Resources and Power Efficient FPGA Accelerators for Real-Time Image Classification
JOURNAL OF IMAGING(2022)
摘要
A plethora of image and video-related applications involve complex processes that impose the need for hardware accelerators to achieve real-time performance. Among these, notable applications include the Machine Learning (ML) tasks using Convolutional Neural Networks (CNNs) that detect objects in image frames. Aiming at contributing to the CNN accelerator solutions, the current paper focuses on the design of Field-Programmable Gate Arrays (FPGAs) for CNNs of limited feature space to improve performance, power consumption and resource utilization. The proposed design approach targets the designs that can utilize the logic and memory resources of a single FPGA device and benefit mainly the edge, mobile and on-board satellite (OBC) computing; especially their image-processing- related applications. This work exploits the proposed approach to develop an FPGA accelerator for vessel detection on a Xilinx Virtex 7 XC7VX485T FPGA device (Advanced Micro Devices, Inc, Santa Clara, CA, USA). The resulting architecture operates on RGB images of size 80 x 80 or sliding windows; it is trained for the "Ships in Satellite Imagery" and by achieving frequency 270 MHz, completing the inference in 0.687 ms and consuming 5 watts, it validates the approach.
更多查看译文
关键词
image processing, CNN, accelerator, FPGA, vessel detection
AI 理解论文
溯源树
样例
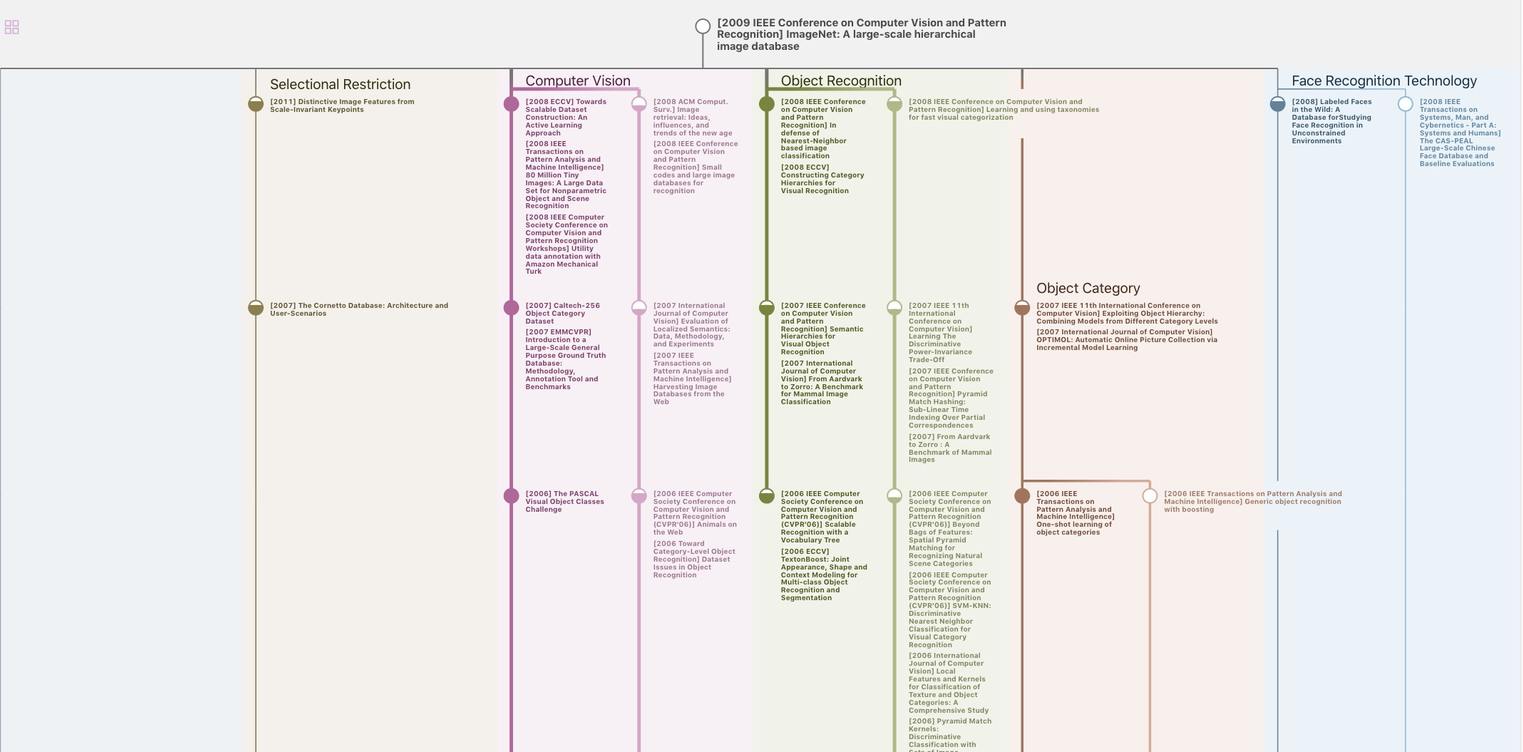
生成溯源树,研究论文发展脉络
Chat Paper
正在生成论文摘要