FairGAN: GANs-based Fairness-aware Learning for Recommendations with Implicit Feedback
International World Wide Web Conference(2022)
摘要
ABSTRACT Ranking algorithms in recommender systems influence people to make decisions. Conventional ranking algorithms based on implicit feedback data aim to maximize the utility to users by capturing users’ preferences over items. However, these utility-focused algorithms tend to cause fairness issues that require careful consideration in online platforms. Existing fairness-focused studies does not explicitly consider the problem of lacking negative feedback in implicit feedback data, while previous utility-focused methods ignore the importance of fairness in recommendations. To fill this gap, we propose a Generative Adversarial Networks (GANs) based learning algorithm FairGAN mapping the exposure fairness issue to the problem of negative preferences in implicit feedback data. FairGAN does not explicitly treat unobserved interactions as negative, but instead, adopts a novel fairness-aware learning strategy to dynamically generate fairness signals. This optimizes the search direction to make FairGAN capable of searching the space of the optimal ranking that can fairly allocate exposure to individual items while preserving users’ utilities as high as possible.
更多查看译文
关键词
Fairness, Ranking, Exposure, GANs, Recommendation
AI 理解论文
溯源树
样例
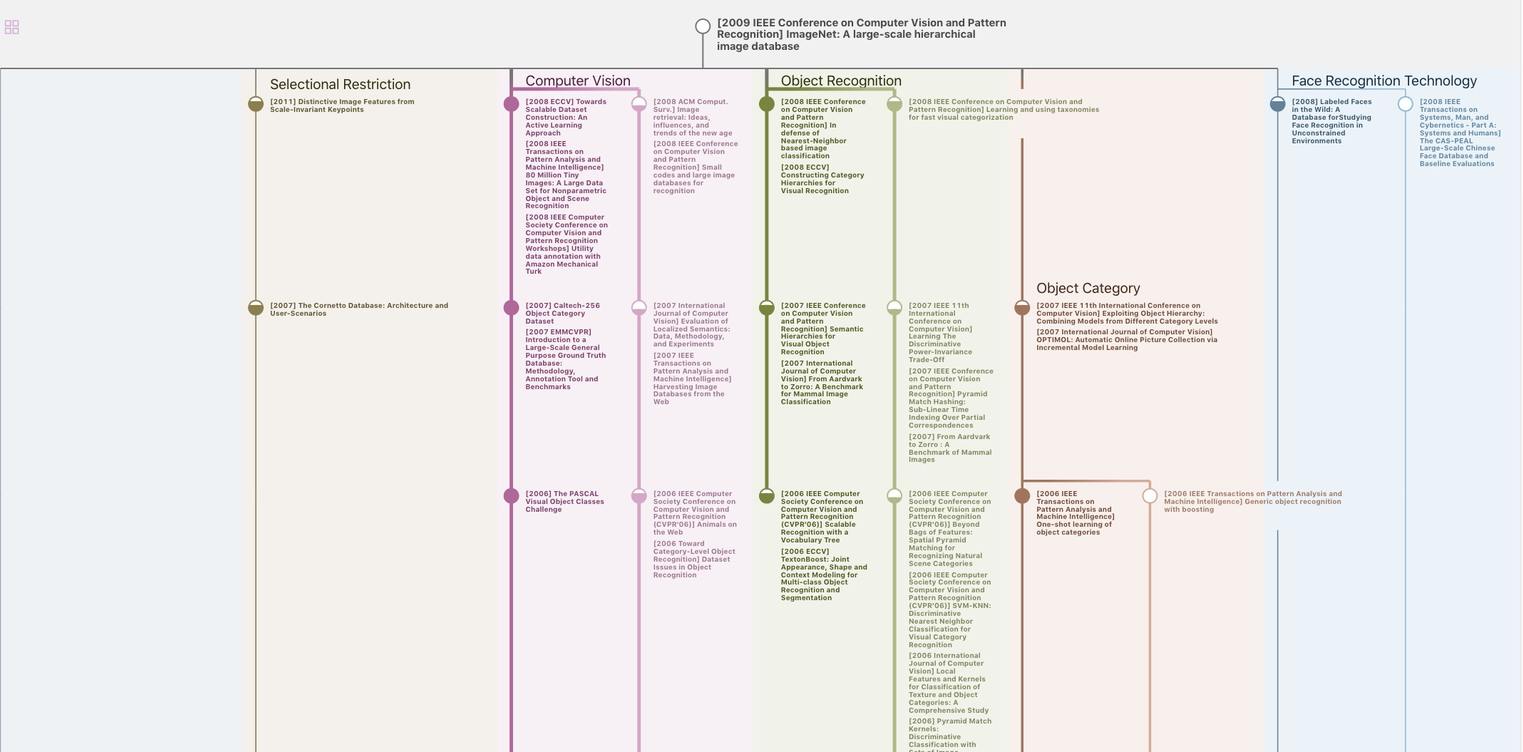
生成溯源树,研究论文发展脉络
Chat Paper
正在生成论文摘要