Implicit Object Mapping With Noisy Data
arxiv(2022)
摘要
Modelling individual objects in a scene as Neural Radiance Fields (NeRFs) provides an alternative geometric scene representation that may benefit downstream robotics tasks such as scene understanding and object manipulation. However, we identify three challenges to using real-world training data collected by a robot to train a NeRF: (i) The camera trajectories are constrained, and full visual coverage is not guaranteed - especially when obstructions to the objects of interest are present; (ii) the poses associated with the images are noisy due to odometry or localization noise; (iii) the objects are not easily isolated from the background. This paper evaluates the extent to which above factors degrade the quality of the learnt implicit object representation. We introduce a pipeline that decomposes a scene into multiple individual object-NeRFs, using noisy object instance masks and bounding boxes, and evaluate the sensitivity of this pipeline with respect to noisy poses, instance masks, and the number of training images. We uncover that the sensitivity to noisy instance masks can be partially alleviated with depth supervision and quantify the importance of including the camera extrinsics in the NeRF optimisation process.
更多查看译文
关键词
implicit object mapping,data
AI 理解论文
溯源树
样例
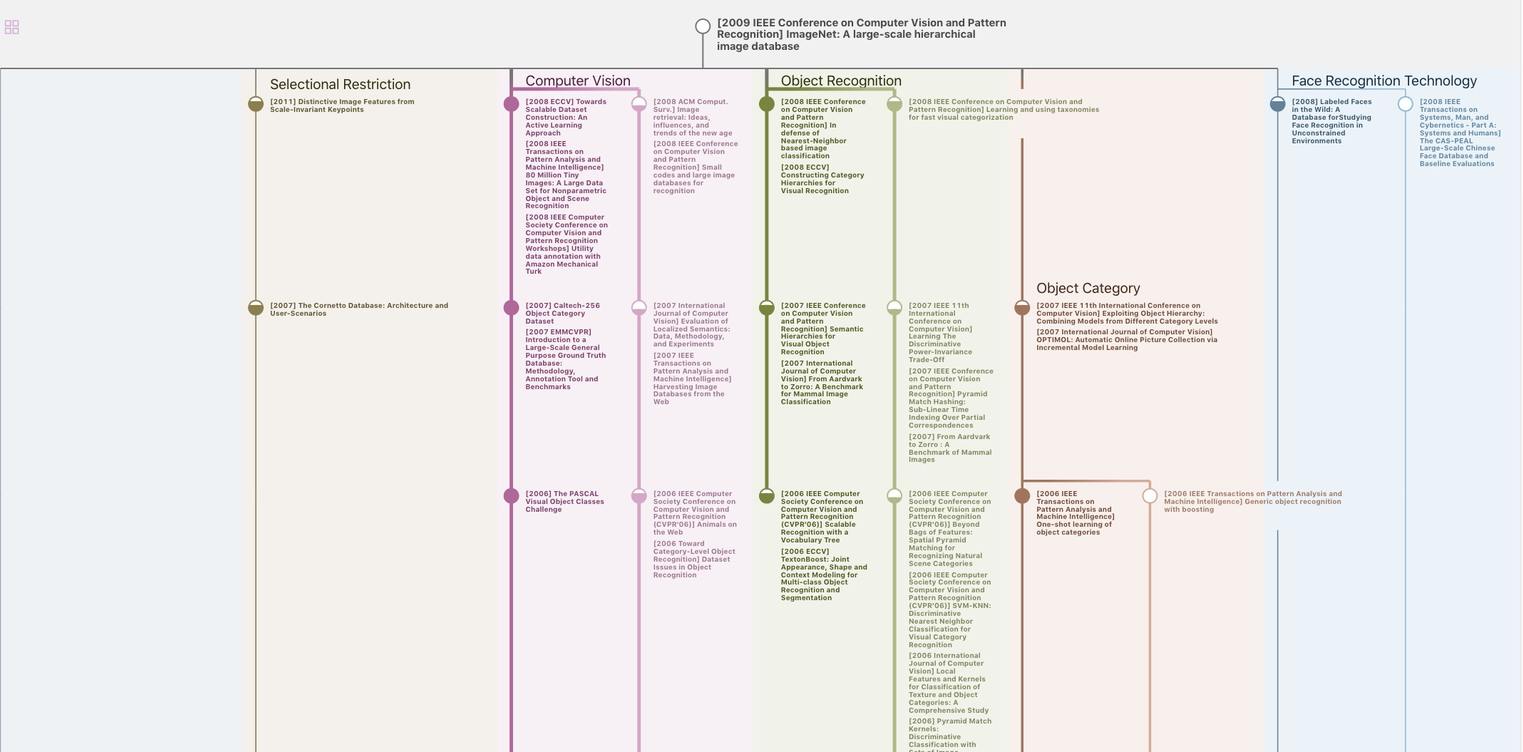
生成溯源树,研究论文发展脉络
Chat Paper
正在生成论文摘要