Relevance-based Margin for Contrastively-trained Video Retrieval Models
PROCEEDINGS OF THE 2022 INTERNATIONAL CONFERENCE ON MULTIMEDIA RETRIEVAL, ICMR 2022(2022)
摘要
Video retrieval using natural language queries has attracted increasing interest due to its relevance in real-world applications, from intelligent access in private media galleries to web-scale video search. Learning the cross-similarity of video and text in a joint embedding space is the dominant approach. To do so, a contrastive loss is usually employed because it organizes the embedding space by putting similar items close and dissimilar items far. This framework leads to competitive recall rates, as they solely focus on the rank of the groundtruth items. Yet, assessing the quality of the ranking list is of utmost importance when considering intelligent retrieval systems, since multiple items may share similar semantics, hence a high relevance. Moreover, the aforementioned framework uses a fixed margin to separate similar and dissimilar items, treating all non-groundtruth items as equally irrelevant. In this paper we propose to use a variable margin: we argue that varying the margin used during training based on how much relevant an item is to a given query, i.e. a relevance-based margin, easily improves the quality of the ranking lists measured through nDCG and mAP. We demonstrate the advantages of our technique using different models on EPIC-Kitchens-100 and YouCook2. We show that even if we carefully tuned the fixed margin, our technique (which does not have the margin as a hyper-parameter) would still achieve better performance. Finally, extensive ablation studies and qualitative analysis support the robustness of our approach. Code will be released at https://github.com/aranciokov/RelevanceMargin- ICMR22.
更多查看译文
关键词
deep learning,cross-modal retrieval,video retrieval,relevance
AI 理解论文
溯源树
样例
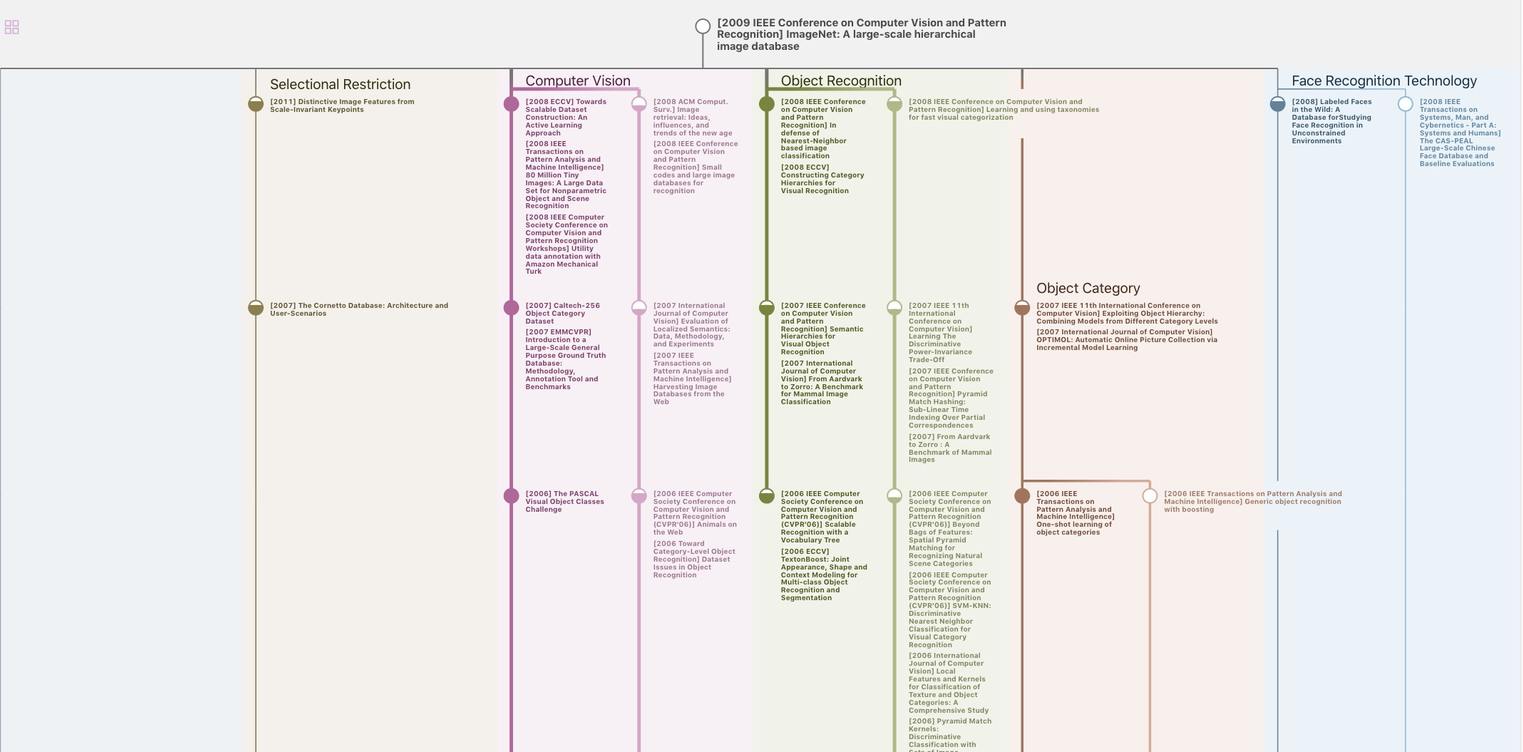
生成溯源树,研究论文发展脉络
Chat Paper
正在生成论文摘要