DGInet: Dynamic graph and interaction-aware convolutional network for vehicle trajectory prediction
Neural Networks(2022)
摘要
This paper investigates vehicle trajectory prediction problems in real traffic scenarios by fully harnessing the spatio-temporal dependencies between multiple vehicles. The existing GCN-based trajectory predictions are often considered in a single traffic scene without time attributes, complete interaction information, dynamic graph-based model, etc. Time and interaction aware models are more challenging than the existing ones. Despite very well does the graph-based model describe the relationship between driving vehicles, the critical problem in the traffic scene is how to deeply explore the spatio-temporal characteristics of dynamic graphs. Therefore, a novel dynamic graph and interaction-aware neural network model called as DGInet is proposed by combining a semi-global graph mechanism and an M-product based graph convolutional network, which are built into novel dual-network architecture in the entire model. The DGInet is built by exploiting the dynamic interaction in depth between driving vehicles in urban traffic scenarios, and then realized by utilizing semi-global graph convolution operations on the input data cell to capture the basic spatial interaction features of the driving scene. Meanwhile, the dynamic graph is further extracted by a novel M-product approach, in which the embedding of the model is then established along with the embedding of the semi-global network to perform the final embedding. Extensive experiments have been conducted on the two public datasets, named NGSIM and Apollo respectively, to show that our approach outperforms the existing ones with better performance and less computing time. Besides the real-world Shenzhen traffic dataset, China, is also developed to verify the effectiveness of our approach.
更多查看译文
关键词
Vehicle trajectory prediction,Graph convolutional network,Dynamic graph,Interaction-aware network,M-product
AI 理解论文
溯源树
样例
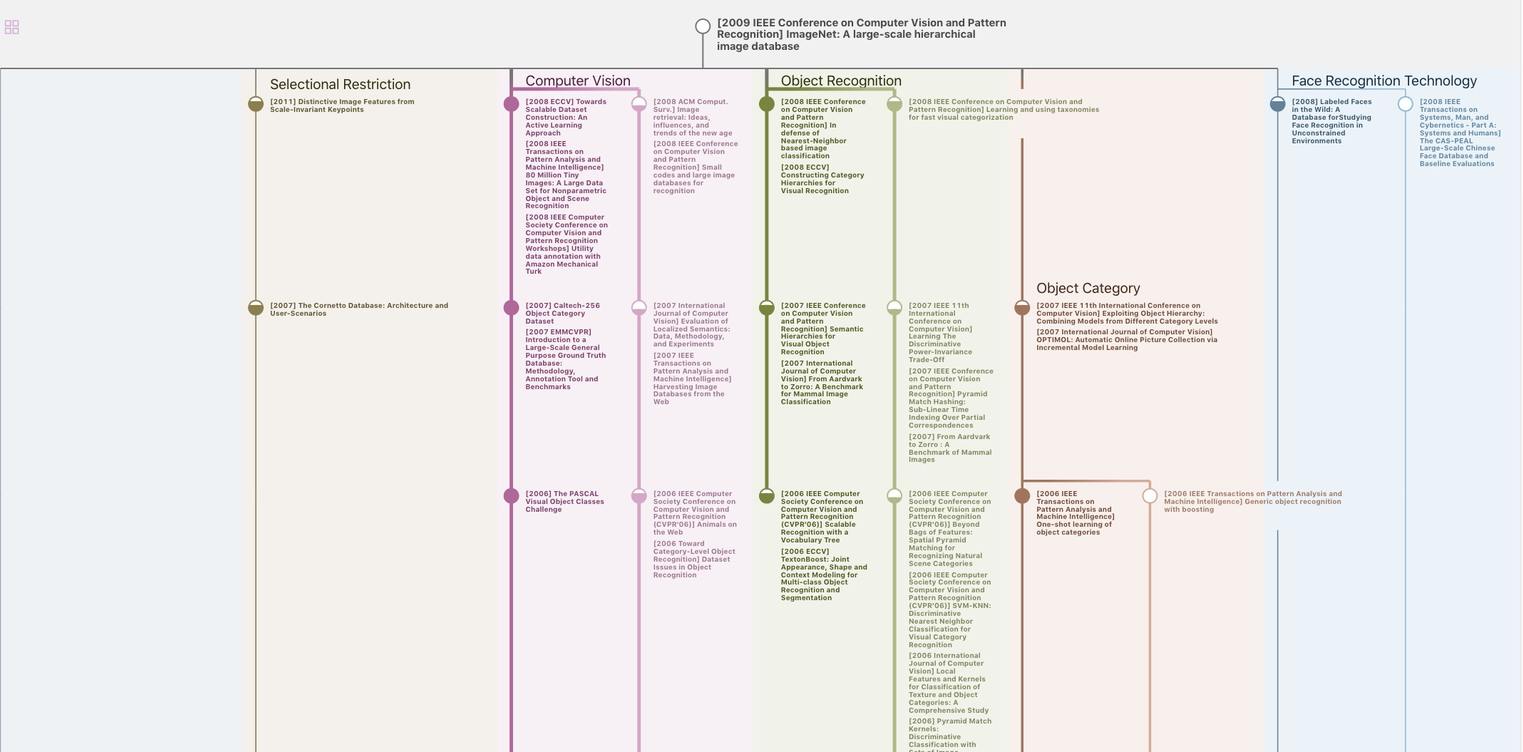
生成溯源树,研究论文发展脉络
Chat Paper
正在生成论文摘要