Deep Learning-Enabled Semantic Communication Systems With Task-Unaware Transmitter and Dynamic Data
IEEE Journal on Selected Areas in Communications(2023)
摘要
Existing deep learning-enabled semantic communication systems often rely on shared background knowledge between the transmitter and receiver that includes empirical data and their associated semantic information. In practice, the semantic information is defined by the pragmatic task of the receiver and cannot be known to the transmitter. The actual observable data at the transmitter can also have non-identical distribution with the empirical data in the shared background knowledge library. To address these practical issues, this paper proposes a new neural network-based semantic communication system for image transmission, where the task is unaware at the transmitter and the data environment is dynamic. The system consists of two main parts, namely the semantic coding (SC) network and the data adaptation (DA) network. The SC network learns how to extract and transmit the semantic information using a receiver-leading training process. By using the domain adaptation technique from transfer learning, the DA network learns how to convert the data observed into a similar form of the empirical data that the SC network can process without re-training. Numerical experiments show that the proposed method can be adaptive to observable datasets while keeping high performance in terms of both data recovery and task execution.
更多查看译文
关键词
Task-unaware semantic communication,semantic coding,domain adaptation
AI 理解论文
溯源树
样例
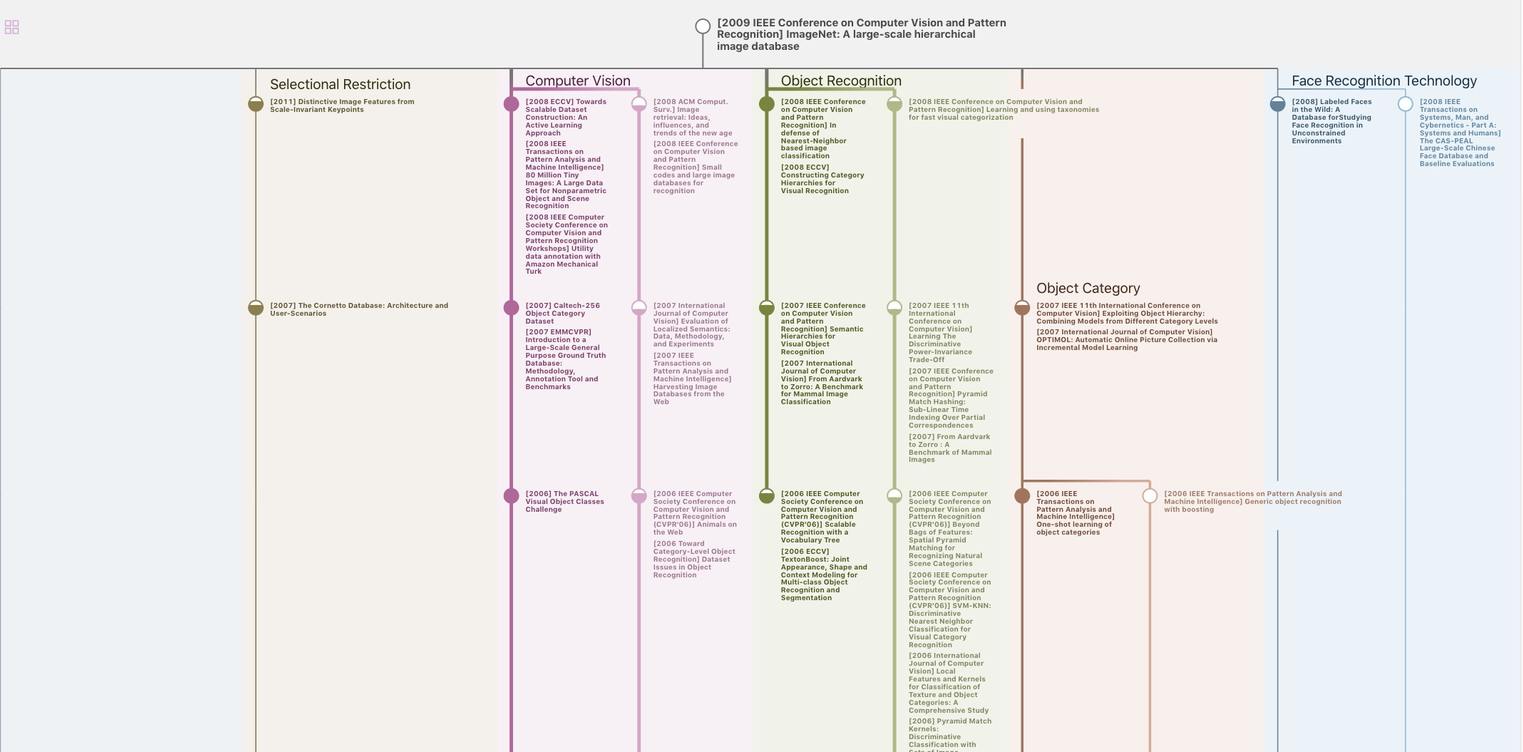
生成溯源树,研究论文发展脉络
Chat Paper
正在生成论文摘要