Evaluation of Abdominal CT Obtained Using a Deep Learning-Based Image Reconstruction Engine Compared with CT Using Adaptive Statistical Iterative Reconstruction
JOURNAL OF THE BELGIAN SOCIETY OF RADIOLOGY(2022)
摘要
Purpose: To compare the image quality of CT obtained using a deep learning-based image reconstruction (DLIR) engine with images with adaptive statistical iterative reconstruction-V (AV). Materials and Methods: Using a phantom, the noise power spectrum (NPS) and taskbased transfer function (TTF) were measured in images with different reconstructions (filtered back projection [FBP], AV30, 50, 100, DLIR-L, M, H) at multiple doses. One hundred and twenty abdominal CTs with 30% dose reduction were processed using AV30, AV50, DLIR-L, M, H. Objective and subjective analyses were performed. Results: The NPS peak of DLIR was lower than that of AV30 or AV50. Compared with AV30, the NPS average spatial frequencies were higher with DLIR-L or DLIR-M. For lower contrast objects, TTF in images with DLIR were higher than those with AV. The standard deviation in DLIR-H and DLIR-M was significantly lower than AV30 and AV50. The overall image quality was the best for DLIR-M (p < 0.001). Conclusions: DLIR showed improved image quality and decreased noise under a decreased radiation dose.
更多查看译文
关键词
deep learning-based image reconstruction, computed tomography, image quality
AI 理解论文
溯源树
样例
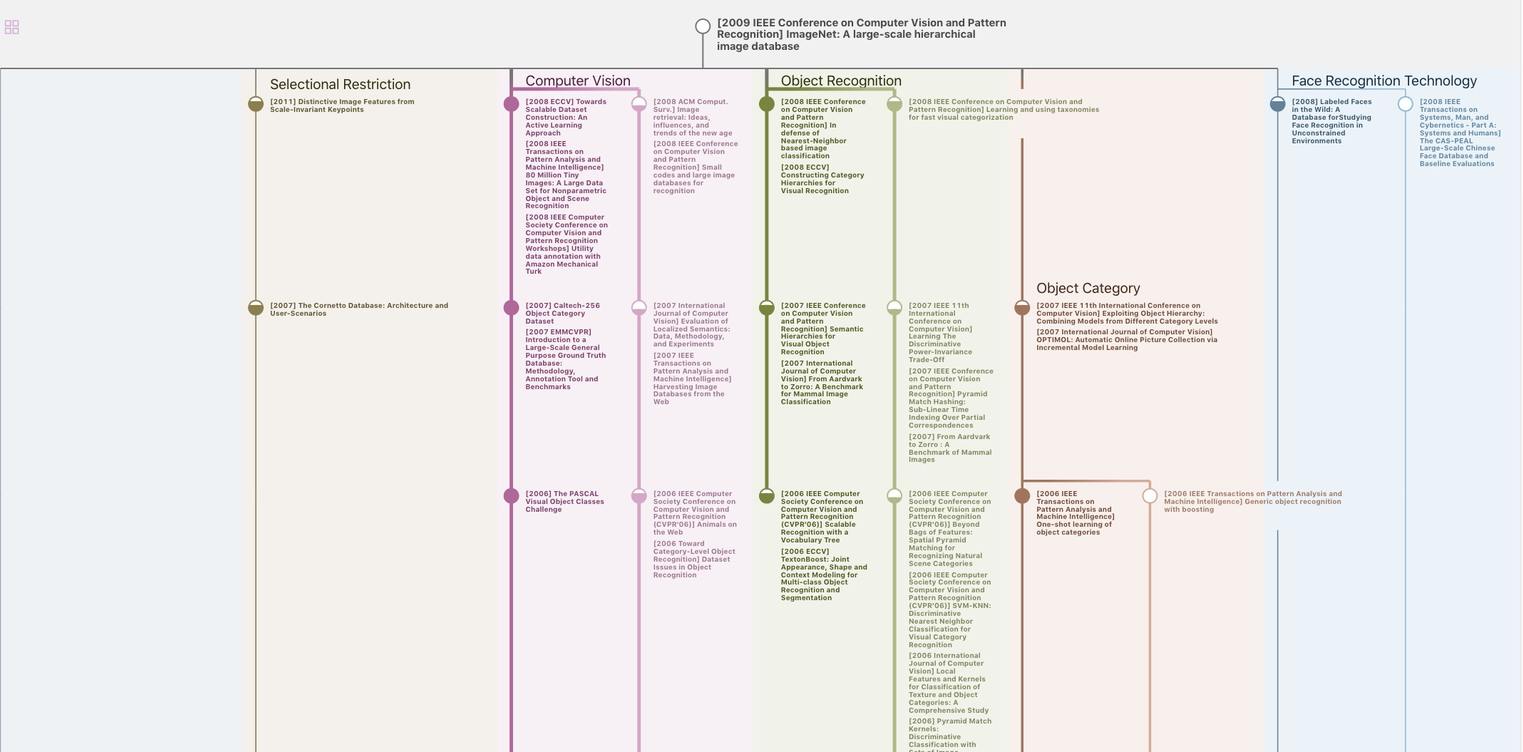
生成溯源树,研究论文发展脉络
Chat Paper
正在生成论文摘要