Cross-Domain Correlation Distillation for Unsupervised Domain Adaptation in Nighttime Semantic Segmentation
IEEE Conference on Computer Vision and Pattern Recognition(2022)
摘要
The performance of nighttime semantic segmentation is restricted by the poor illumination and a lack of pixel-wise annotation, which severely limit its application in autonomous driving. Existing works, e.g., using the twilight as the intermediate target domain to perform the adaptation from daytime to nighttime, may fail to cope with the inherent difference between datasets caused by the camera equipment and the urban style. Faced with these two types of domain shifts, i.e., the illumination and the inherent difference of the datasets, we propose a novel domain adaptation framework via cross-domain correlation distillation, called CCDistill. The invariance of illumination or inherent difference between two images is fully explored so as to make up for the lack of labels for nighttime images. Specifically, we extract the content and style knowledge contained in features, calculate the degree of inherent or illumination difference between two images. The domain adaptation is achieved using the invariance of the same kind of difference. Extensive experiments on Dark Zurich and ACDC demon-strate that CCDistill achieves the state-of-the-art performance for nighttime semantic segmentation. Notably, our method is a one-stage domain adaptation network which can avoid affecting the inference time. Our implementation is available at https://github.com/ghuan99/CCDistill.
更多查看译文
关键词
grouping and shape analysis, Transfer/low-shot/long-tail learning,Self-& semi-& meta- Segmentation
AI 理解论文
溯源树
样例
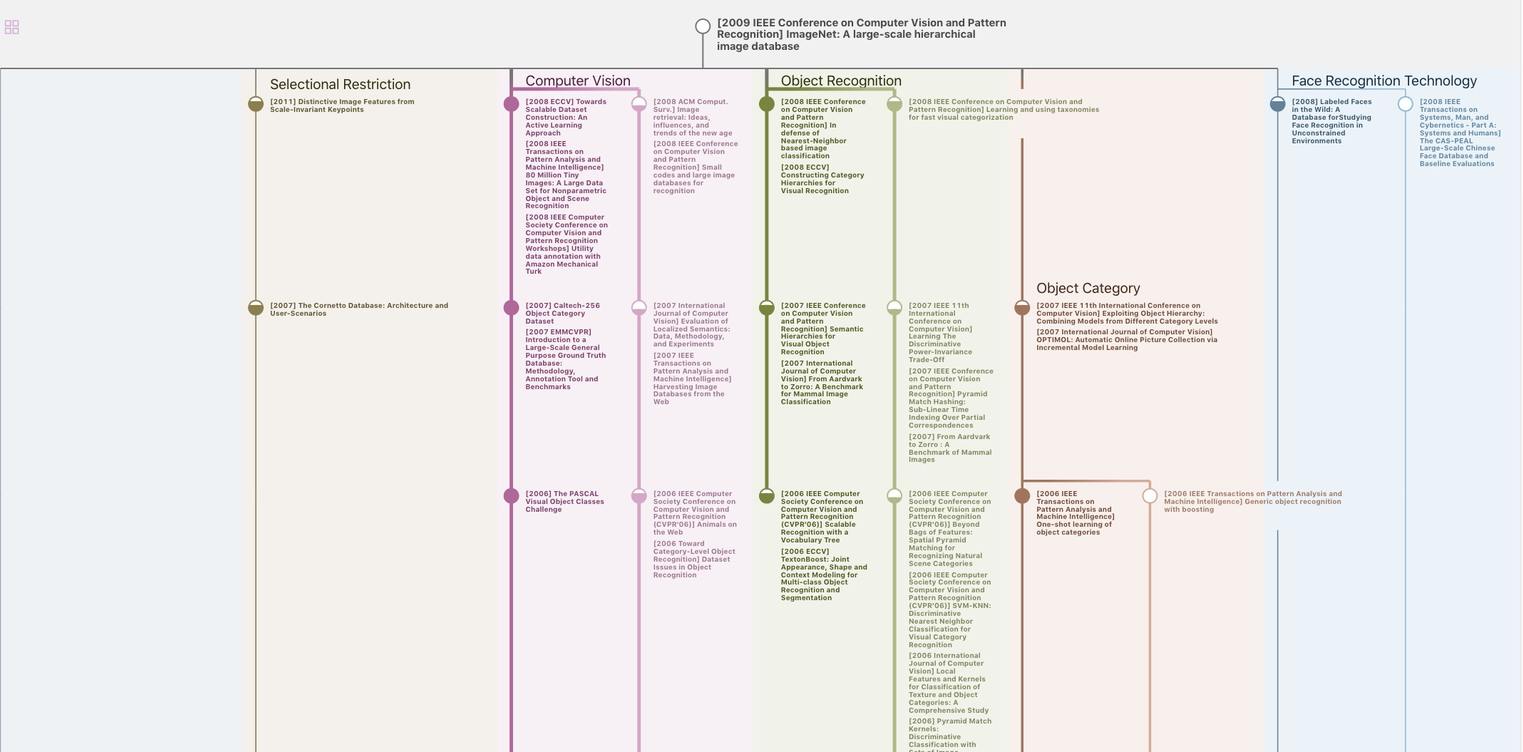
生成溯源树,研究论文发展脉络
Chat Paper
正在生成论文摘要