Designing high- T C superconductors with BCS-inspired screening, density functional theory, and deep-learning
arxiv(2022)
摘要
We develop a multi-step workflow for the discovery of conventional superconductors, starting with a Bardeen–Cooper–Schrieffer inspired pre-screening of 1736 materials with high Debye temperature and electronic density of states. Next, we perform electron-phonon coupling calculations for 1058 of them to establish a large and systematic database of BCS superconducting properties. Using the McMillan-Allen-Dynes formula, we identify 105 dynamically stable materials with transition temperatures, T C ≥ 5 K. Additionally, we analyze trends in our dataset and individual materials including MoN, VC, VTe, KB 6 , Ru 3 NbC, V 3 Pt, ScN, LaN 2 , RuO 2 , and TaC. We demonstrate that deep-learning(DL) models can predict superconductor properties faster than direct first-principles computations. Notably, we find that by predicting the Eliashberg function as an intermediate quantity, we can improve model performance versus a direct DL prediction of T C . We apply the trained models on the crystallographic open database and pre-screen candidates for further DFT calculations.
更多查看译文
关键词
superconductors,bcs-inspired,deep-learning
AI 理解论文
溯源树
样例
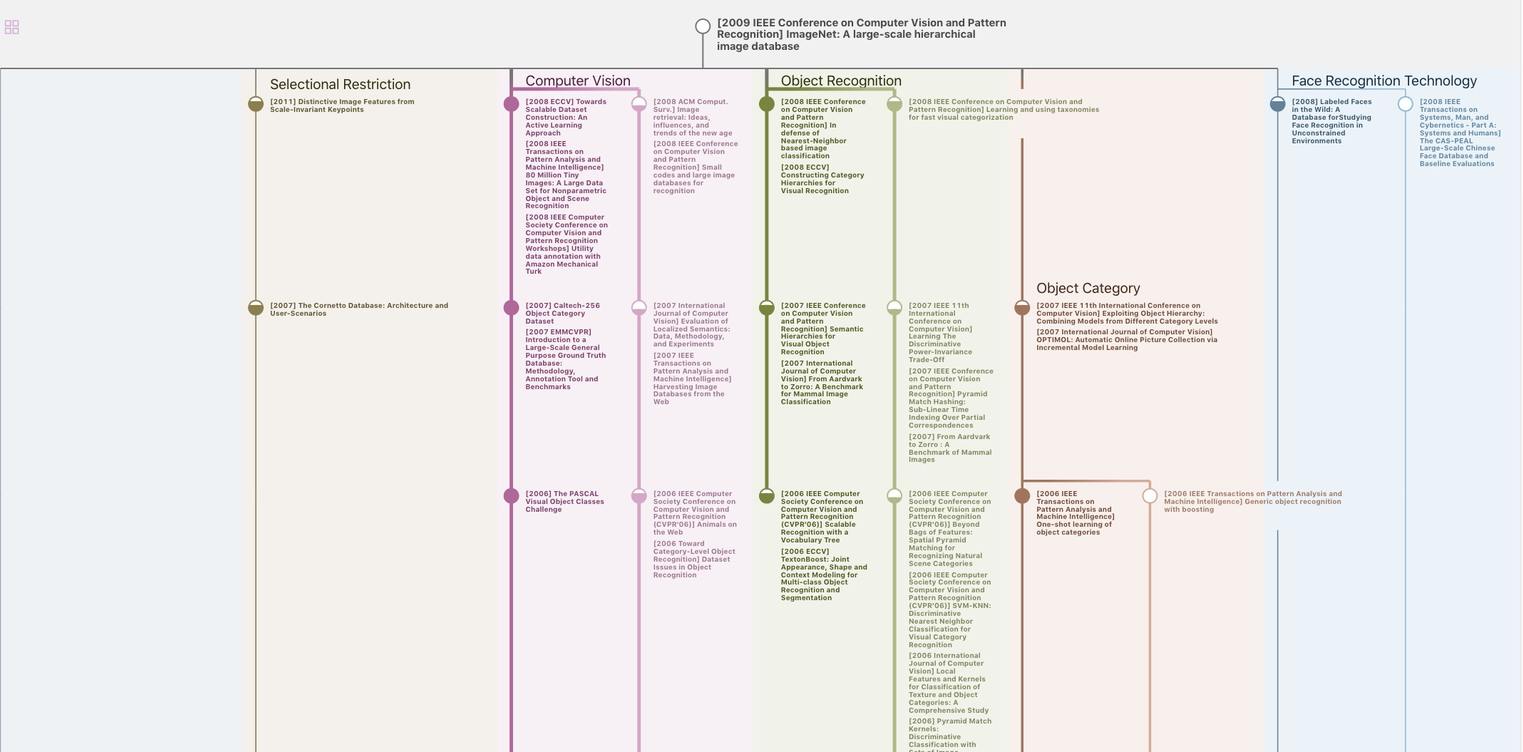
生成溯源树,研究论文发展脉络
Chat Paper
正在生成论文摘要