GANimator: neural motion synthesis from a single sequence
ACM Transactions on Graphics(2022)
摘要
We present GANimator, a generative model that learns to synthesize novel motions from a single, short motion sequence. GANimator generates motions that resemble the core elements of the original motion, while simultaneously synthesizing novel and diverse movements. Existing data-driven techniques for motion synthesis require a large motion dataset which contains the desired and specific skeletal structure. By contrast, GANimator only requires training on a single motion sequence, enabling novel motion synthesis for a variety of skeletal structures e.g., bipeds, quadropeds, hexapeds, and more. Our framework contains a series of generative and adversarial neural networks, each responsible for generating motions in a specific frame rate. The framework progressively learns to synthesize motion from random noise, enabling hierarchical control over the generated motion content across varying levels of detail. We show a number of applications, including crowd simulation, key-frame editing, style transfer, and interactive control, which all learn from a single input sequence. Code and data for this paper are at https://peizhuoli.github.io/ganimator.
更多查看译文
关键词
neural motion processing, motion synthesis
AI 理解论文
溯源树
样例
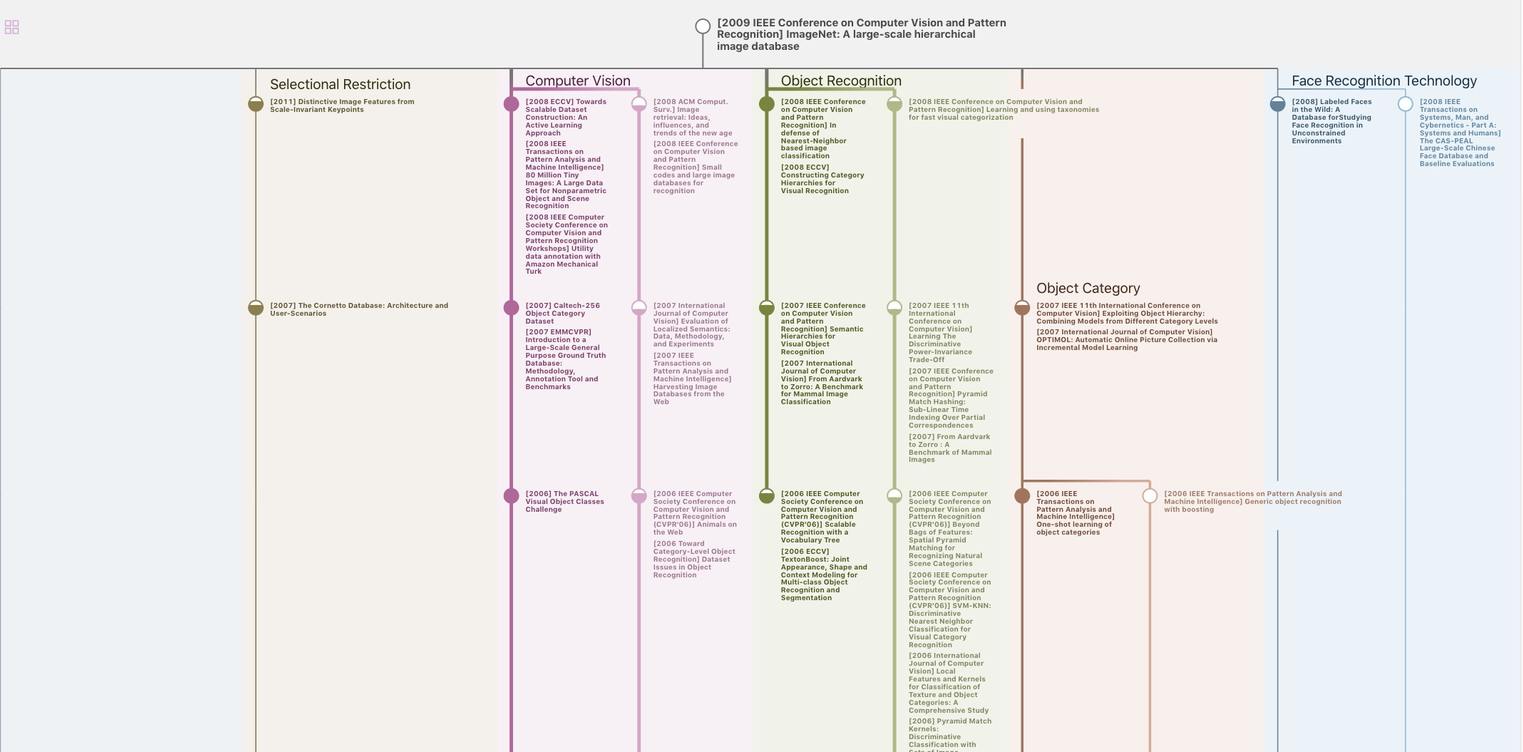
生成溯源树,研究论文发展脉络
Chat Paper
正在生成论文摘要