉络
Chat Paper
正在生成论文摘要