NLU++: A Multi-Label, Slot-Rich, Generalisable Dataset for Natural Language Understanding in Task-Oriented Dialogue.
The Annual Conference of the North American Chapter of the Association for Computational Linguistics(2022)
摘要
We present NLU++, a novel dataset for natural language understanding (NLU) in task-oriented dialogue (ToD) systems, with the aim to provide a much more challenging evaluation environment for dialogue NLU models, up to date with the current application and industry requirements. NLU++ is divided into two domains (BANKING and HOTELS) and brings several crucial improvements over current commonly used NLU datasets. \textbf{1)} NLU++ provides fine-grained domain ontologies with a large set of challenging \textit{multi-intent} sentences, introducing and validating the idea of \textit{intent modules} that can be combined into complex intents that convey complex user goals, combined with finer-grained and thus more challenging slot sets. \textbf{2)} The ontology is divided into \textit{domain-specific} and \textit{generic} (i.e., domain-universal) intent modules that overlap across domains, promoting cross-domain reusability of annotated examples. \textbf{3)} The dataset design has been inspired by the problems observed in industrial ToD systems, and \textbf{4)} it has been collected, filtered and carefully annotated by dialogue NLU experts, yielding high-quality annotated data. Finally, we benchmark a series of current state-of-the-art NLU models on NLU++; the results demonstrate the challenging nature of the dataset, especially in low-data regimes, the validity of `intent modularisation', and call for further research on ToD NLU.
更多查看译文
关键词
dialogue,language understanding,multi-label,slot-rich,task-oriented
AI 理解论文
溯源树
样例
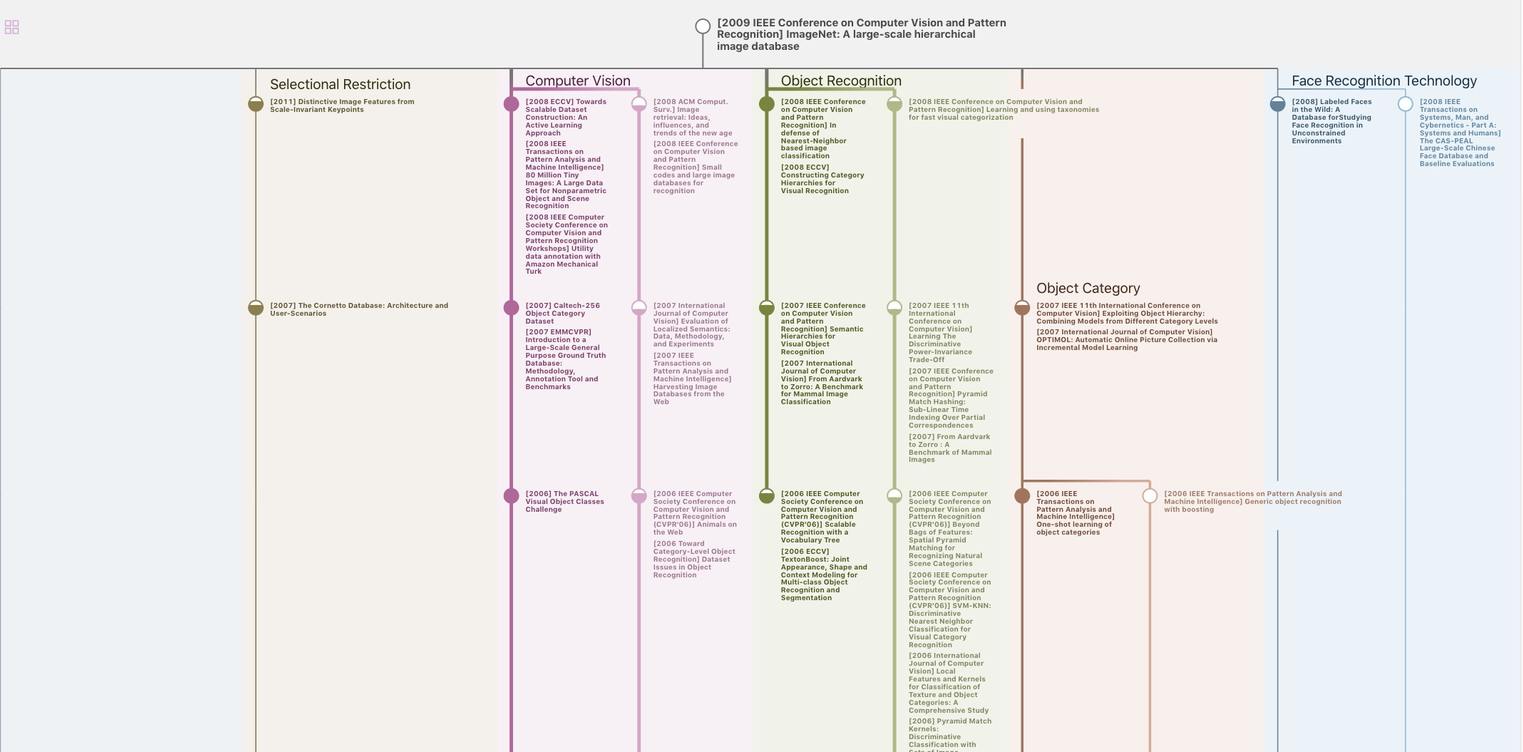
生成溯源树,研究论文发展脉络
Chat Paper
正在生成论文摘要