LVNet: Lightweight Model for Left Ventricle Segmentation for Short Axis Views in Echocardiographic Imaging
IEEE Transactions on Ultrasonics, Ferroelectrics, and Frequency Control(2022)
摘要
Lightweight segmentation models are becoming more popular for fast diagnosis on small and low cost medical imaging devices. This study focuses on the segmentation of the left ventricle (LV) in cardiac ultrasound (US) images. A new lightweight model [LV network (LVNet)] is proposed for segmentation, which gives the benefits of requiring fewer parameters but with improved segmentation performance in terms of Dice score (DS). The proposed model is compared with state-of-the-art methods, such as UNet, MiniNetV2, and fully convolutional dense dilated network (FCdDN). The model proposed comes with a post-processing pipeline that further enhances the segmentation results. In general, the training is done directly using the segmentation mask as the output and the US image as the input of the model. A new strategy for segmentation is also introduced in addition to the direct training method used. Compared with the UNet model, an improvement in DS performance as high as 5% for segmentation with papillary (WP) muscles was found, while showcasing an improvement of 18.5% when the papillary muscles are excluded. The model proposed requires only 5% of the memory required by a UNet model. LVNet achieves a better trade-off between the number of parameters and its segmentation performance as compared with other conventional models. The developed codes are available at
https://github.com/navchetanawasthi/Left_Ventricle_Segmentation
.
更多查看译文
关键词
Echocardiography,Heart Ventricles,Image Processing, Computer-Assisted,Muscles,Ultrasonography
AI 理解论文
溯源树
样例
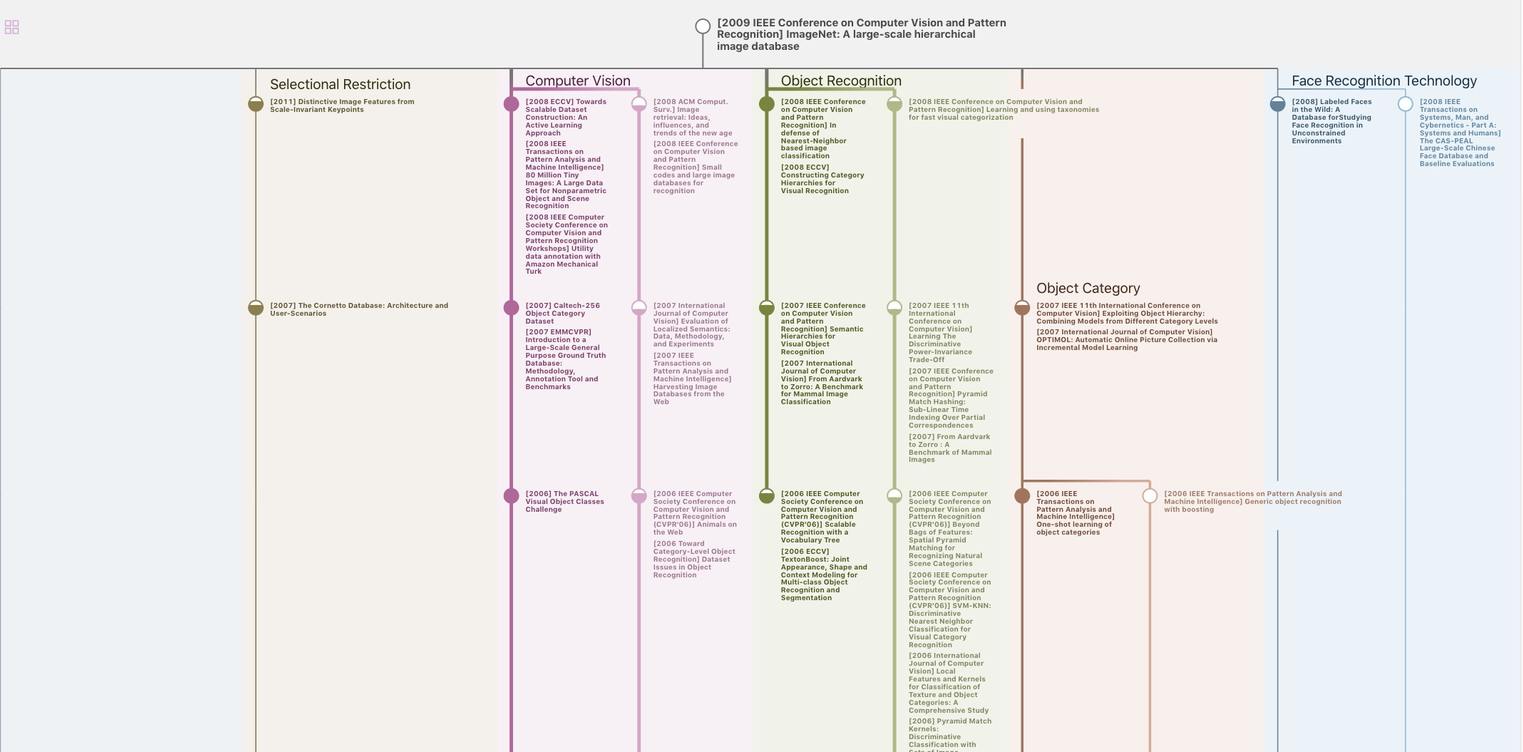
生成溯源树,研究论文发展脉络
Chat Paper
正在生成论文摘要