Paramixer: Parameterizing Mixing Links in Sparse Factors Works Better Than Dot-Product Self-Attention
2022 IEEE/CVF Conference on Computer Vision and Pattern Recognition (CVPR)(2022)
摘要
Self-Attention is a widely used building block in neural modeling to mix long-range data elements. Most self-attention neural networks employ pairwise dot-products to specify the attention coefficients. However, these methods require O(N^2) computing cost for sequence length N. Even though some approximation methods have been introduced to relieve the quadratic cost, the performance of the dot-product approach is still bottlenecked by the low-rank constraint in the attention matrix factorization. In this paper, we propose a novel scalable and effective mixing building block called Paramixer. Our method factorizes the interaction matrix into several sparse matrices, where we parameterize the non-zero entries by MLPs with the data elements as input. The overall computing cost of the new building block is as low as O(N log N). Moreover, all factorizing matrices in Paramixer are full-rank, so it does not suffer from the low-rank bottleneck. We have tested the new method on both synthetic and various real-world long sequential data sets and compared it with several state-of-the-art attention networks. The experimental results show that Paramixer has better performance in most learning tasks.
更多查看译文
关键词
Deep learning architectures and techniques,Machine learning,Representation learning
AI 理解论文
溯源树
样例
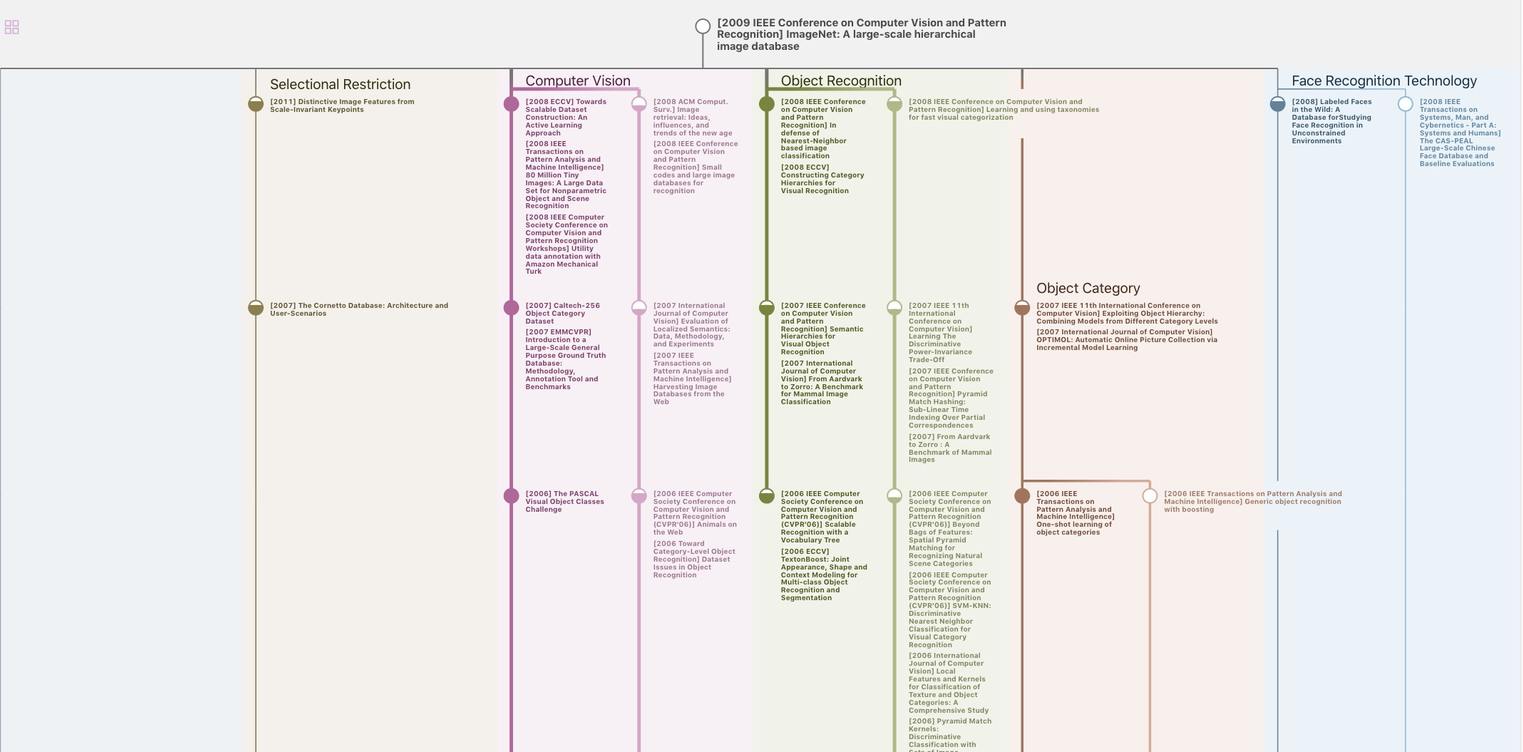
生成溯源树,研究论文发展脉络
Chat Paper
正在生成论文摘要