Fluid Micelle Network for Image Super-Resolution Reconstruction
IEEE transactions on cybernetics(2023)
摘要
Most existing convolutional neural-network-based super-resolution (SR) methods focus on designing effective neural blocks but rarely describe the image SR mechanism from the perspective of image evolution in the SR process. In this study, we explore a new research routine by abstracting the movement of pixels in the reconstruction process as the flow of fluid in the field of fluid dynamics (FD), where explicit motion laws of particles have been discovered. Specifically, a novel fluid micelle network is devised for image SR based on the theory of FD that follows the residual learning scheme but learns the residual structure by solving the finite difference equation in FD. The pixel motion equation in the SR process is derived from the Navier-Stokes (N-S) FD equation, establishing a guided branch that is aware of edge information. Thus, the second-order residual drives the network for feature extraction, and the guided branch corrects the direction of the pixel stream to supplement the details. Experiments on popular benchmarks and a real-world microscope chip image dataset demonstrate that the proposed method outperforms other modern methods in terms of both objective metrics and visual quality. The proposed method can also reconstruct clear geometric structures, offering the potential for real-world applications.
更多查看译文
关键词
Finite-difference equation,fluid dynamics (FD),fluid micelle network (FM-Net),image super-resolution (SR),Navier–Stokes (N-S) FD equation
AI 理解论文
溯源树
样例
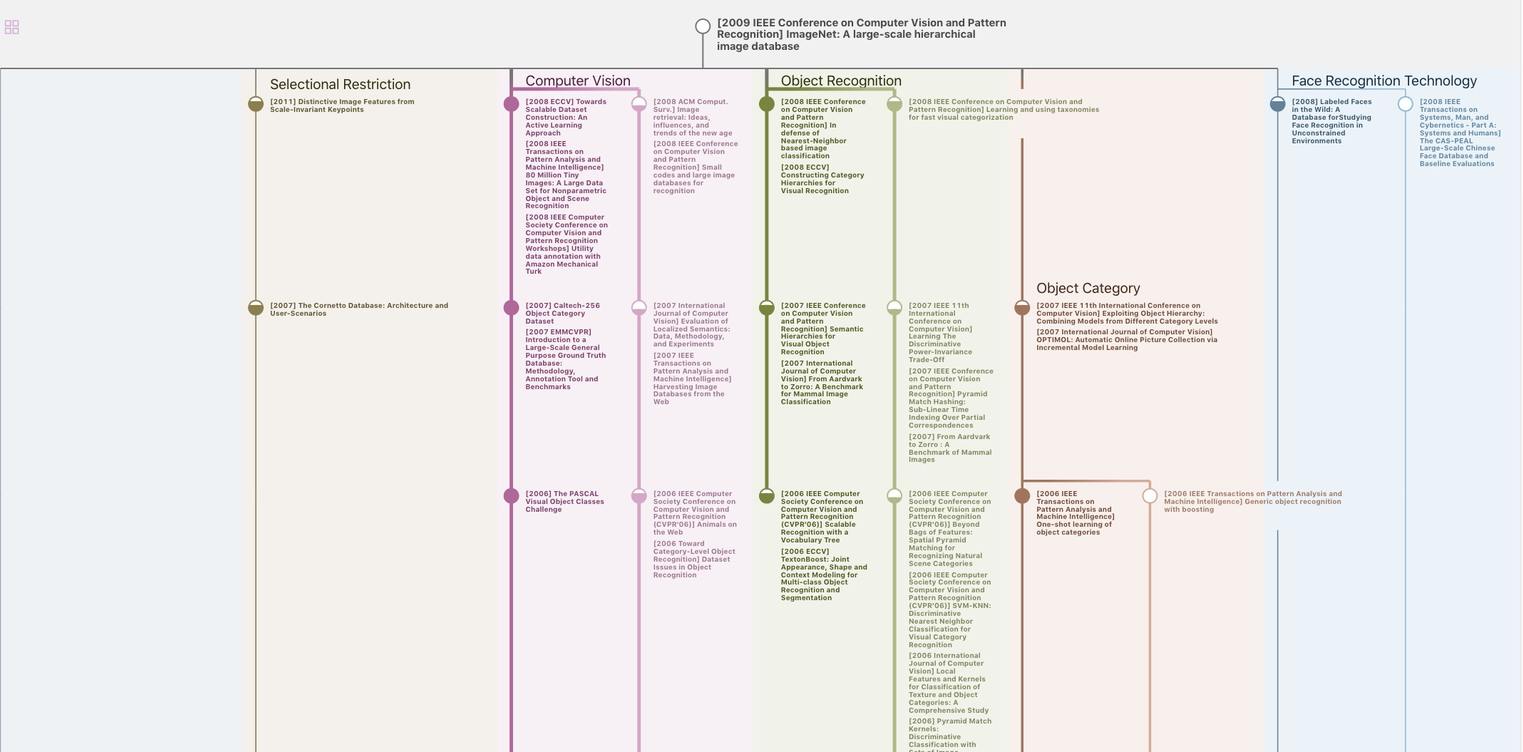
生成溯源树,研究论文发展脉络
Chat Paper
正在生成论文摘要