A framework for estimating actual evapotranspiration at weather stations without flux observations by combining data from MODIS and flux towers through a machine learning approach
Journal of Hydrology(2021)
摘要
•Machine learning provides a feasible framework for estimating actual evapotranspiration at weather stations without flux observations.•Seasonal ET simulations performed better than that of daily, 8-day and monthly using pooled data from all flux towers.•The predictors with high contribution to the model are Tmax, Tmin, Tmean, Prcp, SWI, Rn, NDVI, LAI, FPAR, SWC and Tsoil.•Changes in the importance ranking and value of predictor variables partly explained the variations of model performance.
更多查看译文
关键词
Evapotranspiration,Weather stations without flux observations,Multiple temporal resolutions,Spatial cross-validation,Machine learning,Grasslands
AI 理解论文
溯源树
样例
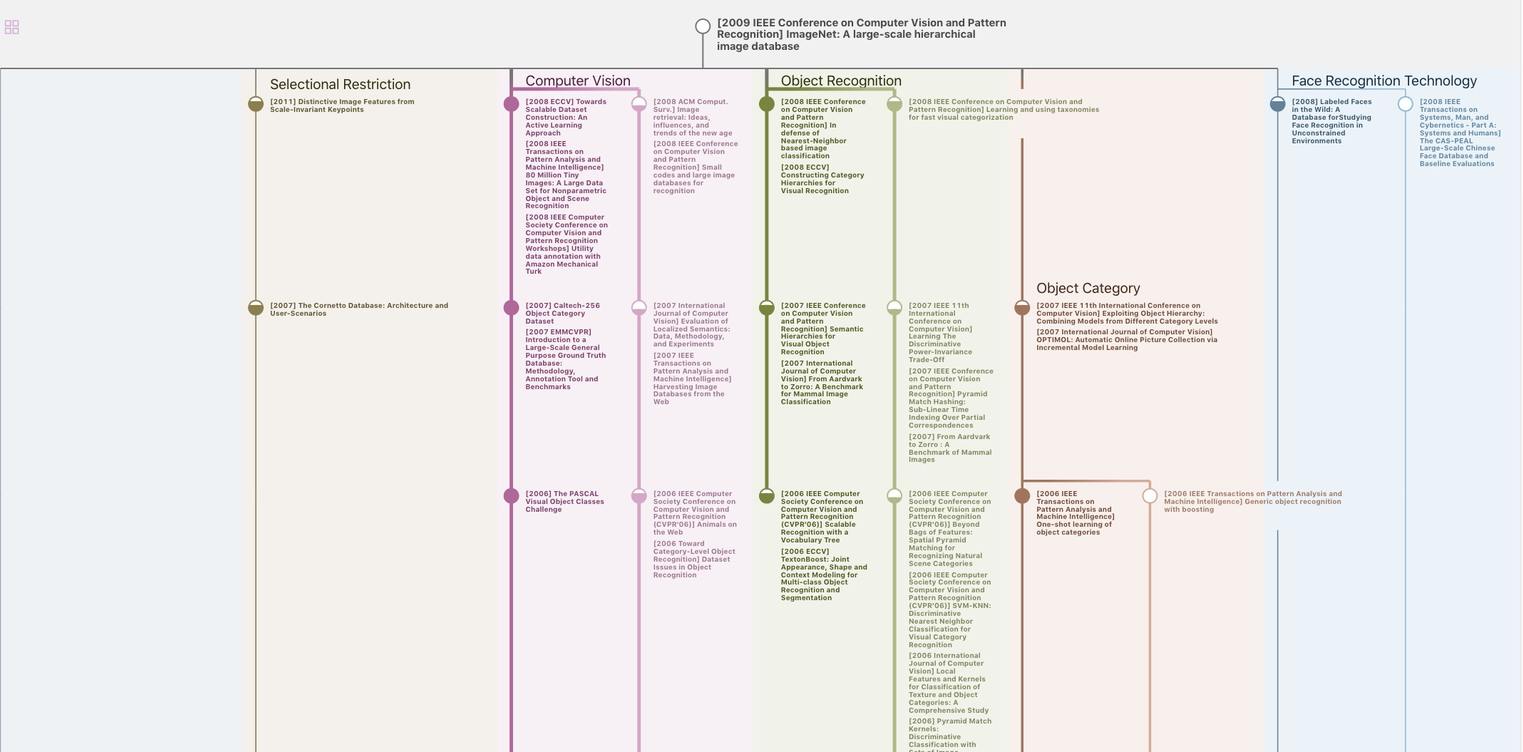
生成溯源树,研究论文发展脉络
Chat Paper
正在生成论文摘要