Equine radiograph classification using deep convolutional neural networks
arxiv(2022)
摘要
Purpose: To assess the capability of deep convolutional neural networks to classify anatomical location and projection from a series of 48 standard views of racehorse limbs. Materials and Methods: 9504 equine pre-import radiographs were used to train, validate, and test six deep learning architectures available as part of the open source machine learning framework PyTorch. Results: ResNet-34 achieved a top-1 accuracy of 0.8408 and the majority (88%) of misclassification was because of wrong laterality. Class activation maps indicated that joint morphology drove the model decision. Conclusion: Deep convolutional neural networks are capable of classifying equine pre-import radiographs into the 48 standard views including moderate discrimination of laterality independent of side marker presence.
更多查看译文
关键词
equine radiograph classification,neural
AI 理解论文
溯源树
样例
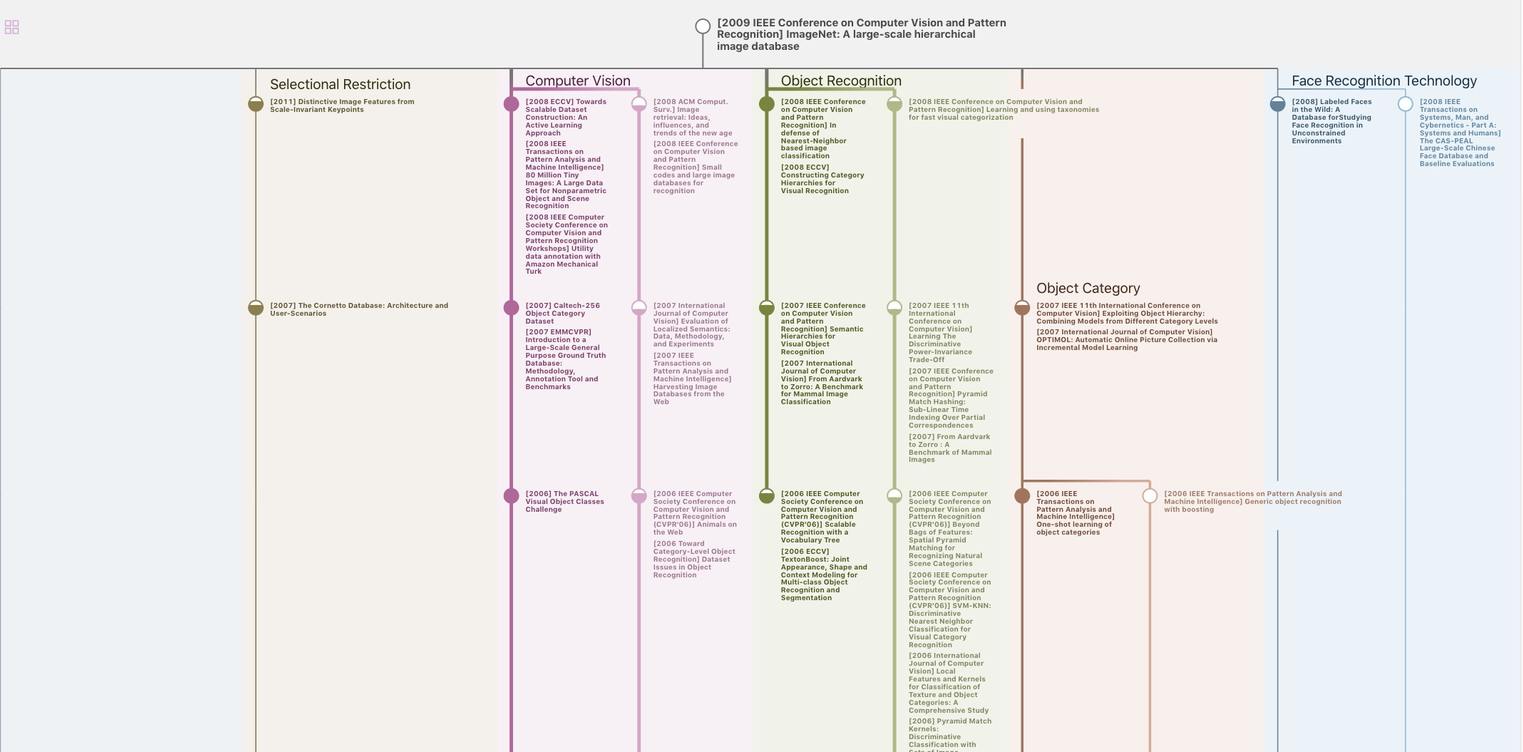
生成溯源树,研究论文发展脉络
Chat Paper
正在生成论文摘要