Towards Accelerated Localization Performance Across Indoor Positioning Datasets
2022 International Conference on Localization and GNSS (ICL-GNSS)(2022)
摘要
The localization speed and accuracy in the indoor scenario can greatly impact the Quality of Experience of the user. While many individual machine learning models can achieve comparable positioning performance, their prediction mechanisms offer different complexity to the system. In this work, we propose a fingerprinting positioning method for multi-building and multi-floor deployments, composed of a cascade of three models for building classification, floor classification, and 2D localization regression. We conduct an exhaustive search for the optimally performing one in each step of the cascade while validating on 14 different openly available datasets. As a result, we bring forward the best-performing combination of models in terms of overall positioning accuracy and processing speed and evaluate on independent sets of samples. We reduce the mean prediction time by 71% while achieving comparable positioning performance across all considered datasets. Moreover, in case of voluminous training dataset, the prediction time is reduced down to 1% of the benchmark's.
更多查看译文
关键词
Cascade,Fingerprinting,Indoor positioning,Localization,Machine learning,Prediction speed
AI 理解论文
溯源树
样例
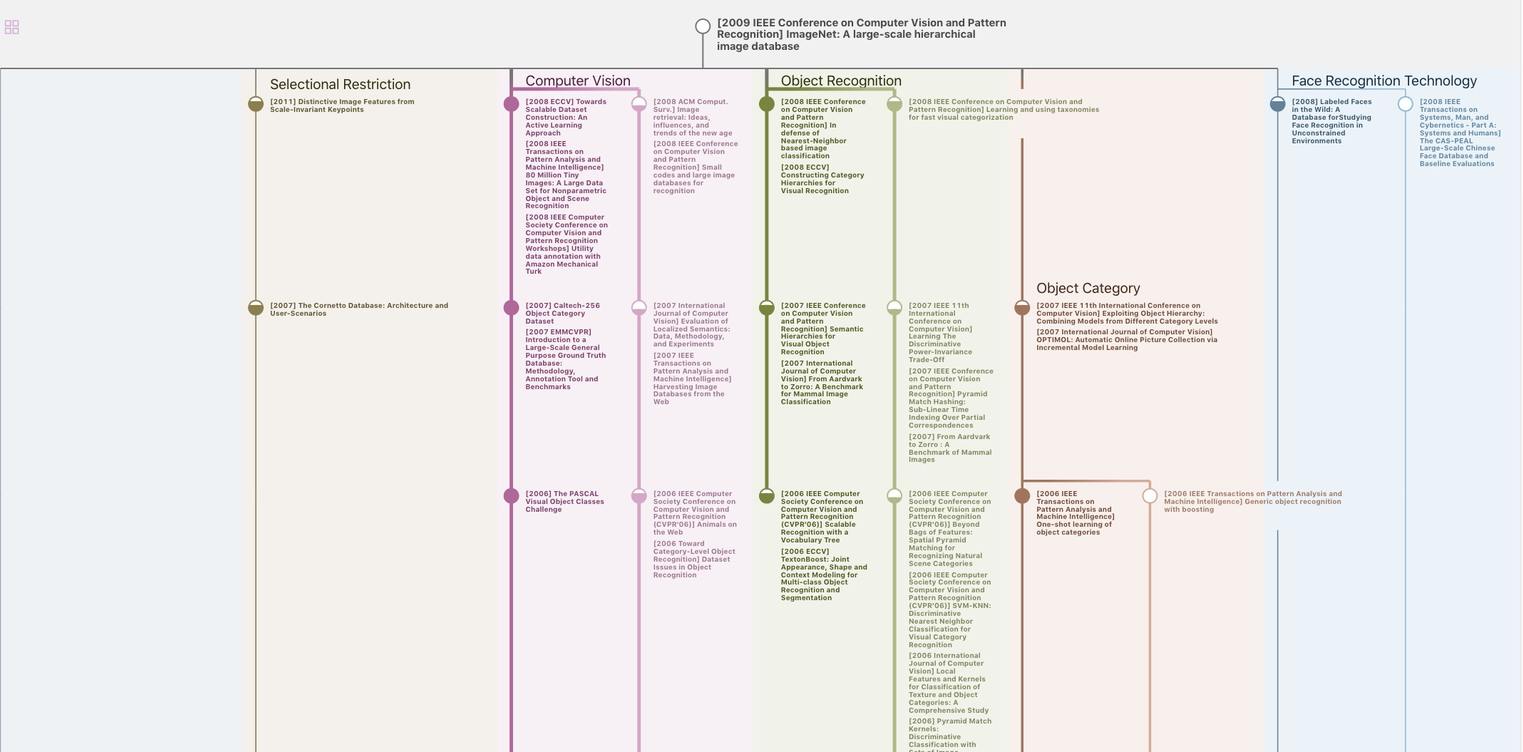
生成溯源树,研究论文发展脉络
Chat Paper
正在生成论文摘要