Scale-aware attention network for weakly supervised semantic segmentation
Neurocomputing(2022)
摘要
Weakly supervised semantic segmentation (WSSS) using image-level labels greatly alleviates the burden of obtaining large amounts of pixel-wise annotations. To create pseudo segmentation labels, most WSSS algorithms rely on the local response regions in the class activation maps (CAMs). However, such activation maps only focus on the local discriminative parts of the object, because the classification network does not require the entire object to optimize the objective function. To enhance the network’s ability to focus more on non-discriminative parts of the object and generate high-quality pseudo-masks, the Scale-aware Attention Network (SAN) is proposed. Specifically, a pyramidal attention module is introduced to propagate discriminative information to adjacent object regions by adaptively selecting contextual features from the convolutional pyramid with varied filter scales. A multi-scale prediction fusion structure with a joint loss is proposed to make better use of the complementary information of localization maps in different scales. The dense and integral localization maps are obtained in the inference stage by weighted fusion of the multi-scale predictions, which are then used to train segmentation models. This novel SAN has demonstrated its effectiveness in a series of experiments. It has achieved a state-of-the-art result of 71.9% mIoU on the PASCAL VOC 2012 segmentation test set compared with other approaches under the same level of supervision.
更多查看译文
关键词
Weakly supervised semantic segmentation,Class activation map,Multi-scale feature,Attention mechanism
AI 理解论文
溯源树
样例
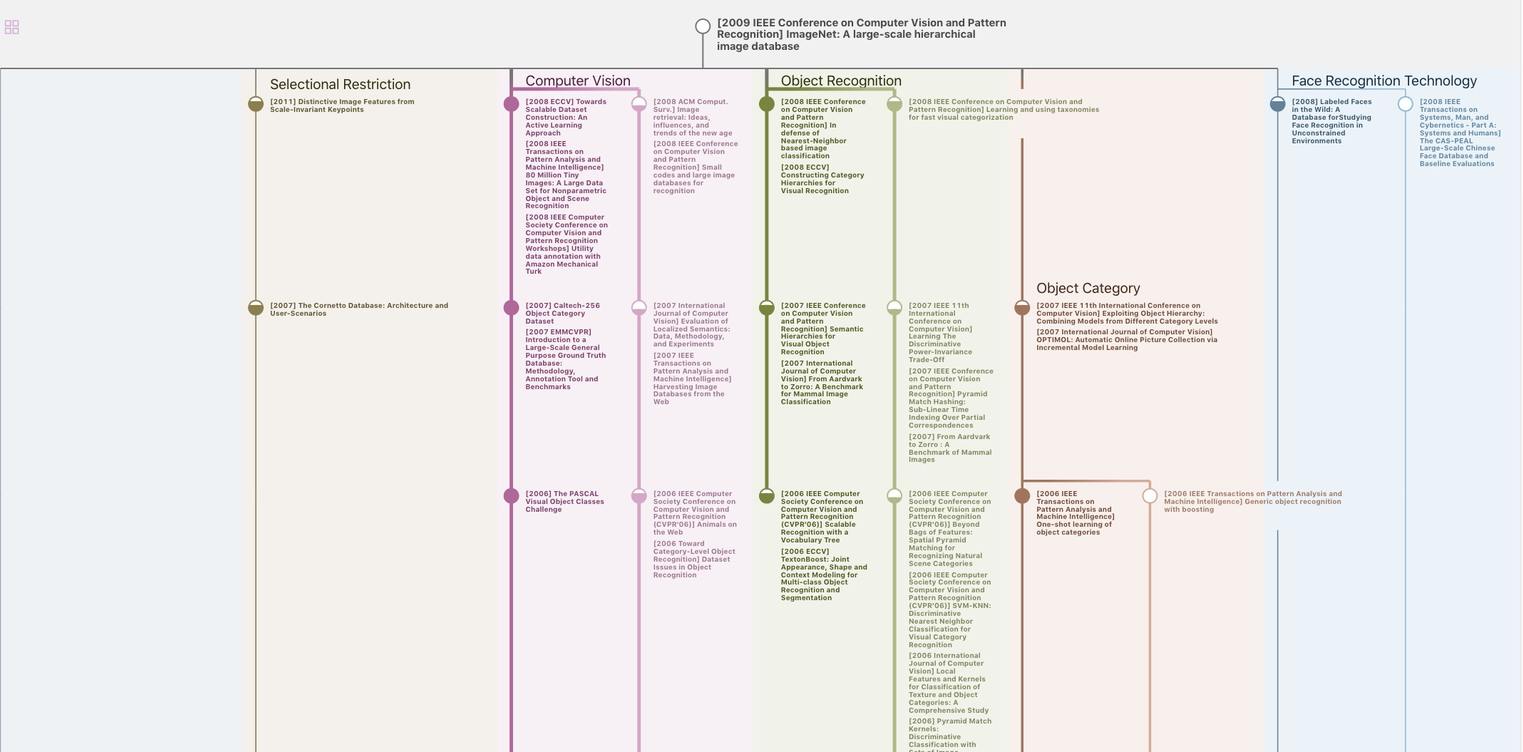
生成溯源树,研究论文发展脉络
Chat Paper
正在生成论文摘要