Multi-granulation Multi-scale Relation Network for Abstract Reasoning
International Journal of Machine Learning and Cybernetics(2022)
摘要
Abstract reasoning, one of the representative works of logic learning, is to make machines intelligent. To test the intelligence of machines, researchers have proposed multiple benchmark data sets and these date sets mainly consist of a few simple geometries and traced reasoning paths. There are three issues for these data sets: (1) it is relatively easy for machines to reason the right answer from the simple geometries; (2) due to the limited number of geometric shapes, these data sets are prone to disclosure of information about reasoning; (3) all traced reasoning paths in these data sets have been known beforehand, some state-of-the-art reasoning models are specially designed according to these paths. Hence, these benchmark data sets cannot truly reflect the reasoning ability of reasoning models. To address these issues, we propose a Fashion Non-descending Path data set (FNP). FNP is designed using a mass of complex samples from Fashion-MNIST data set as objects of FNP and the non-descending path that is a more complex path as the variation directions of logical patterns. For gaining reasoning performance on FNP, inspired by the multi-granulation and multi-scale ideas, we propose a multi-granulation multi-scale relation network (M2RN) to consider the multi-wise relations rather than the simple pair-wise relations. Experimental results show that the M2RN is effective for abstract reasoning task.
更多查看译文
关键词
Multi-granulation, Multi-scale, Non-descending path, Abstract reasoning, Relation network
AI 理解论文
溯源树
样例
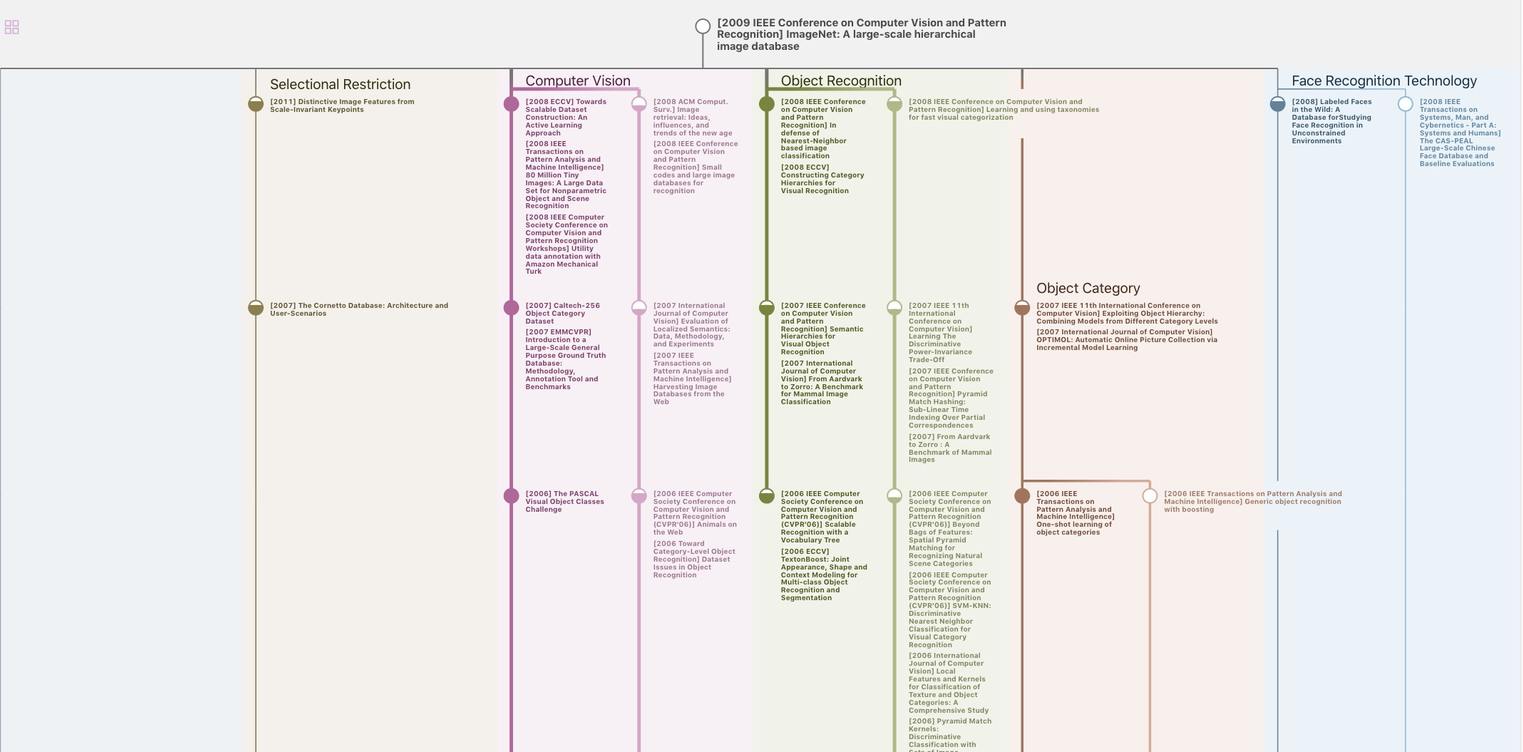
生成溯源树,研究论文发展脉络
Chat Paper
正在生成论文摘要