An Empirical Study on Activity Recognition in Long Surgical Videos
Computing Research Repository (CoRR)(2022)
Intuit Surg | Tubi
Abstract
Activity recognition in surgical videos is a key research area for developing next-generation devices and workflow monitoring systems. Since surgeries are long processes with highly-variable lengths, deep learning models used for surgical videos often consist of a two-stage setup using a backbone and temporal sequence model. In this paper, we investigate many state-of-the-art backbones and temporal models to find architectures that yield the strongest performance for surgical activity recognition. We first benchmark the models performance on a large-scale activity recognition dataset containing over 800 surgery videos captured in multiple clinical operating rooms. We further evaluate the models on the two smaller public datasets, the Cholec80 and Cataract-101 datasets, containing only 80 and 101 videos respectively. We empirically found that Swin-Transformer+BiGRU temporal model yielded strong performance on both datasets. Finally, we investigate the adaptability of the model to new domains by fine-tuning models to a new hospital and experimenting with a recent unsupervised domain adaptation approach.
MoreTranslated text
Key words
Surgical Activity Recognition,Transformers,3D ConvNets
PDF
View via Publisher
AI Read Science
AI Summary
AI Summary is the key point extracted automatically understanding the full text of the paper, including the background, methods, results, conclusions, icons and other key content, so that you can get the outline of the paper at a glance.
Example
Background
Key content
Introduction
Methods
Results
Related work
Fund
Key content
- Pretraining has recently greatly promoted the development of natural language processing (NLP)
- We show that M6 outperforms the baselines in multimodal downstream tasks, and the large M6 with 10 parameters can reach a better performance
- We propose a method called M6 that is able to process information of multiple modalities and perform both single-modal and cross-modal understanding and generation
- The model is scaled to large model with 10 billion parameters with sophisticated deployment, and the 10 -parameter M6-large is the largest pretrained model in Chinese
- Experimental results show that our proposed M6 outperforms the baseline in a number of downstream tasks concerning both single modality and multiple modalities We will continue the pretraining of extremely large models by increasing data to explore the limit of its performance
Try using models to generate summary,it takes about 60s
Must-Reading Tree
Example
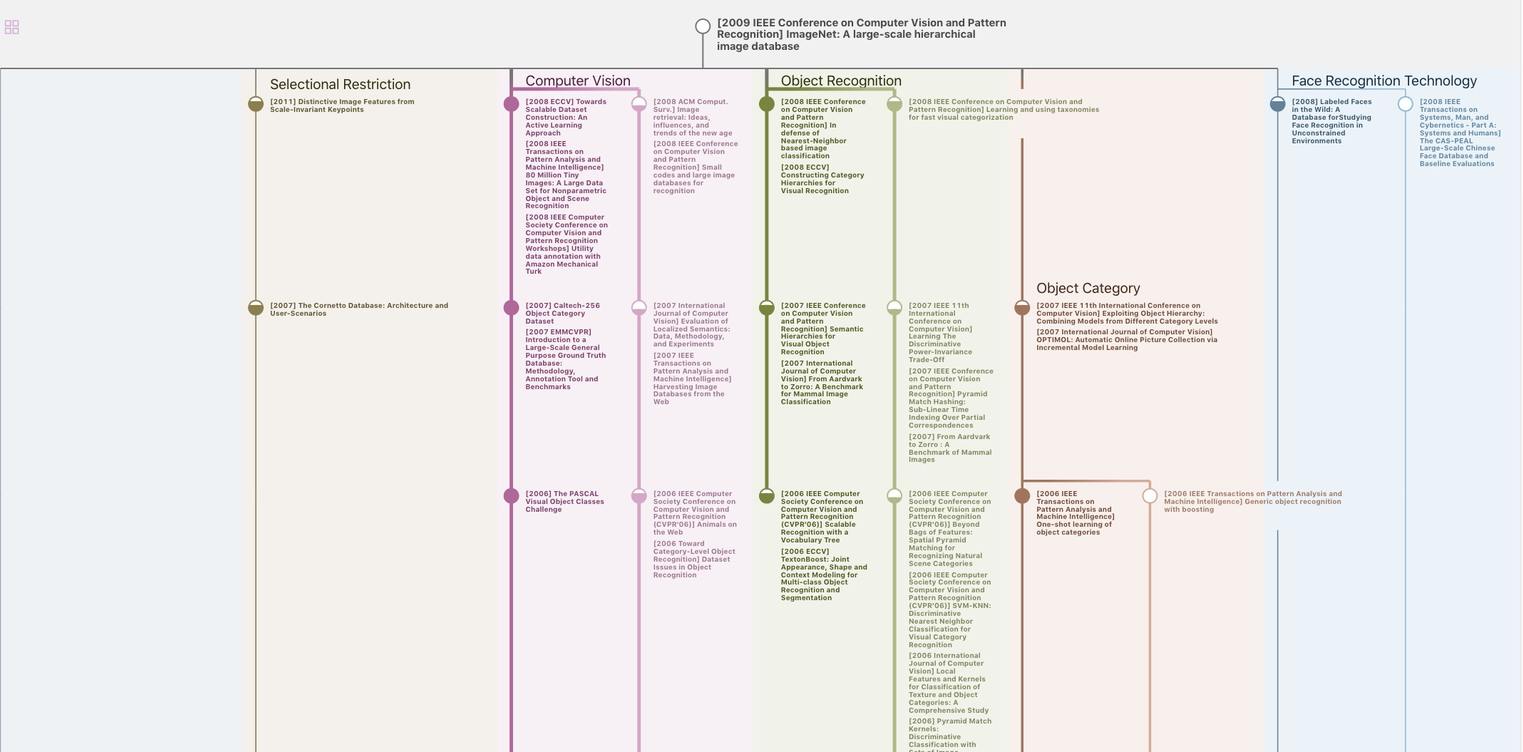
Generate MRT to find the research sequence of this paper
Related Papers
2018
被引用28 | 浏览
2018
被引用45 | 浏览
Data Disclaimer
The page data are from open Internet sources, cooperative publishers and automatic analysis results through AI technology. We do not make any commitments and guarantees for the validity, accuracy, correctness, reliability, completeness and timeliness of the page data. If you have any questions, please contact us by email: report@aminer.cn
Chat Paper
GPU is busy, summary generation fails
Rerequest