Privacy-Preserving Model Upgrades with Bidirectional Compatible Training in Image Retrieval
arxiv(2022)
摘要
The task of privacy-preserving model upgrades in image retrieval desires to reap the benefits of rapidly evolving new models without accessing the raw gallery images. A pioneering work introduced backward-compatible training, where the new model can be directly deployed in a backfill-free manner, i.e., the new query can be directly compared to the old gallery features. Despite a possible solution, its improvement in sequential model upgrades is gradually limited by the fixed and under-quality old gallery embeddings. To this end, we propose a new model upgrade paradigm, termed Bidirectional Compatible Training (BiCT), which will upgrade the old gallery embeddings by forward-compatible training towards the embedding space of the backward-compatible new model. We conduct comprehensive experiments to verify the prominent improvement by BiCT and interestingly observe that the inconspicuous loss weight of backward compatibility actually plays an essential role for both backward and forward retrieval performance. To summarize, we introduce a new and valuable problem named privacy-preserving model upgrades, with a proper solution BiCT. Several intriguing insights are further proposed to get the most out of our method.
更多查看译文
关键词
bidirectional compatible training
AI 理解论文
溯源树
样例
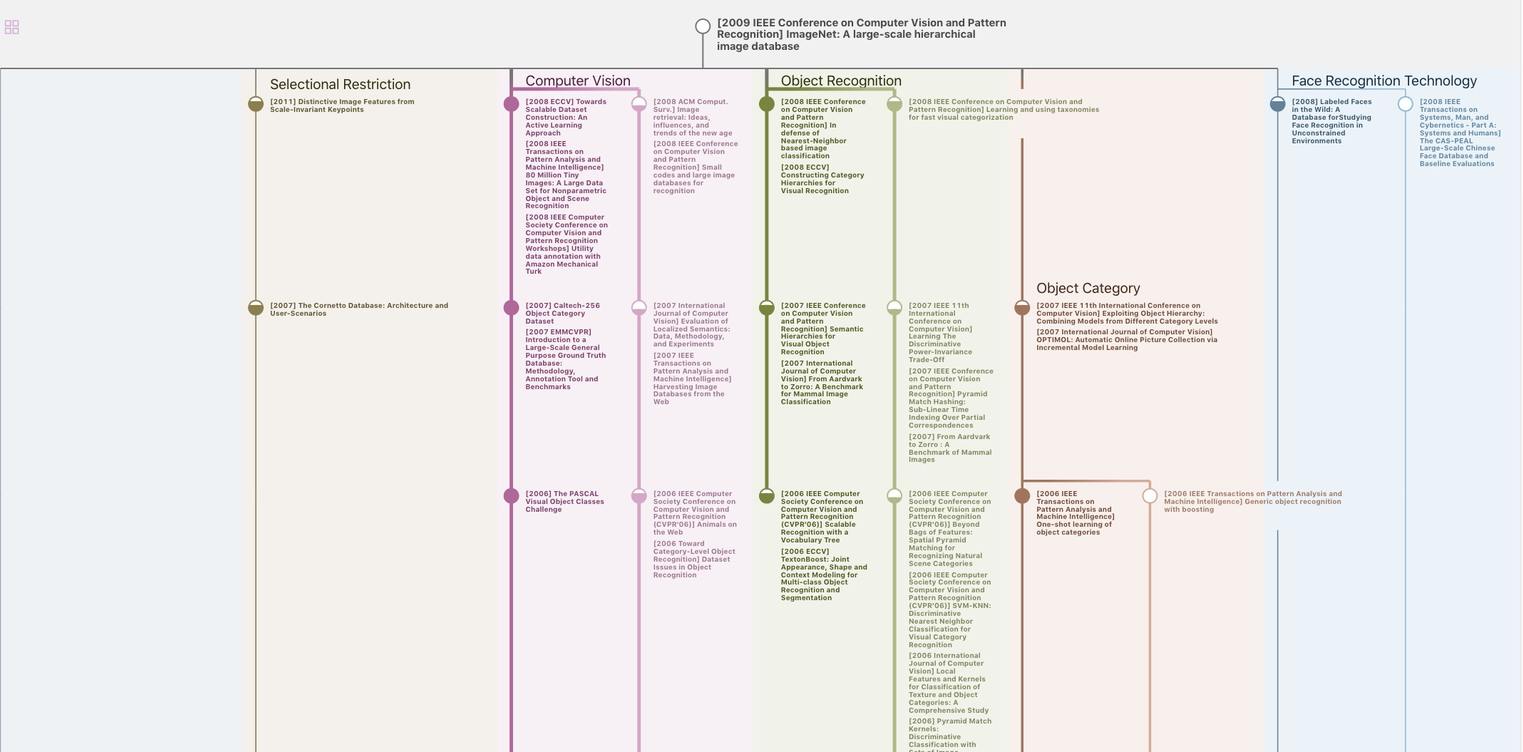
生成溯源树,研究论文发展脉络
Chat Paper
正在生成论文摘要