Roles of Physicochemical and Structural Properties of RNA-Binding Proteins in Predicting the Activities of Trans-Acting Splicing Factors with Machine Learning.
International journal of molecular sciences(2022)
摘要
Trans-acting splicing factors play a pivotal role in modulating alternative splicing by specifically binding to cis-elements in pre-mRNAs. There are approximately 1500 RNA-binding proteins (RBPs) in the human genome, but the activities of these RBPs in alternative splicing are unknown. Since determining RBP activities through experimental methods is expensive and time consuming, the development of an efficient computational method for predicting the activities of RBPs in alternative splicing from their sequences is of great practical importance. Recently, a machine learning model for predicting the activities of splicing factors was built based on features of single and dual amino acid compositions. Here, we explored the role of physicochemical and structural properties in predicting their activities in alternative splicing using machine learning approaches and found that the prediction performance is significantly improved by including these properties. By combining the minimum redundancy–maximum relevance (mRMR) method and forward feature searching strategy, a promising feature subset with 24 features was obtained to predict the activities of RBPs. The feature subset consists of 16 dual amino acid compositions, 5 physicochemical features, and 3 structural features. The physicochemical and structural properties were as important as the sequence composition features for an accurate prediction of the activities of splicing factors. The hydrophobicity and distribution of coil are suggested to be the key physicochemical and structural features, respectively.
更多查看译文
关键词
machine learning,partial least square regression,minimum redundancy-maximum relevance,forward searching strategy,hydrophobicity,secondary structure,amino acid compositions
AI 理解论文
溯源树
样例
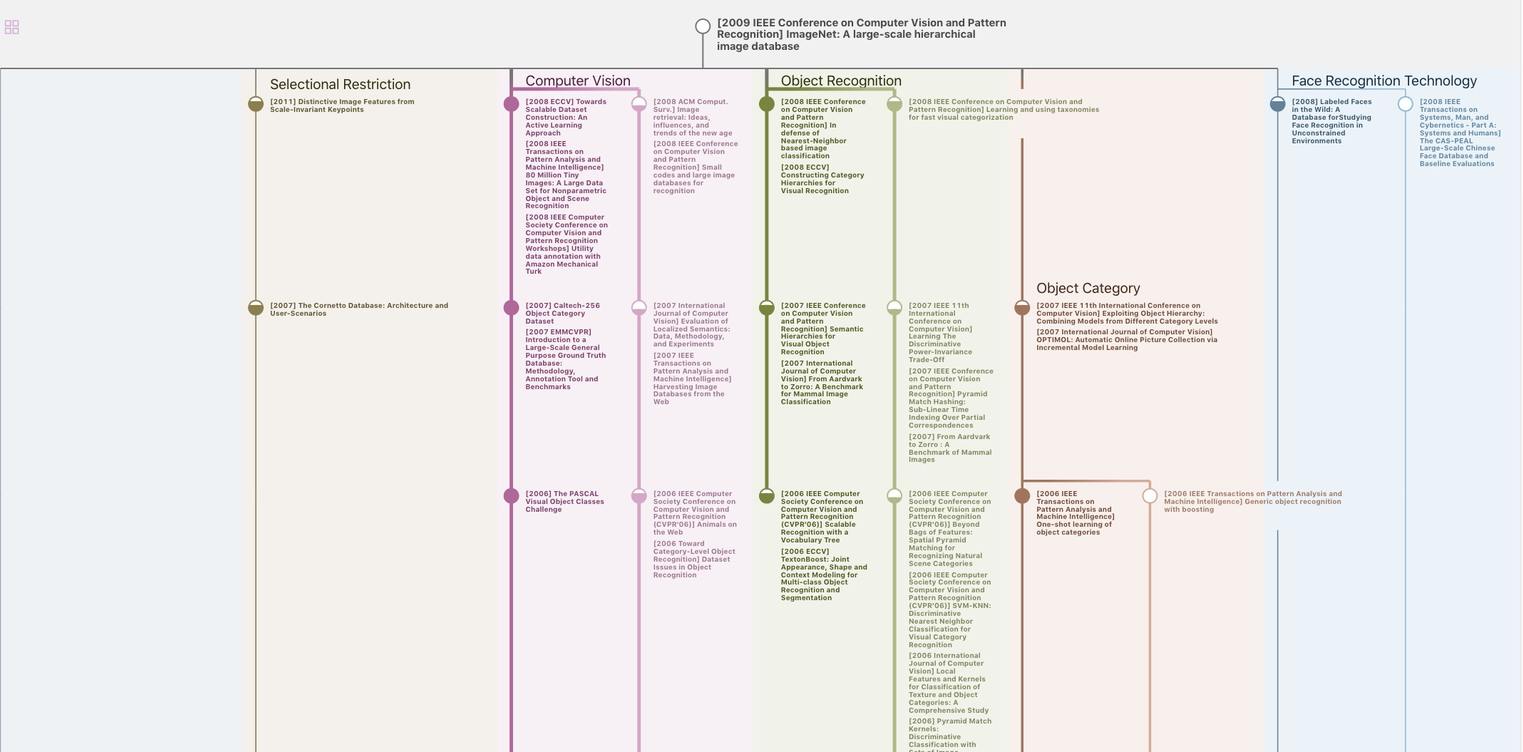
生成溯源树,研究论文发展脉络
Chat Paper
正在生成论文摘要