Evaluation of Proximal Policy Optimization with Extensions in Virtual Environments of Various Complexity
International Conference Radioelektronika(2022)
摘要
This paper presents an evaluation of the Proximal Policy Optimization deep reinforcement learning algorithm alone and with several extensions. The extensions used are imitation learning and intrinsic curiosity module. The evaluation takes place in a custom-made racing game, where the goal of an agent, a car, is to complete a racing circuit. The game is implemented in the Unity game engine and the machine learning algorithms are provided by the ML-Agents toolkit. The game provides three levels with racing circuits of different complexity. The results described focus on the hyperparameter search, training progress for all the combinations of the algorithm and the extensions and evaluation in the inference mode.
更多查看译文
关键词
reinforcement learning,Proximal Policy Optimization,evaluation,virtual environment,Unity,ML-Agents
AI 理解论文
溯源树
样例
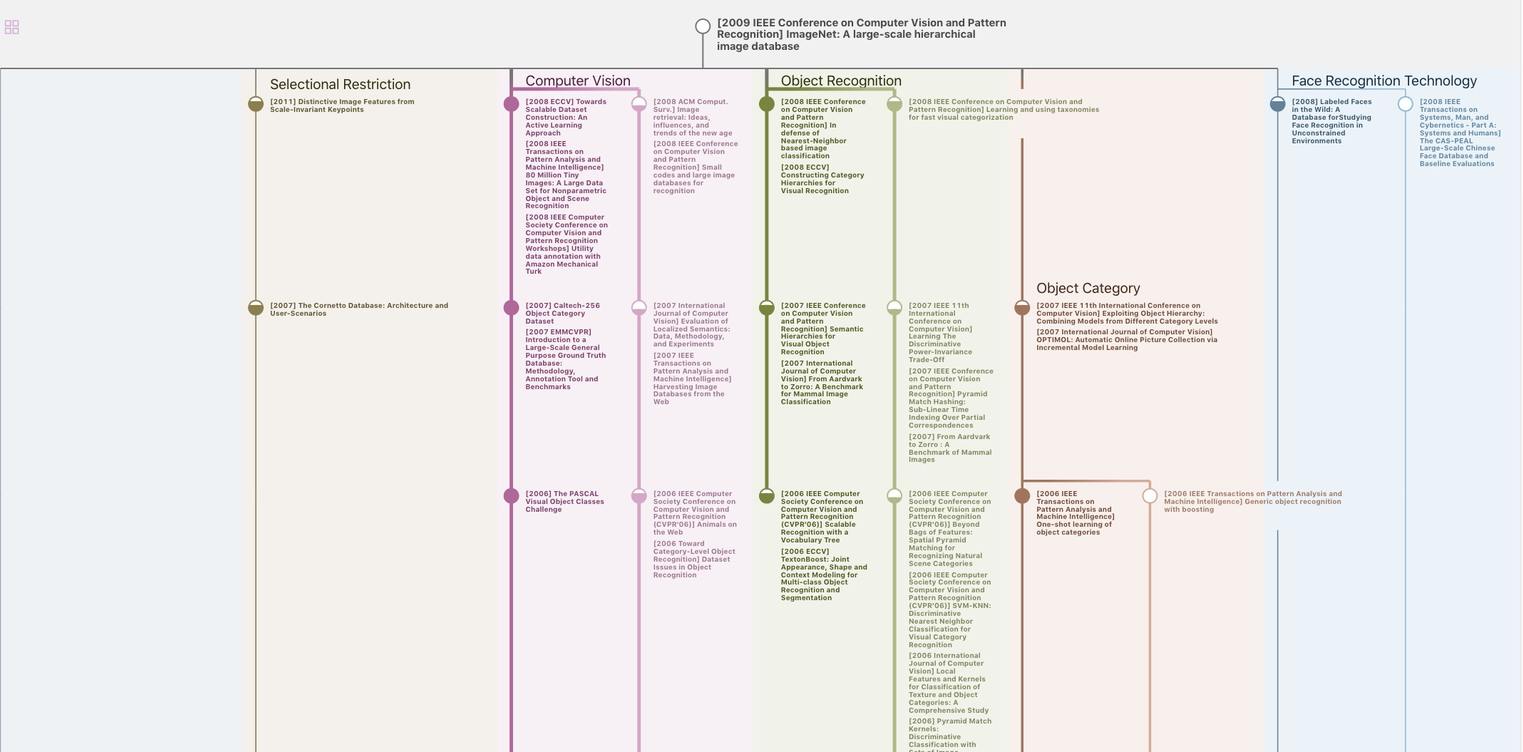
生成溯源树,研究论文发展脉络
Chat Paper
正在生成论文摘要