Zero and Few-Shot Learning for Author Profiling
NATURAL LANGUAGE PROCESSING AND INFORMATION SYSTEMS (NLDB 2022)(2022)
摘要
Author profiling classifies author characteristics by analyzing how language is shared among people. In this work, we study that task from a low-resource viewpoint: using little or no training data. We explore different zero and few-shot models based on entailment and evaluate our systems on several profiling tasks in Spanish and English. In addition, we study the effect of both the entailment hypothesis and the size of the few-shot training sample. We find that entailment-based models outperform supervised text classifiers based on roberta-XLM and that we can reach 80% of the accuracy of previous approaches using less than 50% of the training data on average.
更多查看译文
关键词
Author profiling, Zero-shot text classification, Few-shot text classification, Entailment
AI 理解论文
溯源树
样例
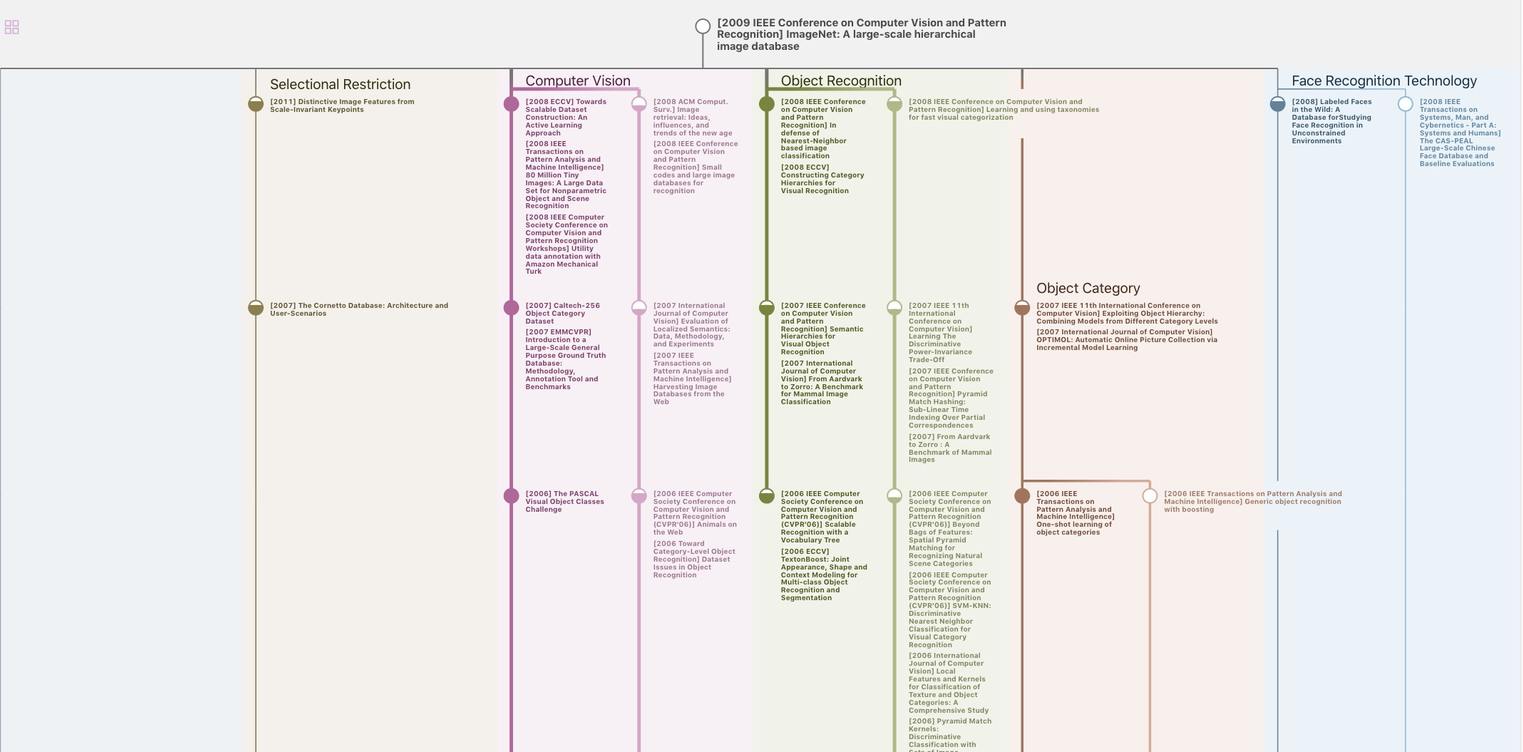
生成溯源树,研究论文发展脉络
Chat Paper
正在生成论文摘要