Mineral prospecting from biogeochemical and geological information using hyperspectral remote sensing - Feasibility and challenges
Journal of Geochemical Exploration(2022)
摘要
Biogeochemistry is an effective and fast approach for orientation studies in mineral exploration. Vegetative cover has been used in a range of geological environments to gain insight into the composition of sub-surface soils and bedrock found at depth; however, there is little to no records of biogeochemistry being coupled within a remote sensing and spectroscopy framework in the domain of mineral exploration. The study uses lab- and airborne hyperspectral remote sensing to localize gold mineralisation using the concept of biogeochemistry. This pilot study uses plant species, Pinus radiata in close proximity to a mineralised shear zone. This study used a 2 m spatial resolution airborne hyperspectral data, captured by AisaFENIX sensor and was complimented with lab-based hyperspectral data of the sampled vegetation and soil/rock exposed along the Hyde-Macraes Shear Zone, within the Otago schist belt in the South Island, New Zealand. The hyperspectral data, and the biogeochemistry data was explored using several statistical approaches to determine significant relationships between the underlying soil and rock substrate and biogeochemistry of the sampled vegetation with a focus on pathfinder elements to gold-mineralisation. Three commonly used multivariate predictive regression models, were tested, including partial least squares regression (PLSR), kernel based PLSR (kPLSR) and random forest regression (RFR) modelling. The results suggest that hyperspectral scanning can discriminate samples from a mineralised zone spectrally. Furthermore, the lab-based hyperspectral data outcompetes the airborne hyperspectral data for vegetation to identify areas of potential mineralisation. The model performances vary depending on the part of the plant analysed, and bark samples are found to be promising to explore underlying geological resources in the future. The regression model that show up to R2cv > 0.7 using bark samples decreases to R2cv ~ 0.2 when trained with needle samples. The airborne data is significantly more complex, which is observed in poorer model performances with R2cv ranging from 0.2 to 0.5.
更多查看译文
关键词
Mineral exploration,Shear zone,Biogeochemistry,Statistical modelling,Hyperspectral remote sensing,Spectroscopy
AI 理解论文
溯源树
样例
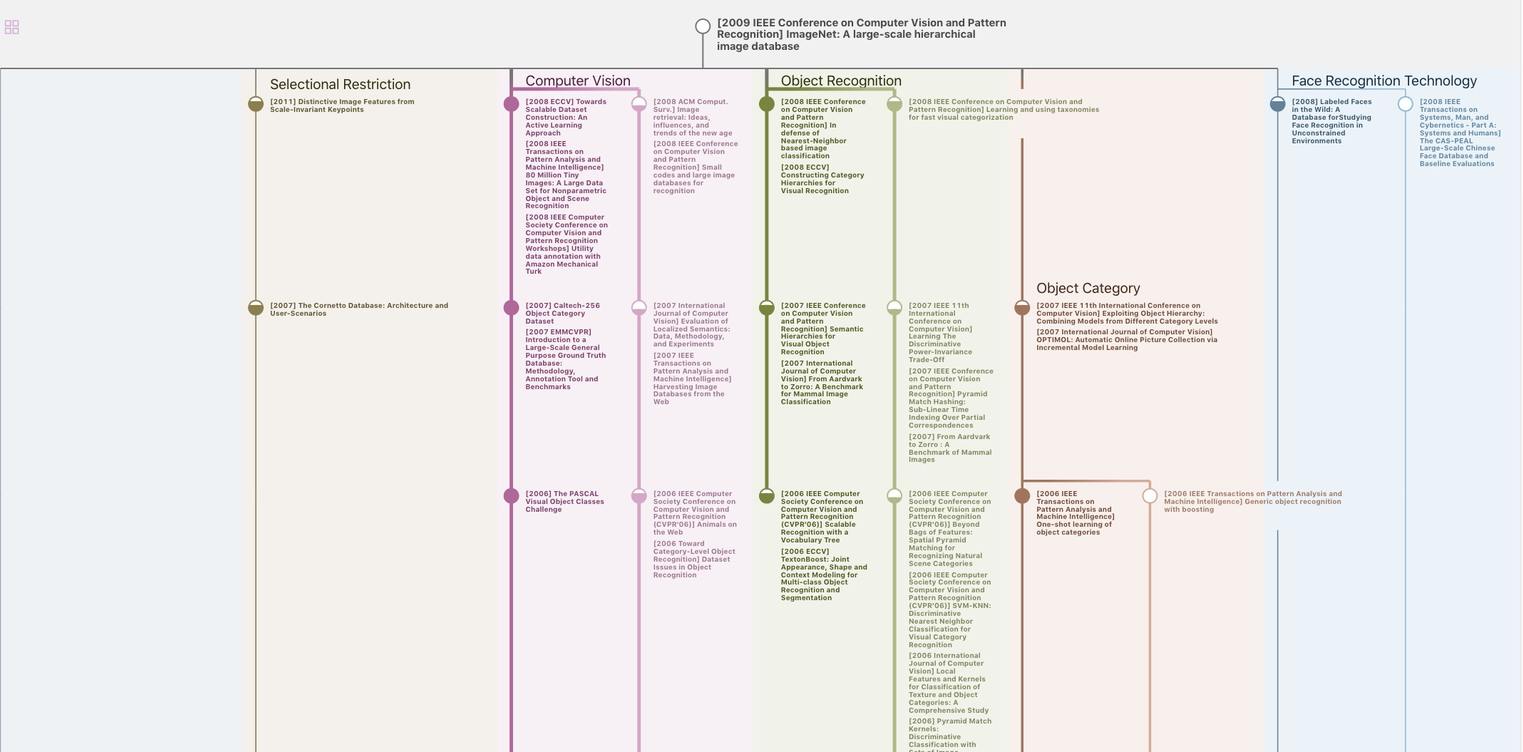
生成溯源树,研究论文发展脉络
Chat Paper
正在生成论文摘要