Towards a Multi-View Attentive Matching for Personalized Expert Finding
International World Wide Web Conference(2022)
摘要
ABSTRACT In Community Question Answering (CQA) websites, expert finding aims at seeking suitable experts to answer questions. The key is to explore the inherent relevance based on the representations of questions and experts. Existing methods usually learn these features from single view information (e.g., question title), which would be not insufficient to fully learn their representations. In this paper, we propose a personalized expert finding method with a multi-view attentive matching mechanism. We design three modules under the multi-view paradigm, including a question encoder, an intra-view encoder, and an inter-view encoder, which aims to comprehend the comprehensive relationships between experts and questions. In the question encoder, we learn the multi-view question features from its title, body and tag views respectively. In the intra-view encoder, we design an interactive attention network to capture the view-specific relevance between the target question and the historical answered questions of experts for all different views. Furthermore, in the inter-view encoder we employ a personalized attention network to aggregate different view information to learn expert/question representations. In this way, the match of the expert and question could be fully captured from the multi-view information via the intra- and inter-view mechanisms. Experimental results on six datasets demonstrate that the proposed method could achieve better performance than existing state-of-the-art methods.
更多查看译文
关键词
Expert Finding, Community Question Answering, Multi-view, Personalized, Matching
AI 理解论文
溯源树
样例
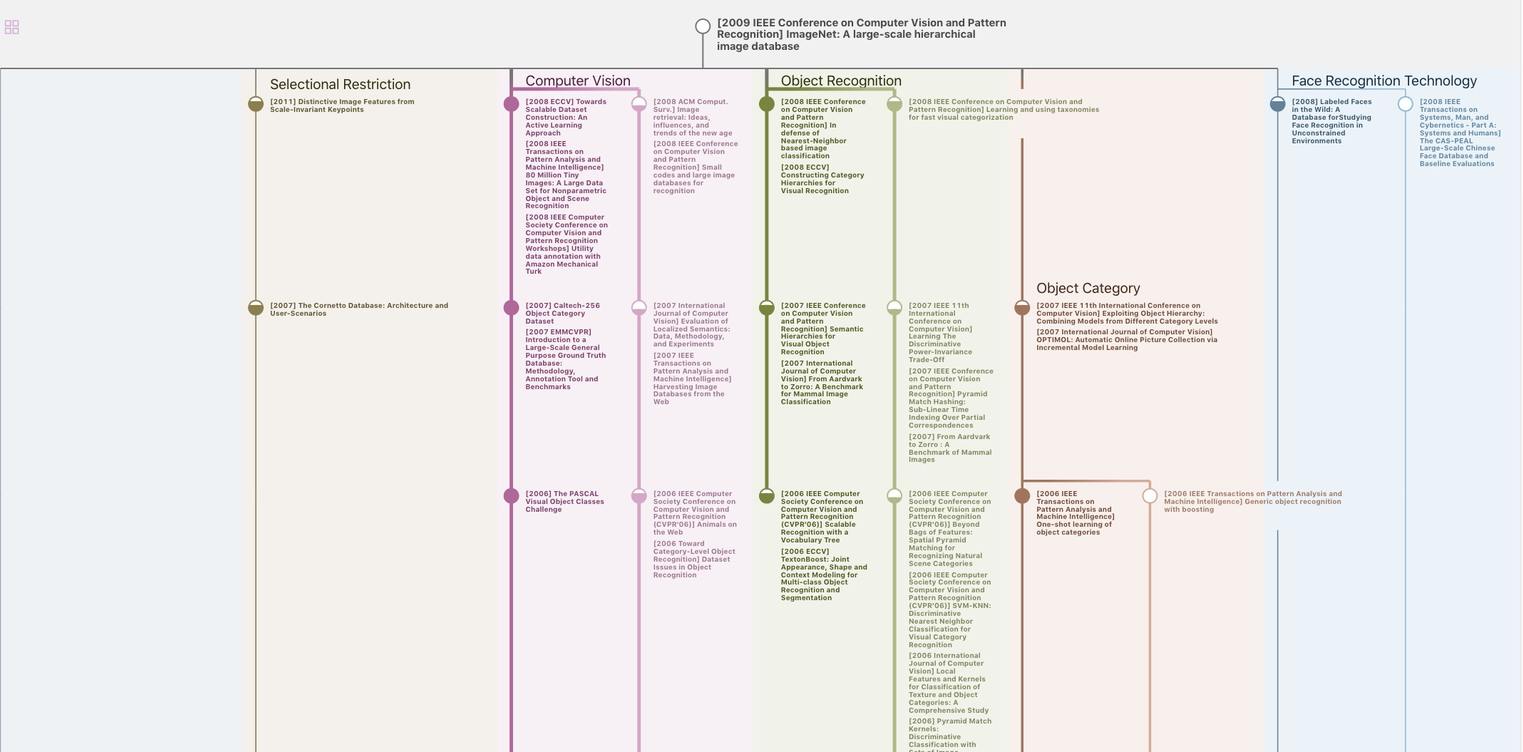
生成溯源树,研究论文发展脉络
Chat Paper
正在生成论文摘要