A Weakly Supervised Deep Learning Approach for Label-Free Imaging Flow-Cytometry-based Blood Diagnostics
Cell reports methods(2021)
摘要
The application of machine learning approaches to imaging flow cytometry (IFC) data has the potential to transform the diagnosis of hematological diseases. However, the need for manually labeled single-cell images for machine learning model training has severely limited its clinical application. To address this, we present iCellCnn, a weakly supervised deep learning approach for label-free IFC-based blood diagnostics. We demonstrate the capability of iCellCnn to achieve diagnosis of Se ' zary syndrome (SS) from patient samples on the basis of bright-field IFC images of T cells obtained after fluorescence-activated cell sorting of human peripheral blood mononuclear cell specimens. With a sample size of four healthy donors and five SS patients, iCellCnn achieved a 100% classification accuracy. As iCellCnn is not restricted to the diagnosis of SS, we expect such weakly supervised approaches to tap the diagnostic potential of IFC by providing automatic data-driven diagnosis of diseases with so-far unknown morphological manifestations.
更多查看译文
关键词
deep learning,machine learning,weakly supervised learning,image flow cytometry,cancer cell imaging,high-throughput imaging,peripheral blood mononuclear samples,Sézary syndrome
AI 理解论文
溯源树
样例
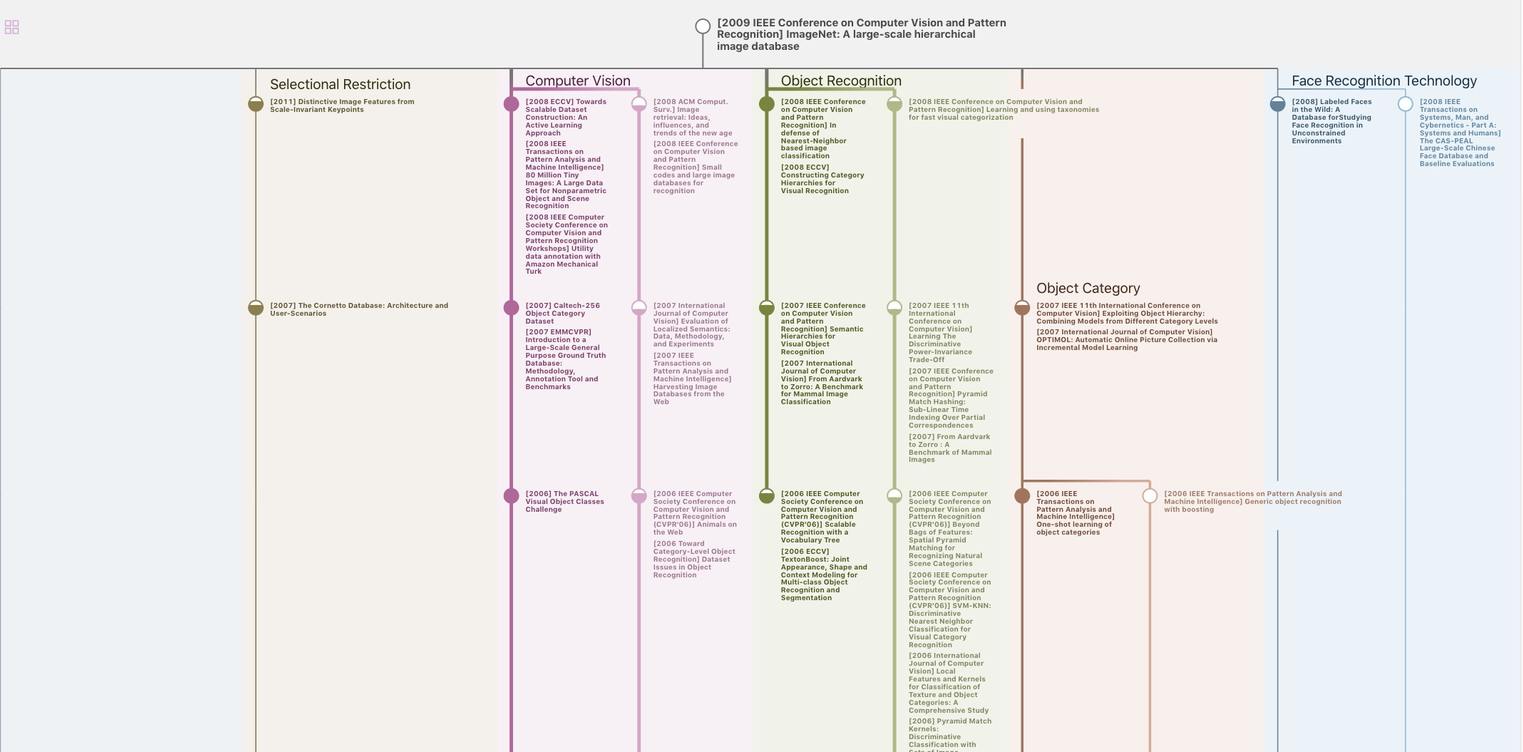
生成溯源树,研究论文发展脉络
Chat Paper
正在生成论文摘要