Subverting Fair Image Search with Generative Adversarial Perturbations.
ACM Conference on Fairness, Accountability and Transparency (FAccT)(2022)
摘要
In this work we explore the intersection fairness and robustness in the context of ranking: \textit{when a ranking model has been calibrated to achieve some definition of fairness, is it possible for an external adversary to make the ranking model behave unfairly without having access to the model or training data?} To investigate this question, we present a case study in which we develop and then attack a state-of-the-art, fairness-aware image search engine using images that have been maliciously modified using a Generative Adversarial Perturbation (GAP) model. These perturbations attempt to cause the fair re-ranking algorithm to unfairly boost the rank of images containing people from an adversary-selected subpopulation. We present results from extensive experiments demonstrating that our attacks can successfully confer significant unfair advantage to people from the majority class relative to fairly-ranked baseline search results. We demonstrate that our attacks are robust across a number of variables, that they have close to zero impact on the relevance of search results, and that they succeed under a strict threat model. Our findings highlight the danger of deploying fair machine learning algorithms in-the-wild when (1) the data necessary to achieve fairness may be adversarially manipulated, and (2) the models themselves are not robust against attacks.
更多查看译文
AI 理解论文
溯源树
样例
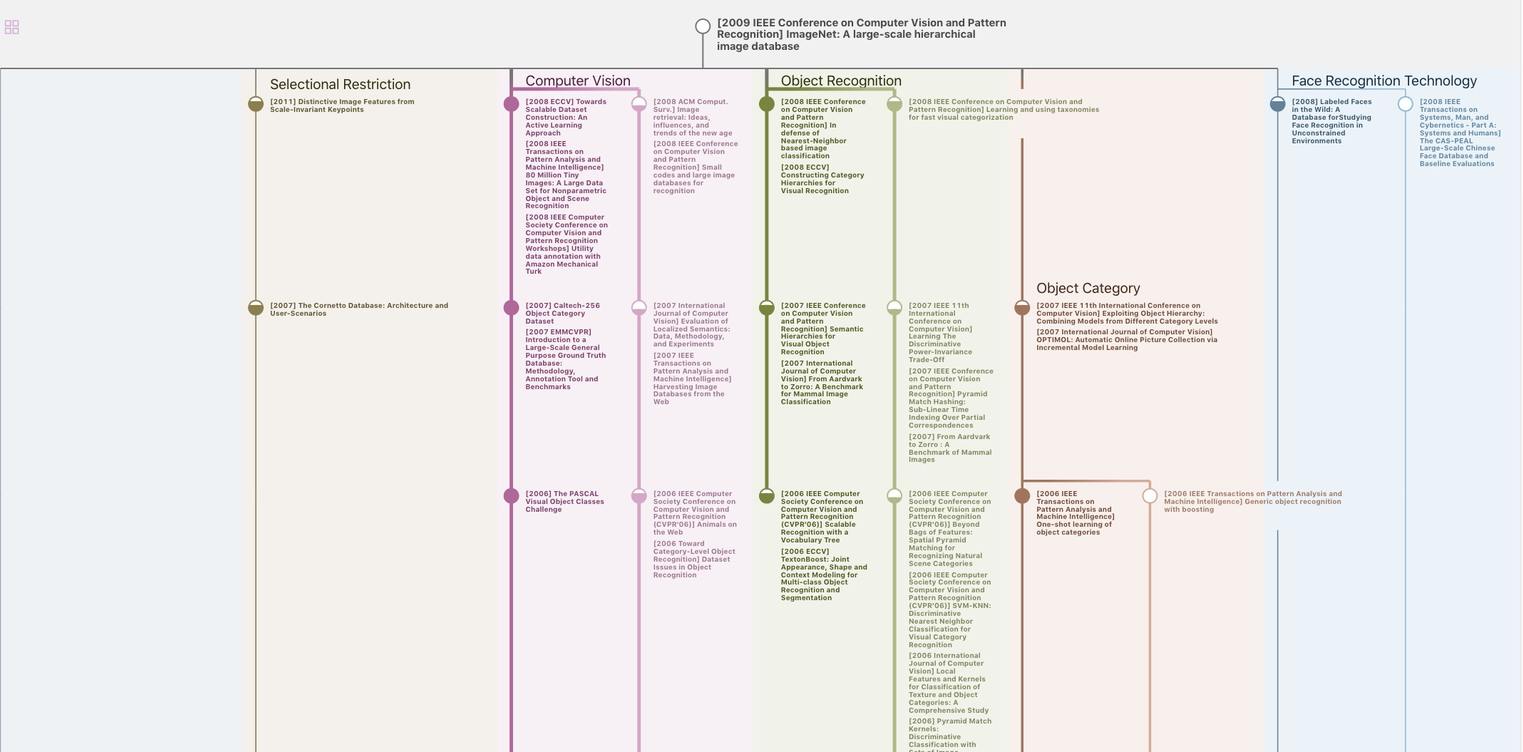
生成溯源树,研究论文发展脉络
Chat Paper
正在生成论文摘要