Cross-Modal Transfer Learning From EEG to Functional Near-Infrared Spectroscopy for Classification Task in Brain-Computer Interface System
FRONTIERS IN PSYCHOLOGY(2022)
摘要
The brain-computer interface (BCI) based on functional near-infrared spectroscopy (fNIRS) has received more and more attention due to its vast application potential in emotion recognition. However, the relatively insufficient investigation of the feature extraction algorithms limits its use in practice. In this article, to improve the performance of fNIRS-based BCI, we proposed a method named R-CSP-E, which introduces EEG signals when computing fNIRS signals' features based on transfer learning and ensemble learning theory. In detail, we used the Independent Component Analysis (ICA) algorithm for the correspondence between the sources of the two signals. We then introduced the EEG signals when computing the spatial filter based on a modified Common Spatial Pattern (CSP) algorithm. Experimental results on public datasets show that the proposed method in this paper outperforms traditional methods without transfer. In general, the mean classification accuracy can be increased by up to 5%. To our knowledge, it is an innovation that we tried to apply transfer learning between EEG and fNIRS. Our study's findings not only prove the potential of the transfer learning algorithm in cross-model brain-computer interface, but also offer a new and innovative perspective to research the hybrid brain-computer interface.
更多查看译文
关键词
fNIRS signals, transfer learning, brain computer interface, ICA, RCSP
AI 理解论文
溯源树
样例
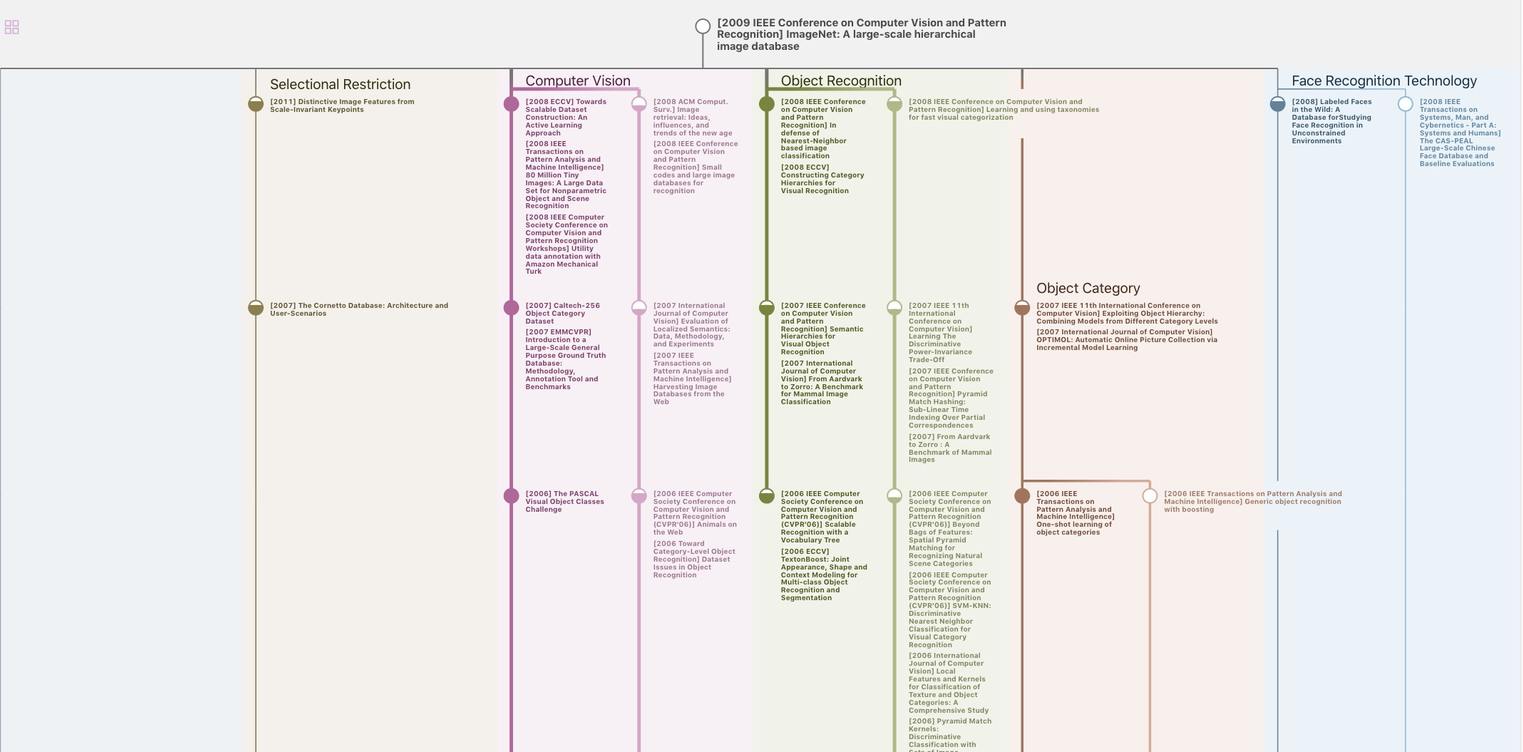
生成溯源树,研究论文发展脉络
Chat Paper
正在生成论文摘要