Elucidating Meta-Structures of Noisy Labels in Semantic Segmentation by Deep Neural Networks
arxiv(2022)
摘要
Supervised training of deep neural networks (DNNs) by noisy labels has been studied extensively in image classification but much less in image segmentation. Our understanding of the learning behavior of DNNs trained by noisy segmentation labels remains limited. We address this deficiency in both binary segmentation of biological microscopy images and multi-class segmentation of natural images. We classify segmentation labels according to their noise transition matrices (NTMs) and compare performance of DNNs trained by different types of labels. When we randomly sample a small fraction (e.g., 10%) or flip a large fraction (e.g., 90%) of the ground-truth labels to train DNNs, their segmentation performance remains largely unchanged. This indicates that DNNs learn structures hidden in labels rather than pixel-level labels per se in their supervised training for semantic segmentation. We call these hidden structures meta-structures. When labels with different perturbations to the meta-structures are used to train DNNs, their performance in feature extraction and segmentation degrades consistently. In contrast, addition of meta-structure information substantially improves performance of an unsupervised model in binary semantic segmentation. We formulate meta-structures mathematically as spatial density distributions. We show theoretically and experimentally how this formulation explains key observed learning behavior of DNNs.
更多查看译文
关键词
noisy labels,semantic segmentation,deep neural networks,neural networks,meta-structures
AI 理解论文
溯源树
样例
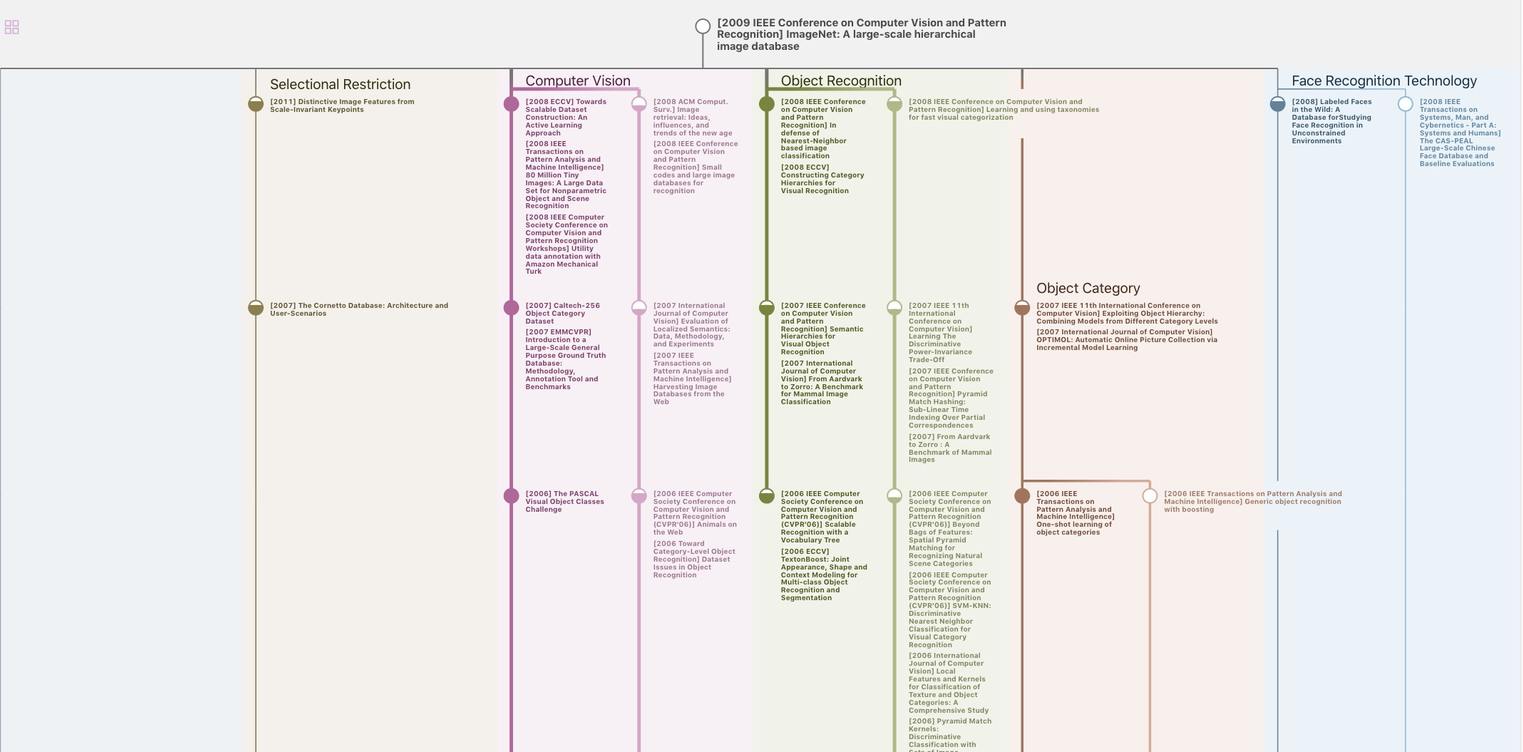
生成溯源树,研究论文发展脉络
Chat Paper
正在生成论文摘要