Type-aware Embeddings for Multi-Hop Reasoning over Knowledge Graphs.
European Conference on Artificial Intelligence(2022)
摘要
Multi-hop reasoning over real-life knowledge graphs (KGs) is a highly challenging problem as traditional subgraph matching methods are not capable to deal with noise and missing information. To address this problem, it has been recently introduced a promising approach based on jointly embedding logical queries and KGs into a low-dimensional space to identify answer entities. However, existing proposals ignore critical semantic knowledge inherently available in KGs, such as type information. To leverage type information, we propose a novel TypE-aware Message Passing (TEMP) model, which enhances the entity and relation representations in queries, and simultaneously improves generalization, deductive and inductive reasoning. Remarkably, TEMP is a plug-and-play model that can be easily incorporated into existing embedding-based models to improve their performance. Extensive experiments on three real-world datasets demonstrate TEMP's effectiveness.
更多查看译文
关键词
Machine Learning: Knowledge Aided Learning,Knowledge Representation and Reasoning: Reasoning about Knowledge and Belief
AI 理解论文
溯源树
样例
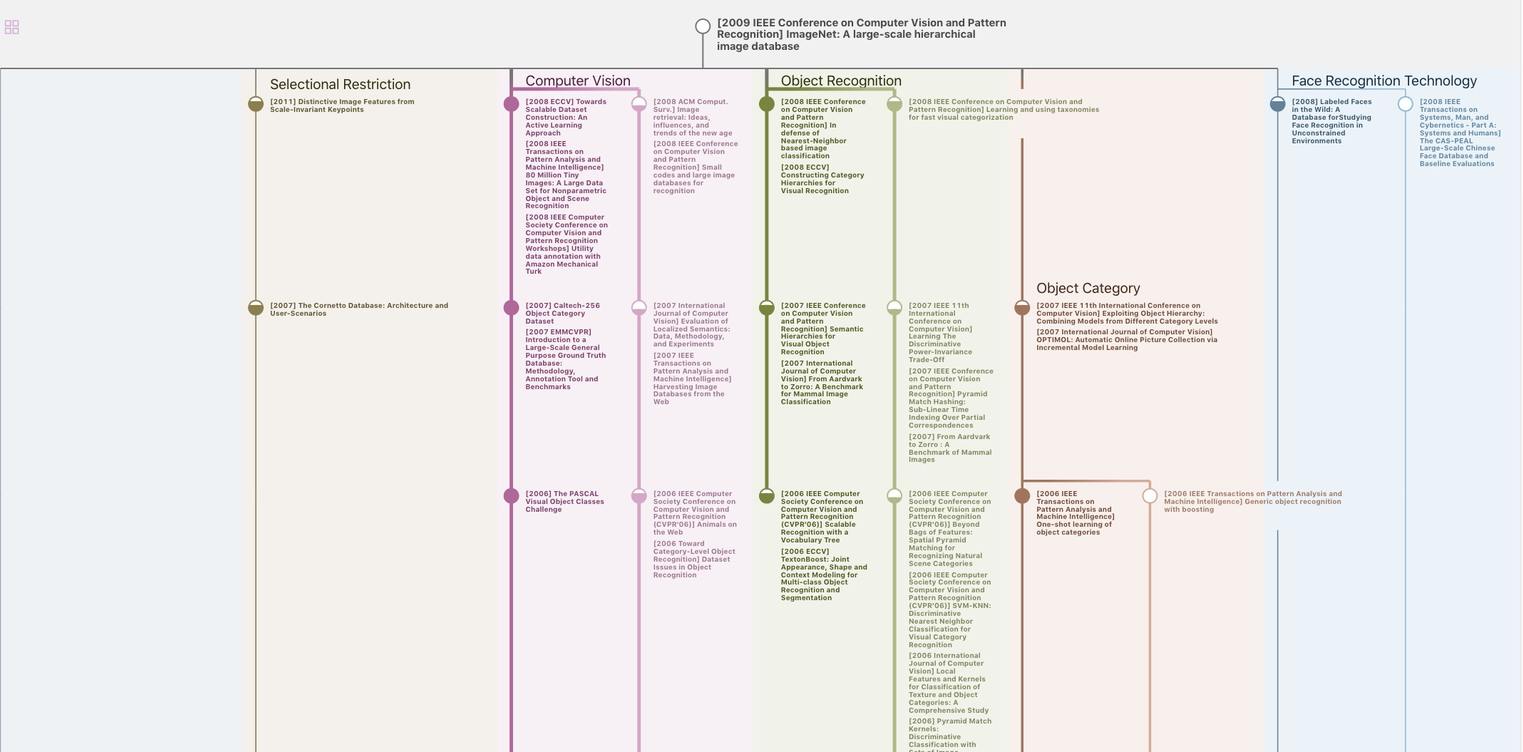
生成溯源树,研究论文发展脉络
Chat Paper
正在生成论文摘要