Baseline Evaluation Methodology for Adversarial Patterns on Object Detection Models
2021 IEEE Applied Imagery Pattern Recognition Workshop (AIPR)(2021)
摘要
Physically realizable adversarial patterns have become a sophisticated form of adversarial ML across industry and academia, with several algorithms that successfully thwart state-of-the-art ML models. However, there are currently no standard practices for evaluating adversarial patterns. We identify two components that provide insight into an adversarial pattern's performance: the seeding of the algorithm for producing the adversarial pattern and the use of control patterns (patterns that serve as the baseline comparisons to the adversarial pattern). In this study, we implement and compare the performance of a variety of control patterns (solid white, solid gray, solid black, and random noise). We train state-of-the-art DNN object detection models on an open-source dataset. Using the trained models, we evaluate performance on the various control patterns in order to establish performance baselines for current and future adversarial pattern algorithms.
更多查看译文
关键词
adversarial machine learning,ML,attacks,baseline,computer vision,AI,security
AI 理解论文
溯源树
样例
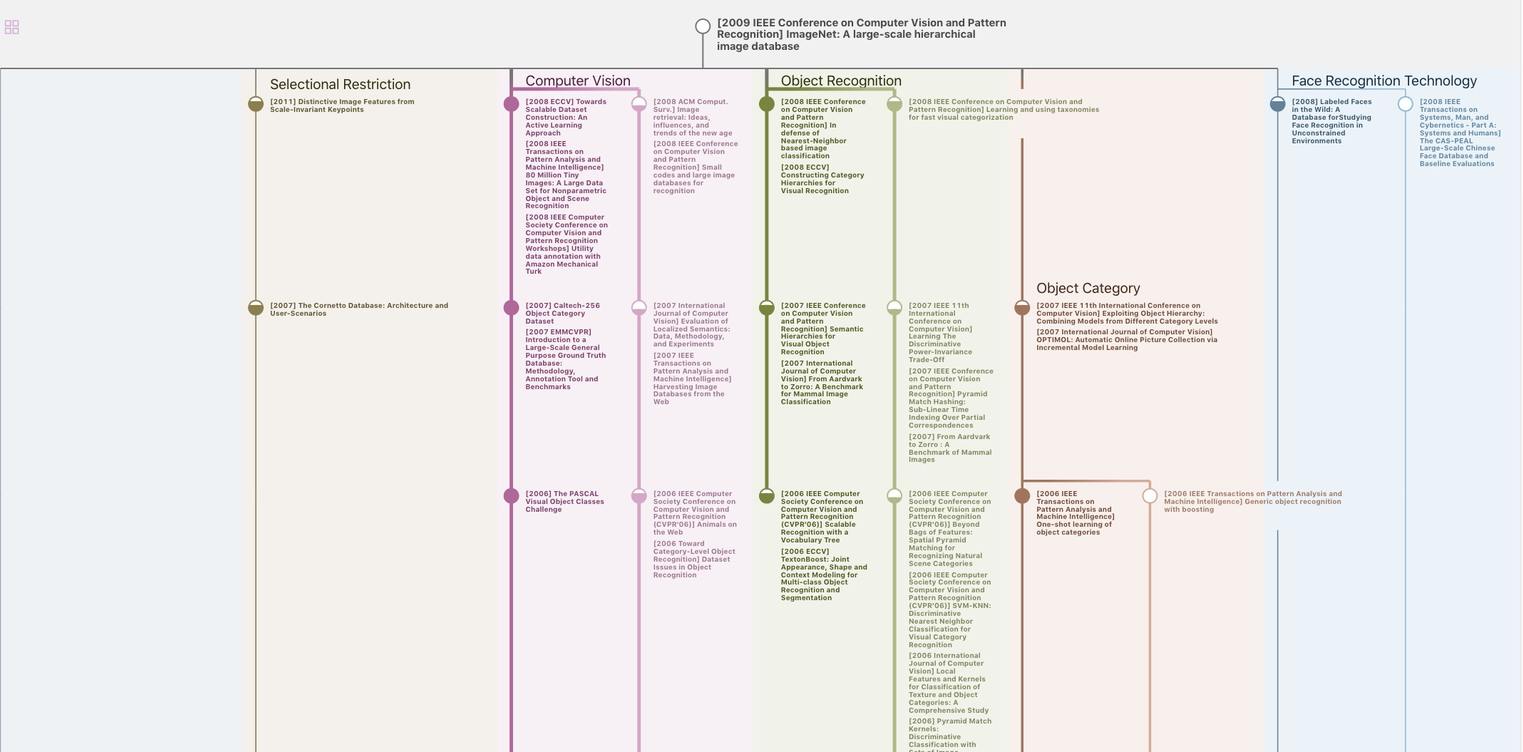
生成溯源树,研究论文发展脉络
Chat Paper
正在生成论文摘要