Improving Tracking Accuracy for Repetitive Learning Systems by High-Order Extended State Observers.
IEEE transactions on neural networks and learning systems(2022)
摘要
For systems executing repetitive tasks, how to realize the perfect tracking objective is generally desirable, for which an effective method called ``iterative learning control (ILC)'' emerges thanks to the incorporation of the repetitive execution of systems into an ILC design framework. However, nonrepetitive (iteration-varying) uncertainties are often inevitable in practice and greatly degrade the tracking accuracy of ILC, which has not been treated well, regardless of considerable robust ILC results. This motivates this article to develop a new design method to improve the tracking accuracy of ILC by adopting a high-order extended state observer (ESO) to address ill effects of nonrepetitive uncertainties and uncertain system models. With the designed ESO-based ILC, the robust tracking of any desired trajectory can be achieved such that the tracking error can be decreased to vary in a small bound depending continuously on the bounds of high-order variations of nonrepetitive uncertainties with respect to the iteration. It makes the tracking accuracy of ILC possible to be regulated through the design of ESO, of which the validity is demonstrated by including a simulation example.
更多查看译文
关键词
Uncertainty,Task analysis,Learning systems,Observers,Adaptive systems,Control systems,Uncertain systems,Extended state observer (ESO),iterative learning control (ILC),nonrepetitive uncertainty,repetitive learning system,robust tracking,tracking accuracy
AI 理解论文
溯源树
样例
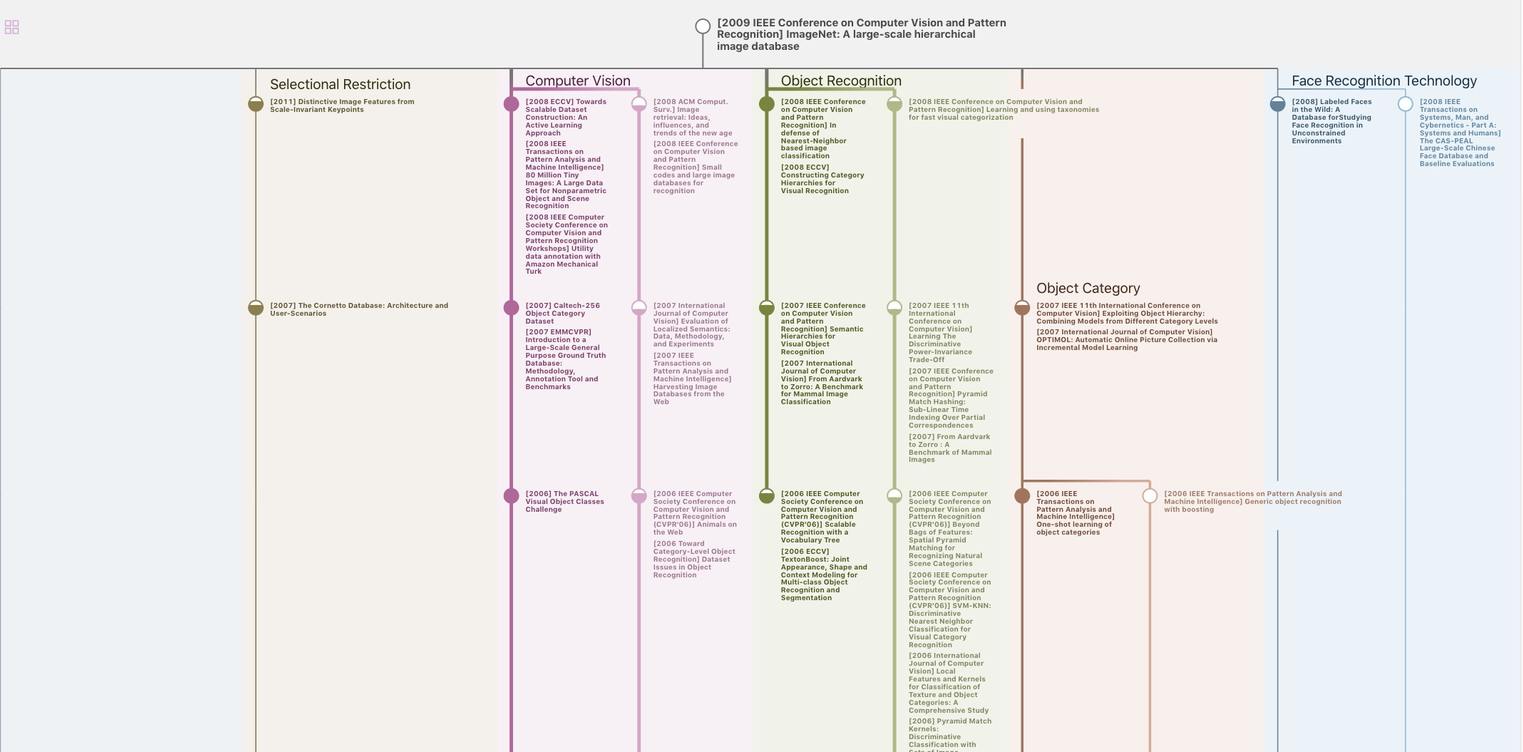
生成溯源树,研究论文发展脉络
Chat Paper
正在生成论文摘要