SELC: Self-Ensemble Label Correction Improves Learning with Noisy Labels
European Conference on Artificial Intelligence(2022)
摘要
Deep neural networks are prone to overfitting noisy labels, resulting in poor generalization performance. To overcome this problem, we present a simple and effective method self-ensemble label correction (SELC) to progressively correct noisy labels and refine the model. We look deeper into the memorization behavior in training with noisy labels and observe that the network outputs are reliable in the early stage. To retain this reliable knowledge, SELC uses ensemble predictions formed by an exponential moving average of network outputs to update the original noisy labels. We show that training with SELC refines the model by gradually reducing supervision from noisy labels and increasing supervision from ensemble predictions. Despite its simplicity, compared with many state-of-the-art methods, SELC obtains more promising and stable results in the presence of class-conditional, instance-dependent, and real-world label noise. The code is available at https://github.com/MacLLL/SELC.
更多查看译文
关键词
Machine Learning: Weakly Supervised Learning,Machine Learning: Classification,Machine Learning: Ensemble Methods
AI 理解论文
溯源树
样例
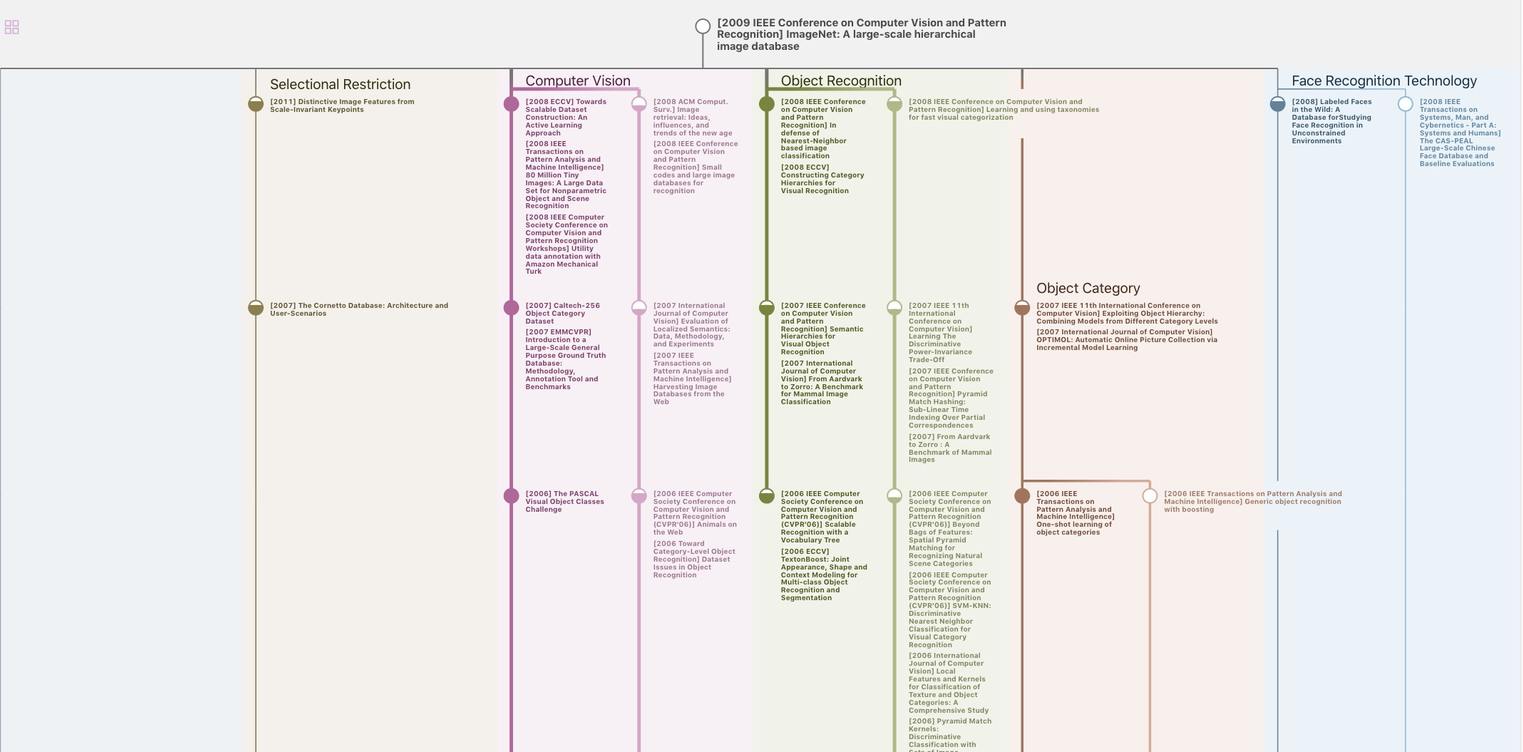
生成溯源树,研究论文发展脉络
Chat Paper
正在生成论文摘要