Source free domain adaptation for medical image segmentation with fourier style mining
Medical Image Analysis(2022)
摘要
•We propose a SFDA framework with Fourier style mining for medical image segmentation, composing of a generation stage and an adaptation stage. To the best of our knowledge, this represents the first effort to solve unsupervised domain adaptation with only the source model on medical image segmentation.•In the generation stage, we propose a Fourier Style Mining (FSM) generator to produce high-quality source-like images from the well-trained source segmentation model through BN constraints and mutual Fourier Transform. FSM represents the first attempt to generate source-like images from a segmentation model to deal with domain shift in SFDA.•In the adaptation stage, CDD and CADC modules are proposed to achieve featurelevel and output-level alignment based on paired source-like and target images. The CADC introduces shape compactness metric to filter out noisy pseudo labels for medical image segmentation.•Comprehensive experiments on different domain scenarios (including modalities, vendors and clinical cites), demonstrate the effectiveness of our style mining guided SFDA framework, which outperforms state -of-the-art domain adaptation methods.
更多查看译文
关键词
Source Free Domain Adaptation,Fourier Style Mining,Contrastive Domain Distillation,Consistency Learning
AI 理解论文
溯源树
样例
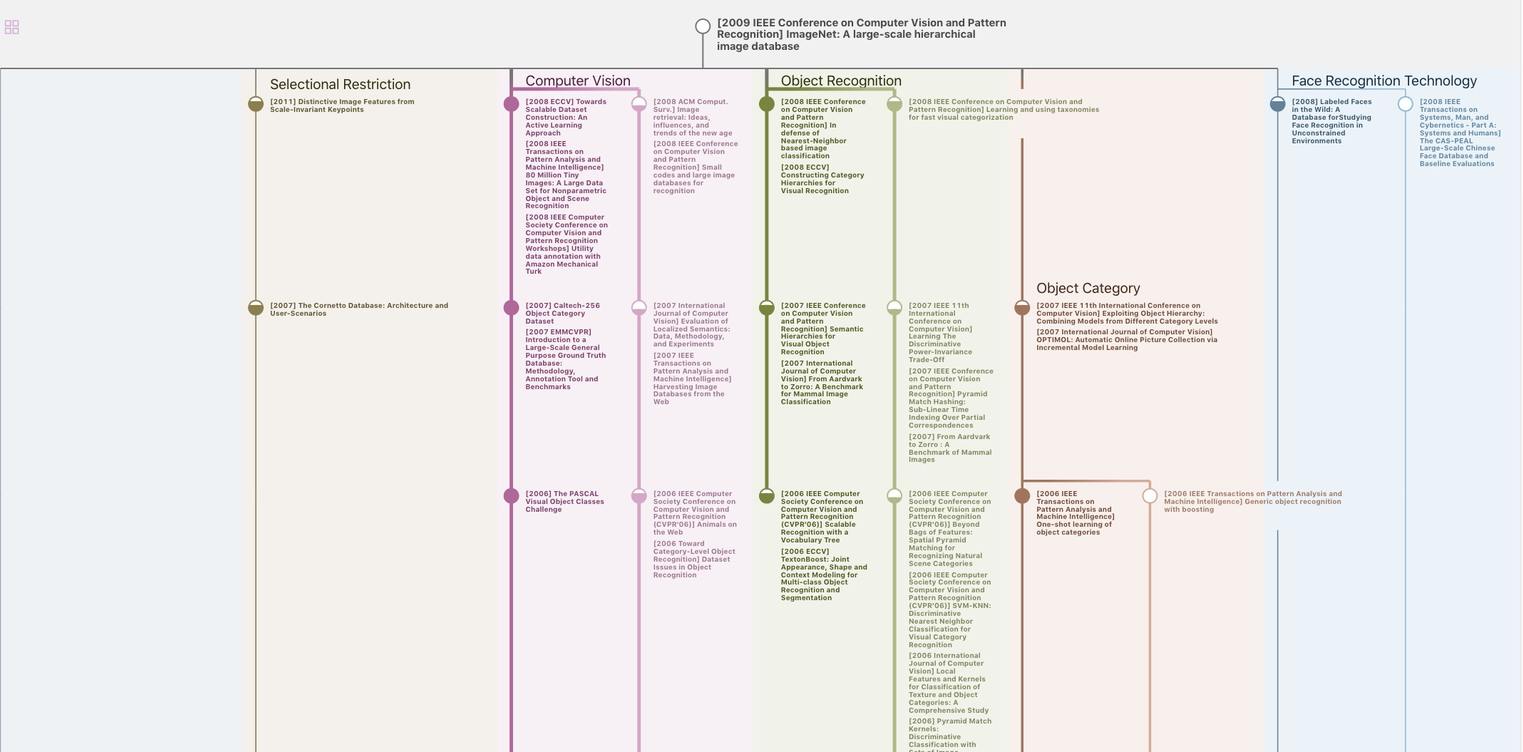
生成溯源树,研究论文发展脉络
Chat Paper
正在生成论文摘要