Robust System Instance Clustering for Large-Scale Web Services
International World Wide Web Conference(2022)
摘要
ABSTRACTSystem instance clustering is crucial for large-scale Web services because it can significantly reduce the training overhead of anomaly detection methods. However, the vast number of system instances with massive time points, redundant metrics, and noise bring significant challenges. We propose OmniCluster to accurately and efficiently cluster system instances for large-scale Web services. It combines a one-dimensional convolutional autoencoder (1D-CAE), which extracts the main features of system instances, with a simple, novel, yet effective three-step feature selection strategy. We evaluated OmniCluster using real-world data collected from a top-tier content service provider providing services for one billion+ monthly active users (MAU), proving that OmniCluster achieves high accuracy (NMI=0.9160) and reduces the training overhead of five anomaly detection models by 95.01% on average.
更多查看译文
关键词
Multivariate time series, Clustering, 1D-CAE
AI 理解论文
溯源树
样例
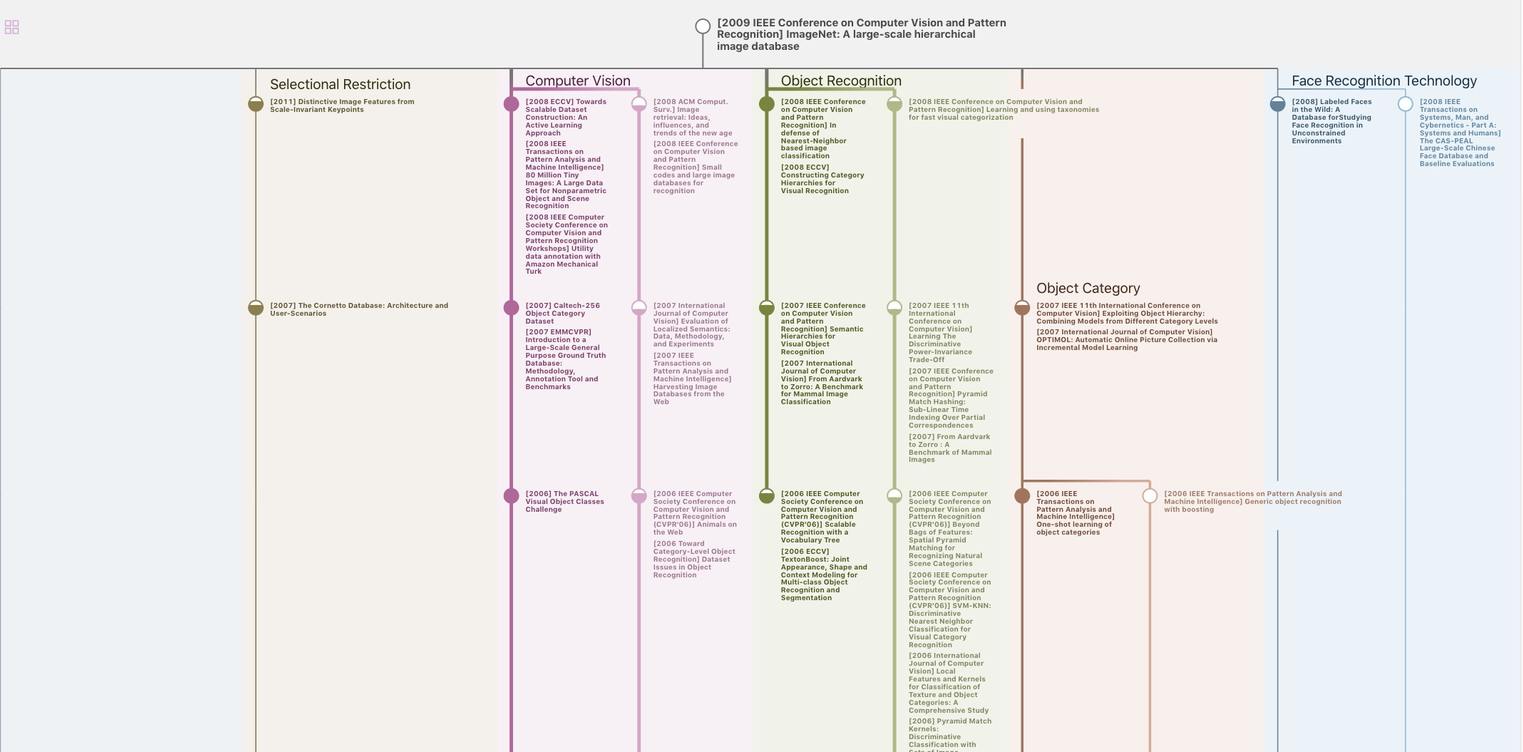
生成溯源树,研究论文发展脉络
Chat Paper
正在生成论文摘要